Generating accurate negative samples for landslide susceptibility mapping: A combined self-organizing-map and one-class SVM method
Frontiers in Earth Science(2023)
摘要
The accuracy of data-driven landslide susceptibility mapping (LSM) is closely affected by the quality of non-landslide samples. This research proposes a method combining a self-organizing-map (SOM) and a one-class SVM (SOM-OCSVM) to generate more reasonable non-landslide samples. We designed two steps: first, a random selection, a SOM network, a one class SVM model, and a SOM-OCSVM model were used to generate non-landslide sample datasets. Second, four machine learning models (MLs)-namely logistic regression (LRG), multilayer perceptron (MLP), support vector machine (SVM), and random forest (RF)-were used to verify the effects of four non-landslide sample datasets on LSM. From the region along the Sichuan-Tibet Highway, we selected 11 conditioning factors and 1186 investigated landslides to perform the aforementioned experiments. The results show that the SOM-OCSVM method achieves the highest AUC (> 0.94) and minimum standard deviation (< 0.081) compared with other methods. Moreover, RF achieves the best performance in different datasets compared with other ML models. The aforementioned results prove that the proposed method can enhance the performance of ML models to produce more reliable LSM.
更多查看译文
关键词
landslide susceptibility mapping,self-organizing map,one-class SVM,the Sichuan-Tibet highway,machine learning
AI 理解论文
溯源树
样例
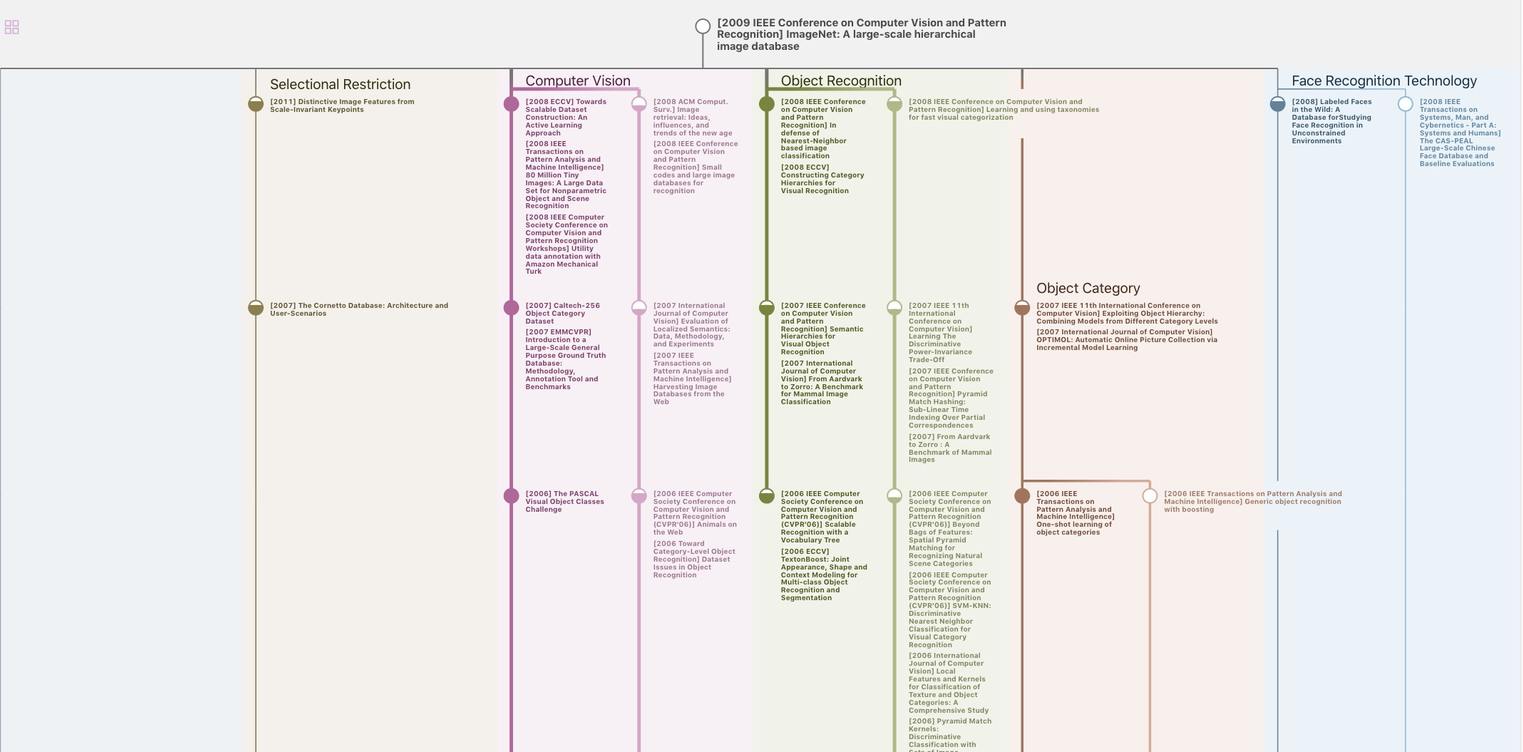
生成溯源树,研究论文发展脉络
Chat Paper
正在生成论文摘要