Deep Reinforcement Learning Based Computation Offloading and Trajectory Planning for Multi-UAV Cooperative Target Search
IEEE Journal on Selected Areas in Communications(2023)
Abstract
Unmanned aerial vehicles (UAVs) are widely used for surveillance and monitoring to complete target search tasks. However, the short battery life and moderate computational capability hinder UAVs to process computation-intensive tasks. The emerging edge computing technologies can alleviate this problem by offloading tasks to the ground edge servers. How to evaluate the search process so as to make optimal offloading decisions and make optimal flying trajectories represent fundamental research challenges. In this paper, we propose to utilize the concept of uncertainty to evaluate the search process, which reflects the reliability of the target search results. Thereafter, we propose a deep reinforcement learning (DRL) technique to jointly make optimal computation offloading decisions and flying orientation choices for multi-UAV cooperative target search. Specifically, we first formulate an uncertainty minimization problem based on the established system model. By introducing a reward function, we prove that the uncertainty minimization problem is equivalent to a reward maximization problem, which is further analyzed by a Markov decision process (MDP). To obtain the optimal task offloading decisions and flying orientation choices, a deep Q-network (DQN) based DRL architecture with a separated Q-network is then proposed. Finally, extensive simulations validate the effectiveness of the proposed techniques, and comprehensive discussions on how different parameters affect the search performance are given.
MoreTranslated text
Key words
Unmanned aerial vehicle,cooperative target search,edge computing,computation offloading,deep reinforcement learning (DRL)
AI Read Science
Must-Reading Tree
Example
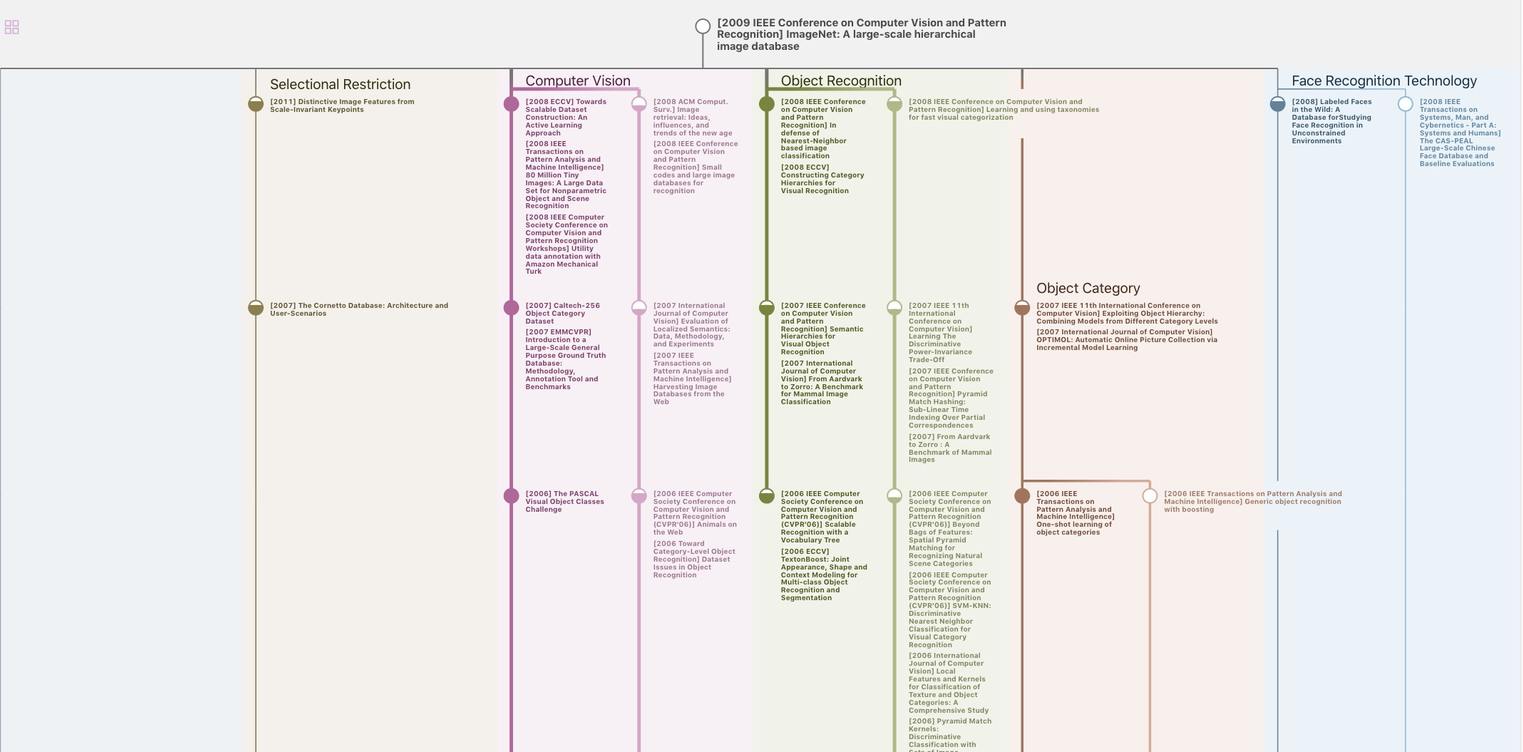
Generate MRT to find the research sequence of this paper
Chat Paper
Summary is being generated by the instructions you defined