MG-CNFNet: A multiple grained channel normalized fusion networks for medical image deblurring
Biomedical Signal Processing and Control(2023)
摘要
In different medical imaging techniques, the relative displacement between the patient and detection instru-ment can lead to different degrees of motion blur, which will affect the diagnosis of the patient's condition. In this paper, we propose a deblurring model with Multiple Grained Channel Normalized Fusion Networks (MG-CNFNet), which decomposes the image input by multi-grained division to cascade feature extraction from fine to coarse, constructs the encoder using semi-channel self-normalization to enrich the multi-scale information of the image, highlight the main features and improve the deblurring performance, and uses a shallow convolutional fusion block to obtain more shallow information and balance the performance and efficiency of deblurring. Compared with other state-of-the-art methods, our method is qualitatively and quantitatively evaluated on three medical image datasets (including X-ray, Ultrasound, and MRI) to demonstrate its effectiveness in removing motion blur from medical images, and yields better restoration results.
更多查看译文
关键词
Multi grain,Semi-channel self-normalization,Shallow convolutional fusion,Deep neural network,Medical image deblurring
AI 理解论文
溯源树
样例
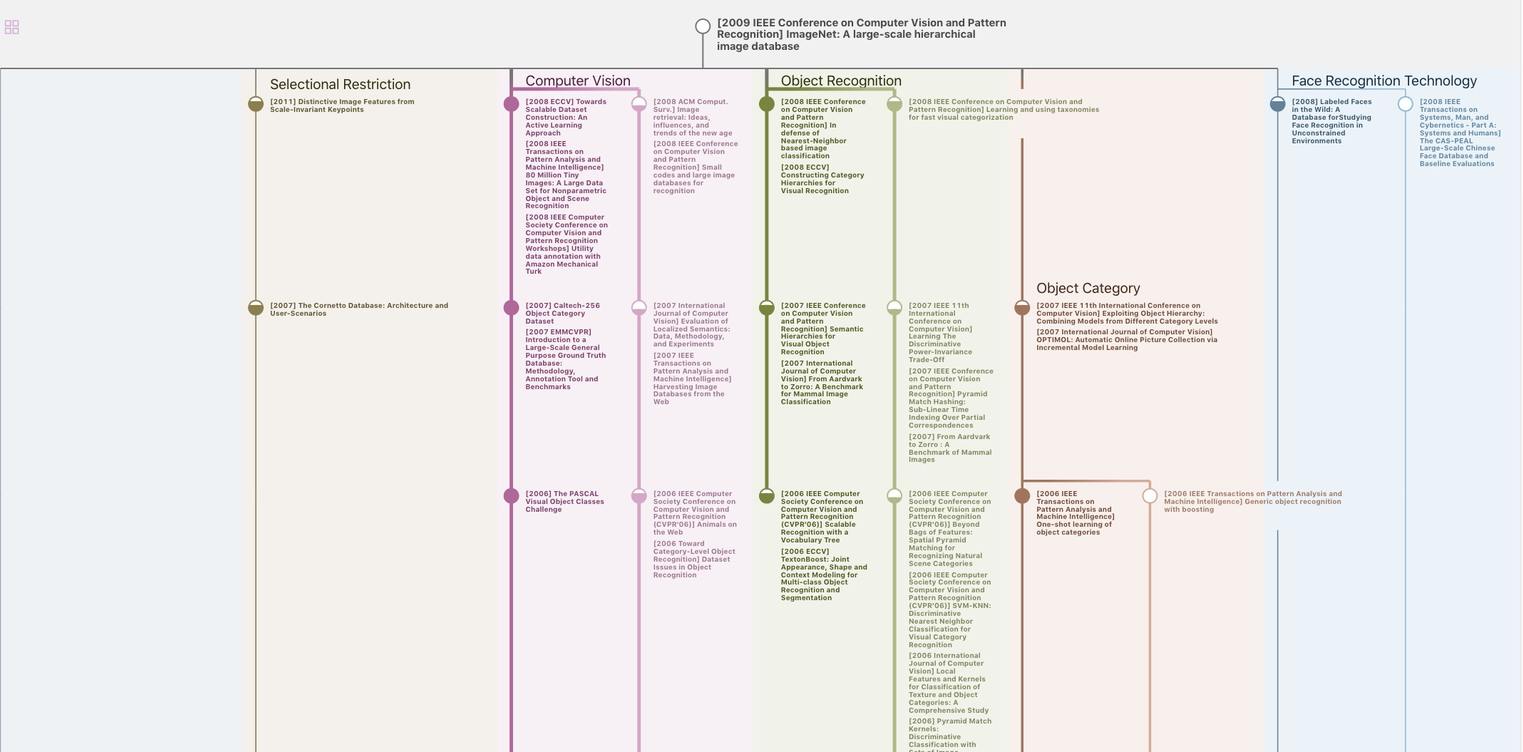
生成溯源树,研究论文发展脉络
Chat Paper
正在生成论文摘要