Solar Power Generation Prediction Using Machine Learning and Study of Power Generation Using Solar Tracking Panels
Transactions of the Korean Society of Mechanical Engineers - B(2023)
摘要
As the importance of renewable energy is growing worldwide, Republic of Korea aims to increase the proportion of renewable energy generation to 30.2% by 2030. Solar power plays an important role, accounting for more than 80% of domestic renewable energy generation. However, since the amount of solar power generation varies greatly depending on weather factors, it is necessary to respond to the instability of solar power generation and increase the generation amount in order to secure stable energy. Therefore, in this paper, we learn a machine learning model based on weather data to predict the amount of solar power generation, calculate the solar altitude and azimuth that change according to seasons and time, and increase the amount of solar power generation by controlling the solar power panel. As a result of the experiment, the power generation was predicted to be within 0.208 mean square error, and it was confirmed that the difference in power generation before and after sun tracking was 23.4%.
更多查看译文
关键词
Machine Learning, Recurrent Neural Network, Pearson Correlation, Ensemble, Photovoltaics, Field Experiments, Sun Tracking System, Computation of Sun Position
AI 理解论文
溯源树
样例
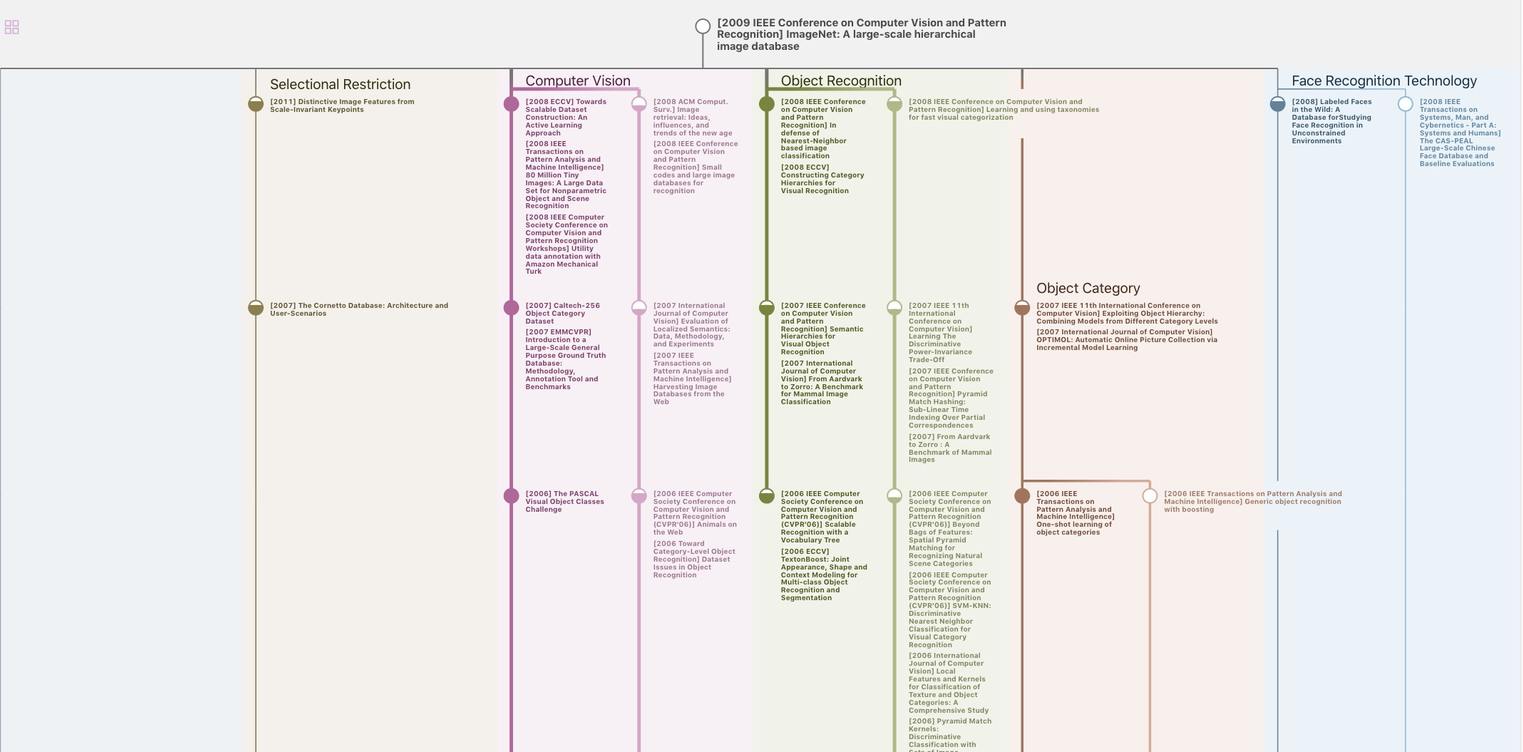
生成溯源树,研究论文发展脉络
Chat Paper
正在生成论文摘要