Fine Tuning an AraT5 Transformer for Arabic Abstractive Summarization
2022 14th International Conference on Computational Intelligence and Communication Networks (CICN)(2022)
摘要
Creating an abstractive summary of a document by rephrasing its most crucial sentences is a challenging but crucial task in natural language processing. The field witnessed a remarkable development with deep learning techniques, especially with the emergence of pre-trained models that achieved the best results by training them on very large data and trained later on specific tasks. In this paper, we used the T5 model, which achieved results that are considered the best in different tasks of natural language processing. AraT5 is the newly launched Arabic language version, as we have worked on fine-tuning it on a dataset of 267,000 Arabic articles. The model was evaluated through ROUGE-1, ROUGE-2, ROUGE-L, and BLEU and the results were 0.494 0.339 0.469 0.4224, respectively. In addition, the AraT5 model is superior to other state-of-the-art research studies using the sequence-to-sequence model.
更多查看译文
关键词
Natural language processing,Automatic Text Summarization,Abstractive Summarization,Arabic text Summarization,pre-trained model
AI 理解论文
溯源树
样例
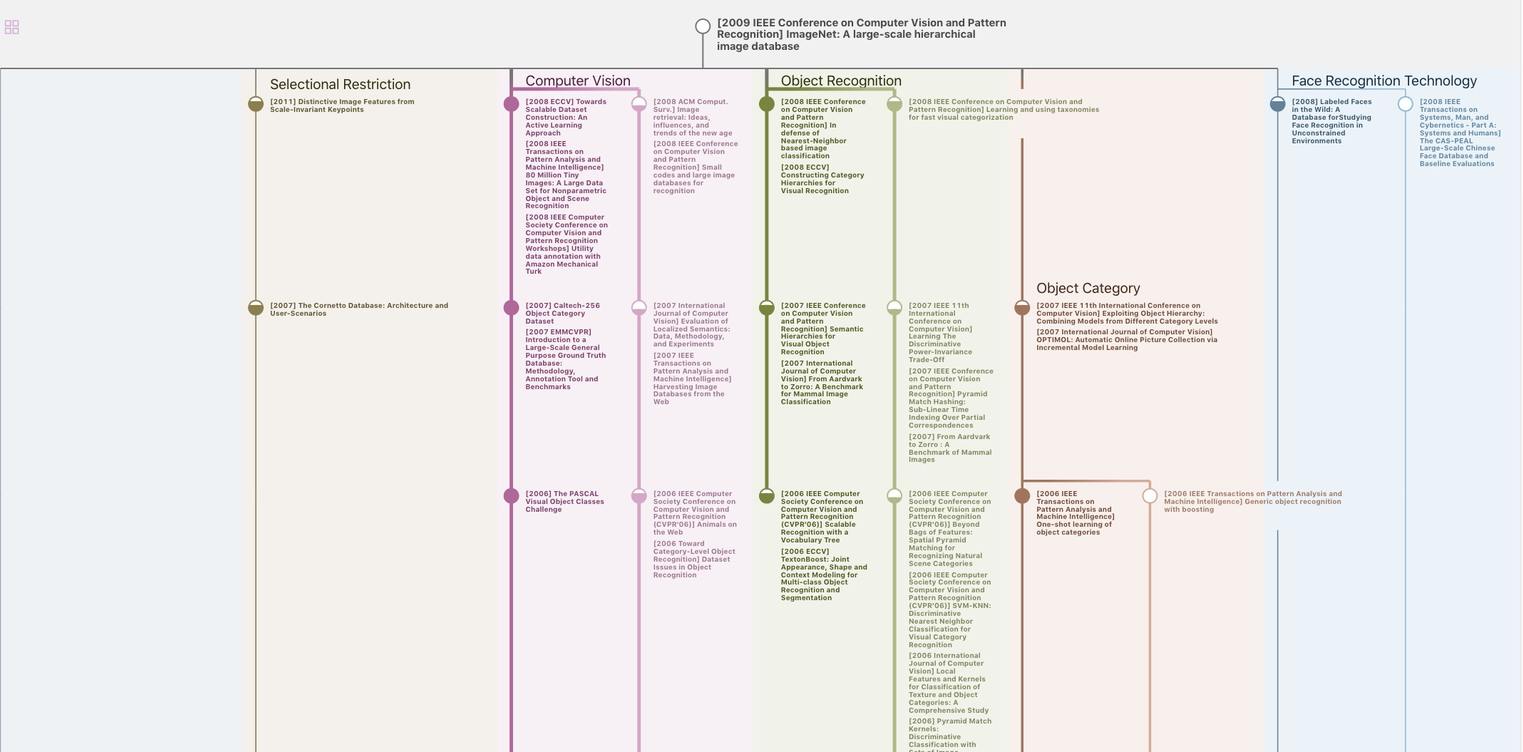
生成溯源树,研究论文发展脉络
Chat Paper
正在生成论文摘要