Hybrid Attention-Based Encoder-Decoder Fully Convolutional Network for PolSAR Image Classification
Remote. Sens.(2023)
摘要
Recently, methods based on convolutional neural networks (CNNs) achieve superior performance in polarimetric synthetic aperture radar (PolSAR) image classification. However, the current CNN-based classifiers follow patch-based frameworks, which need input images to be divided into overlapping patches. Consequently, these classification approaches have the drawback of requiring repeated calculations and only relying on local information. In addition, the receptive field size in conventional CNN-based methods is fixed, which limits the potential to extract features. In this paper, a hybrid attention-based encoder-decoder fully convolutional network (HA-EDNet) is presented for PolSAR classification. Unlike traditional CNN-based approaches, the encoder-decoder fully convolutional network (EDNet) can use an arbitrary-size image as input without dividing. Then, the output is the whole image classification result. Meanwhile, the self-attention module is used to establish global spatial dependence and extract context characteristics, which can improve the performance of classification. Moreover, an attention-based selective kernel module (SK module) is included in the network. In the module, softmax attention is employed to fuse several branches with different receptive field sizes. Consequently, the module can capture features with different scales and further boost classification accuracy. The experiment results demonstrate that the HA-EDNet achieves superior performance compared to CNN-based and traditional fully convolutional network methods.
更多查看译文
关键词
polarimetric synthetic aperture radar (PolSAR),image classification,fully convolutional neural network (FCN),self-attention,receptive field
AI 理解论文
溯源树
样例
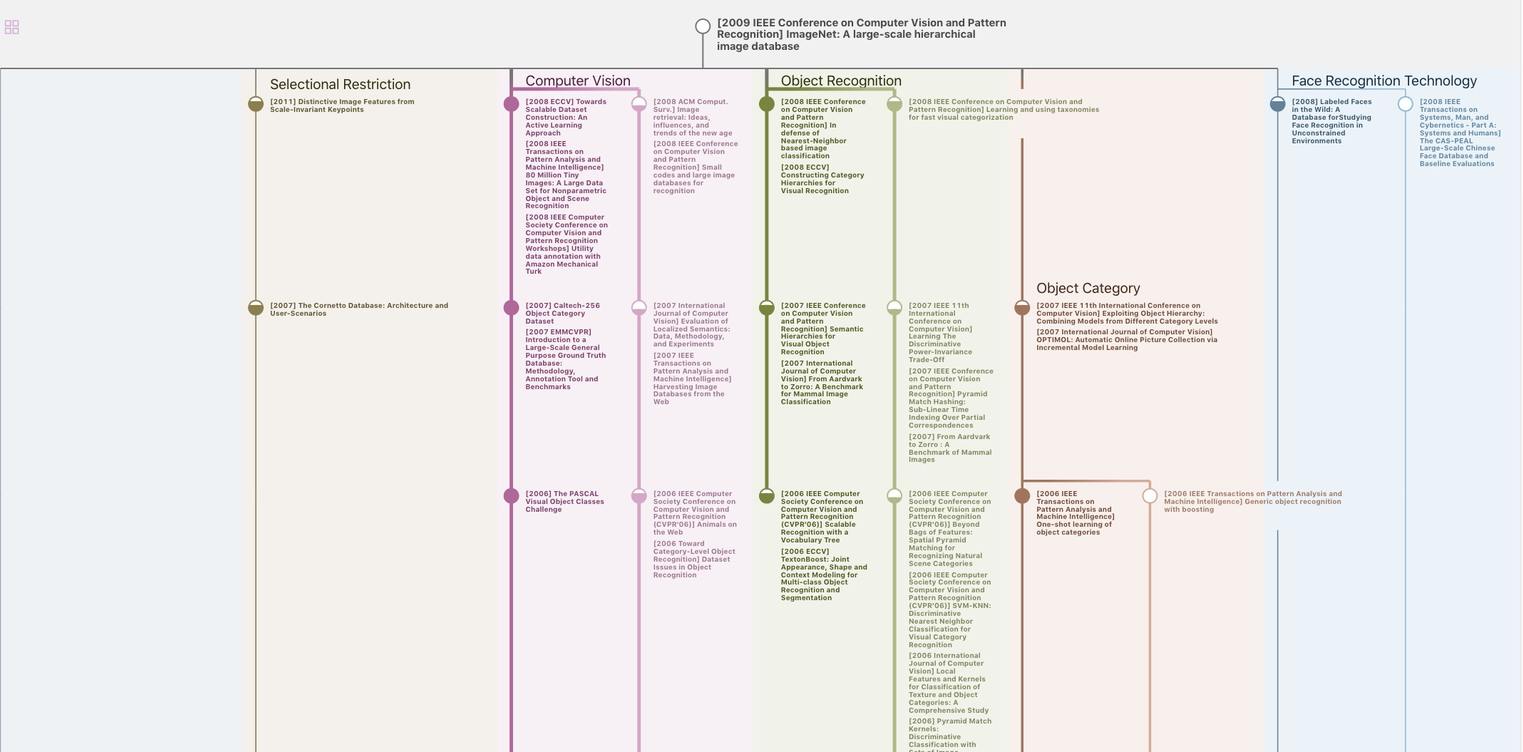
生成溯源树,研究论文发展脉络
Chat Paper
正在生成论文摘要