ENDE-GNN: An Encoder-decoder GNN Framework for Sketch Semantic Segmentation
2022 IEEE International Conference on Visual Communications and Image Processing (VCIP)(2022)
摘要
Sketch semantic segmentation serves as an important part of sketch interpretation. Recently, some researchers have obtained significant results using graph neural networks (GNN) for this task. However, existing GNN-based methods usually neglect the drawing order of sketches thus missing out the sequence information inherent to sketches. Towards solving this problem to achieve better performance on sketch semantic segmentation, we propose an encoder-decoder GNN framework named ENDE-GNN. Working with an auxiliary decoder, our ENDE-GNN guides the GNN backbone network to not only extract the inter-stroke and intra-stroke features, but also pays attention to the drawing order of sketches. This decoder acts during training only, preventing any additional overhead during testing. The proposed ENDE-GNN obtains state-of-the-art per-formances on three public sketch semantic segmentation datasets, namely SPG, SketchSeg-150K, and CreativeSketch. We further evaluate the effectiveness of ENDE-GNN via ablation studies and visualizations. Codes are available at https://github.com/PRIS-CV/ENDE_For_SSS.
更多查看译文
关键词
Sketch Semantic Segmentation,Graph Neural Network,Encoder-decoder Framework
AI 理解论文
溯源树
样例
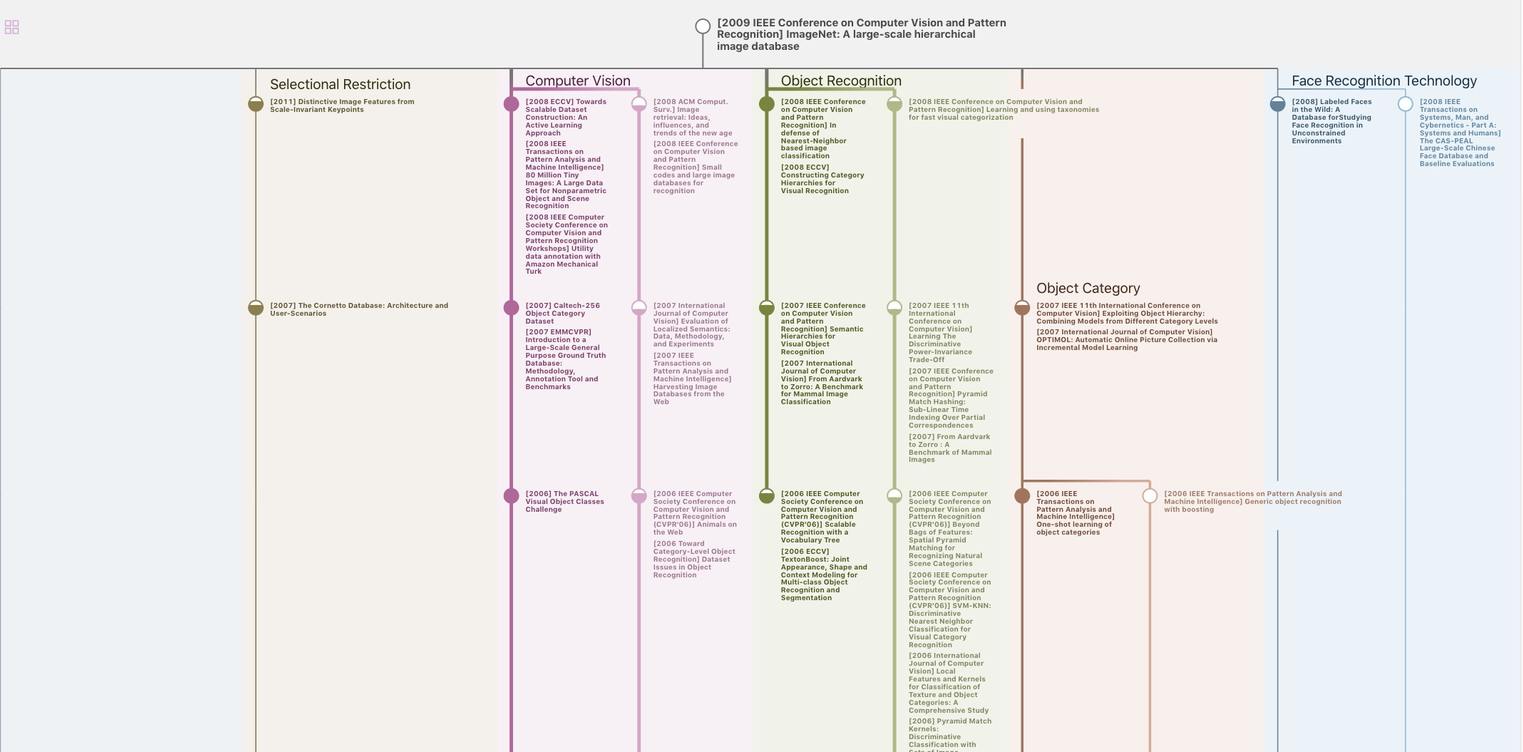
生成溯源树,研究论文发展脉络
Chat Paper
正在生成论文摘要