PrimitivePose: 3D Bounding Box Prediction of Unseen Objects via Synthetic Geometric Primitives
2022 Sixth IEEE International Conference on Robotic Computing (IRC)(2022)
摘要
This paper studies the challenging problem of 3D pose and size estimation for multi-object scene configurations from stereo views. Most existing methods rely on CAD models and are therefore limited to a predefined set of known object categories. This closed-set constraint limits the range of applications for robots interacting in dynamic environments where previously unseen objects may appear. To address this problem we propose an oriented 3D bounding box detection method that does not require 3D models or semantic information of the objects and is learned entirely from the category-specific domain, relying on purely geometric cues. These geometric cues are objectness and compactness, as represented in the synthetic domain by generating a diverse set of stereo image pairs featuring pose annotated geometric primitives. We then use stereo matching and derive three representations for 3D image content: disparity maps, surface normal images and a novel representation of disparity-scaled surface normal images. The proposed model, PrimitivePose, is trained as a single-stage multi-task neural network using any one of those representations as input and 3D oriented bounding boxes, object centroids and object sizes as output. We evaluate PrimitivePose for 3D bounding box prediction on difficult unseen objects in a tabletop environment and compare it to the popular PoseCNN model-a video showcasing our results can be found at: https://preview.tinyurl.com/2pccumvt.
更多查看译文
关键词
3D bounding box prediction,unseen objects,synthetic data,geometric primitives,object pose annotation
AI 理解论文
溯源树
样例
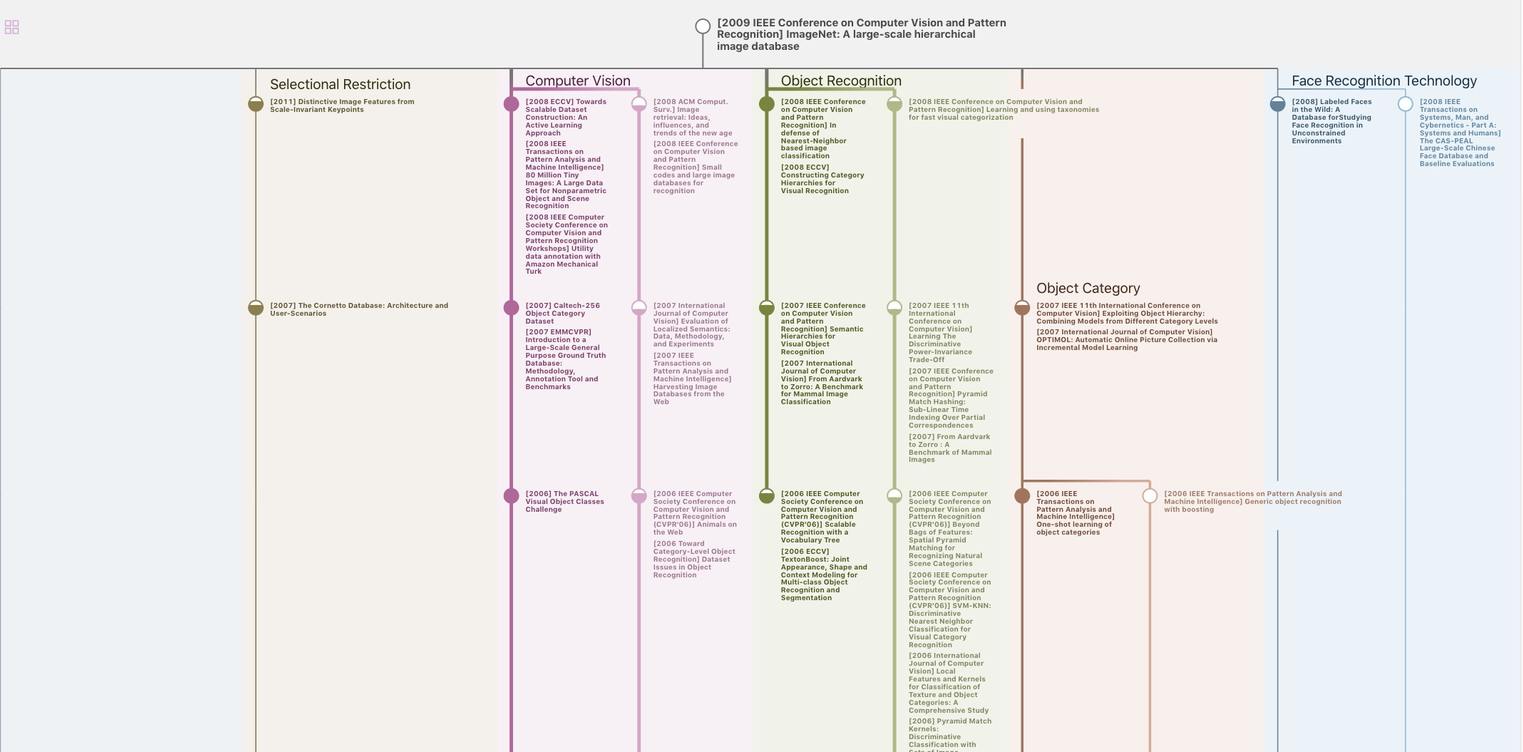
生成溯源树,研究论文发展脉络
Chat Paper
正在生成论文摘要