A Network Science perspective of Graph Convolutional Networks: A survey
arxiv(2023)
摘要
The mining and exploitation of graph structural information have been the focal points in the study of complex networks. Traditional structural measures in Network Science focus on the analysis and modelling of complex networks from the perspective of network structure, such as the centrality measures, the clustering coefficient, and motifs and graphlets, and they have become basic tools for studying and understanding graphs. In comparison, graph neural networks, especially graph convolutional networks (GCNs), are particularly effective at integrating node features into graph structures via neighbourhood aggregation and message passing, and have been shown to significantly improve the performances in a variety of learning tasks. These two classes of methods are, however, typically treated separately with limited references to each other. In this work, aiming to establish relationships between them, we provide a network science perspective of GCNs. Our novel taxonomy classifies GCNs from three structural information angles, i.e., the layer-wise message aggregation scope, the message content, and the overall learning scope. Moreover, as a prerequisite for reviewing GCNs via a network science perspective, we also summarise traditional structural measures and propose a new taxonomy for them. Finally and most importantly, we draw connections between traditional structural approaches and graph convolutional networks, and discuss potential directions for future research.
更多查看译文
关键词
Complex networks,network science,graph convolutional networks,deep learning
AI 理解论文
溯源树
样例
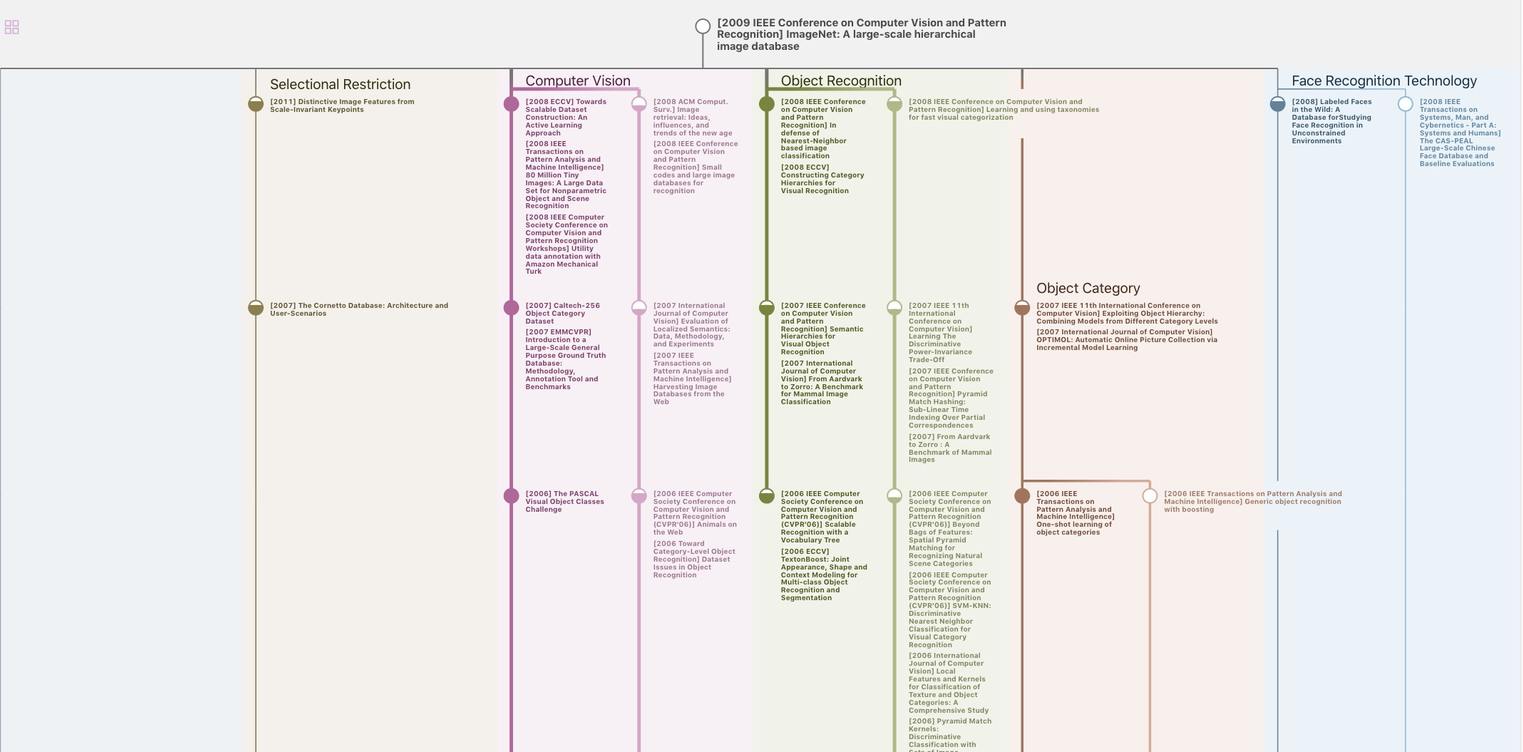
生成溯源树,研究论文发展脉络
Chat Paper
正在生成论文摘要