Physics-informed machine learning model for prediction of long-rod penetration depth in a semi-infinite target
International Journal of Impact Engineering(2023)
摘要
Traditional data-driven approaches to long-rod penetration modeling incorporate significant expert bias through handcrafted analytical formulas. In this work, we investigate machine learning as an alternative to build a more flexible, less biased model. Published data from approximately 900 experiments were used to train an artificial neural network to predict the depth of penetration into a semi-infinite target. On the basis of empirical parameter studies, we optimized the neural network architecture and regularization technique for this task. To aid the learning process, we incorporated into the machine learning model general physical principles, such as formalized in the Buckingham Pi theorem. The resulting physics-informed machine learning model shows good performance in generalization to new combinations of penetrator and target materials. As exemplary model application, we accurately postdict experimental results on the influence of target hardness fluctuations on penetration depth.
更多查看译文
关键词
Ballistic impact,Long rod penetration,Machine learning,Artificial neural network,Buckingham Pi theorem
AI 理解论文
溯源树
样例
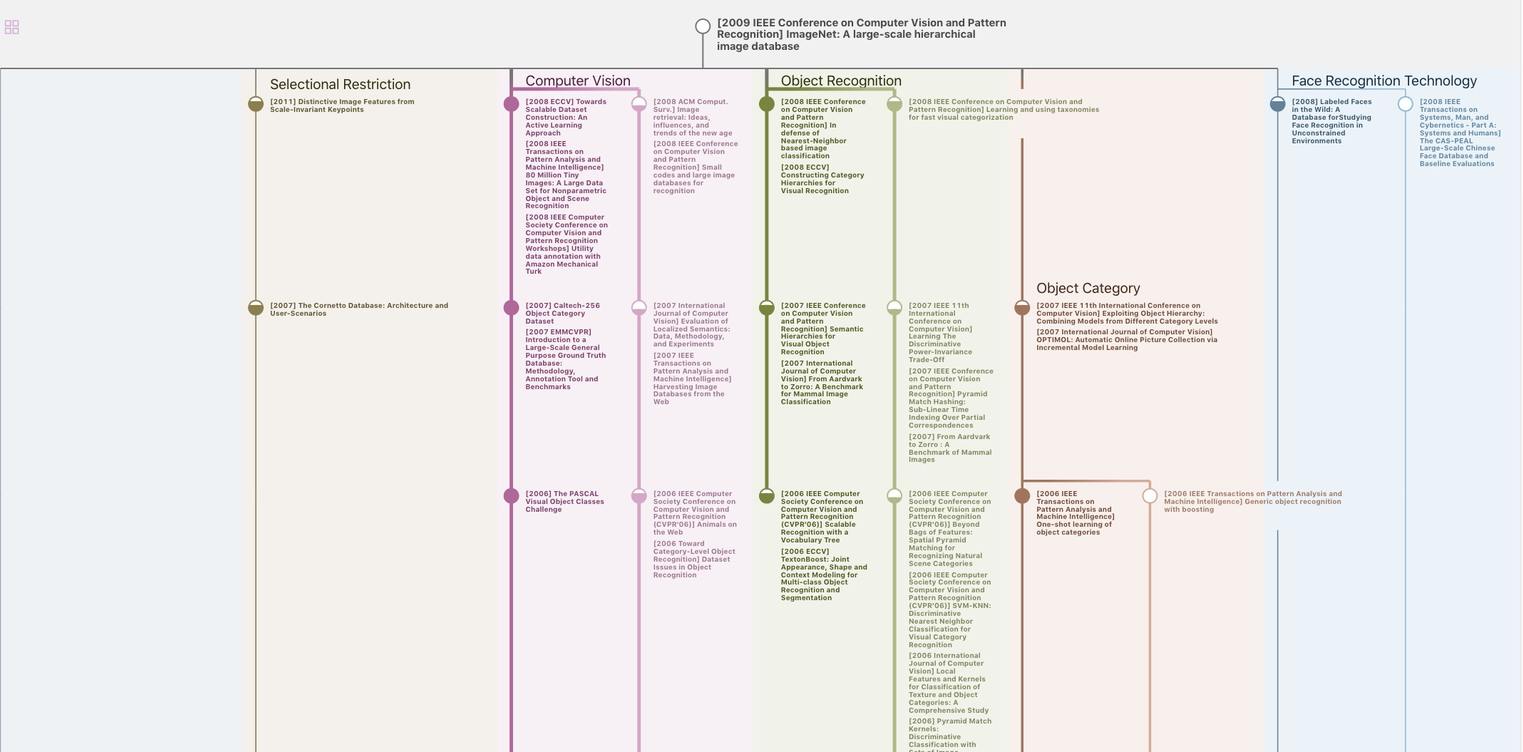
生成溯源树,研究论文发展脉络
Chat Paper
正在生成论文摘要