Evaluation of Deep Learning Context-Sensitive Visualization Models
2022 26th International Conference Information Visualisation (IV)(2022)
摘要
The introduction of Transformer neural networks has changed the landscape of Natural Language Processing (NLP) during the recent years. These models are very complex, and therefore hard to debug and explain. In this context, visual explanation became an attractive approach. The visualization of the path that leads to certain outputs of a model is at the core of visual explanation, as this illuminates the features or parts of the model that may need to be changed to achieve the desired results. In particular, one goal of a NLP visual explanation is to highlight the most significant parts of the text that have the greatest impact on the model output. Several visual explanation methods for NLP models were recently proposed. A major challenge is how to compare the performances of such methods since we cannot simply use the usual classification accuracy measures to evaluate the quality of visualizations. We need good metrics and rigorous criteria to measure how useful the extracted knowledge is for explaining the models. In addition, we want to visualize the differences between the knowledge extracted by different models, in order to be able to rank them. In this paper, we investigate how to evaluate explanations/visualizations resulted from machine learning models for text classification. The goal is not to improve the accuracy of a particular NLP classifier, but to assess the quality of the visualizations that explain its decisions. We describe several methods for evaluating the quality of NLP visualizations, including both automated techniques based on quantifiable measures and subjective techniques based on human judgements.
更多查看译文
关键词
Natural Language Processing,Transformers,BERT,Visualization of Neural Networks
AI 理解论文
溯源树
样例
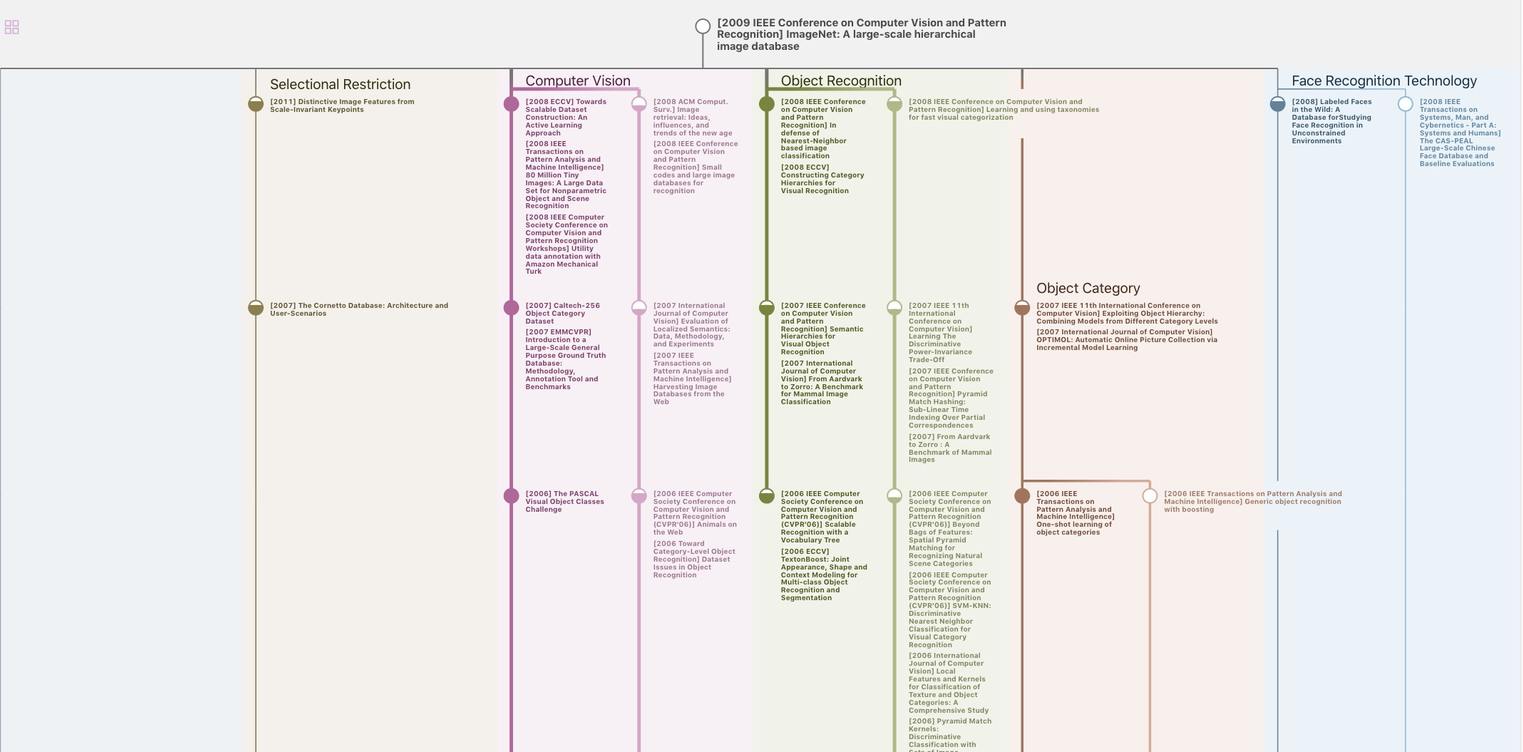
生成溯源树,研究论文发展脉络
Chat Paper
正在生成论文摘要