Improving brain tumor segmentation performance using CycleGAN based feature extraction
MULTIMEDIA TOOLS AND APPLICATIONS(2022)
摘要
The analysis of brain tumors plays a significant role in medical applications and provides a huge amount of anatomic and functional information. Automatic tumor segmentation is one of the most challenging issues among radiologists and other specialists intent on lowering and eliminating manual detection errors and speeding up the detection of tissue types. In recent years, automatic segmentation combined with deep learning has been proven to be more powerful than traditional approaches. This study introduces a two-step model for the segmentation of brain tumors on multi-channel MRI images based on the Generative Adversarial Network (GAN). First of all, we use CycleGAN and two segmentors added to its structure to train networks that produce new features appropriate for segmentation. Different modules of MRI images are fed into these two-way networks, and the same modules, along with the target tumor segment, are requested at the output. In addition to segmentation, features created in the middle layers of these networks are capable of mapping images to one another. In the second step, the transfer learning technique is used to extract the related subnets and inject the features produced into the main segmentation network. In fact, the present study attempts to find features with greater detection power by converting different MRI images to each other. It is assumed that features beneficial in the conversion of different MRI image modules to each other will also improve segmentation performance. The proposed method is evaluated on BraTS 2018, and the results demonstrate the superiority of this method over the majority of existing approaches.
更多查看译文
关键词
Generative adversarial networks, Transfer learning, CycleGAN, BraTs, Brain tumor segmentation
AI 理解论文
溯源树
样例
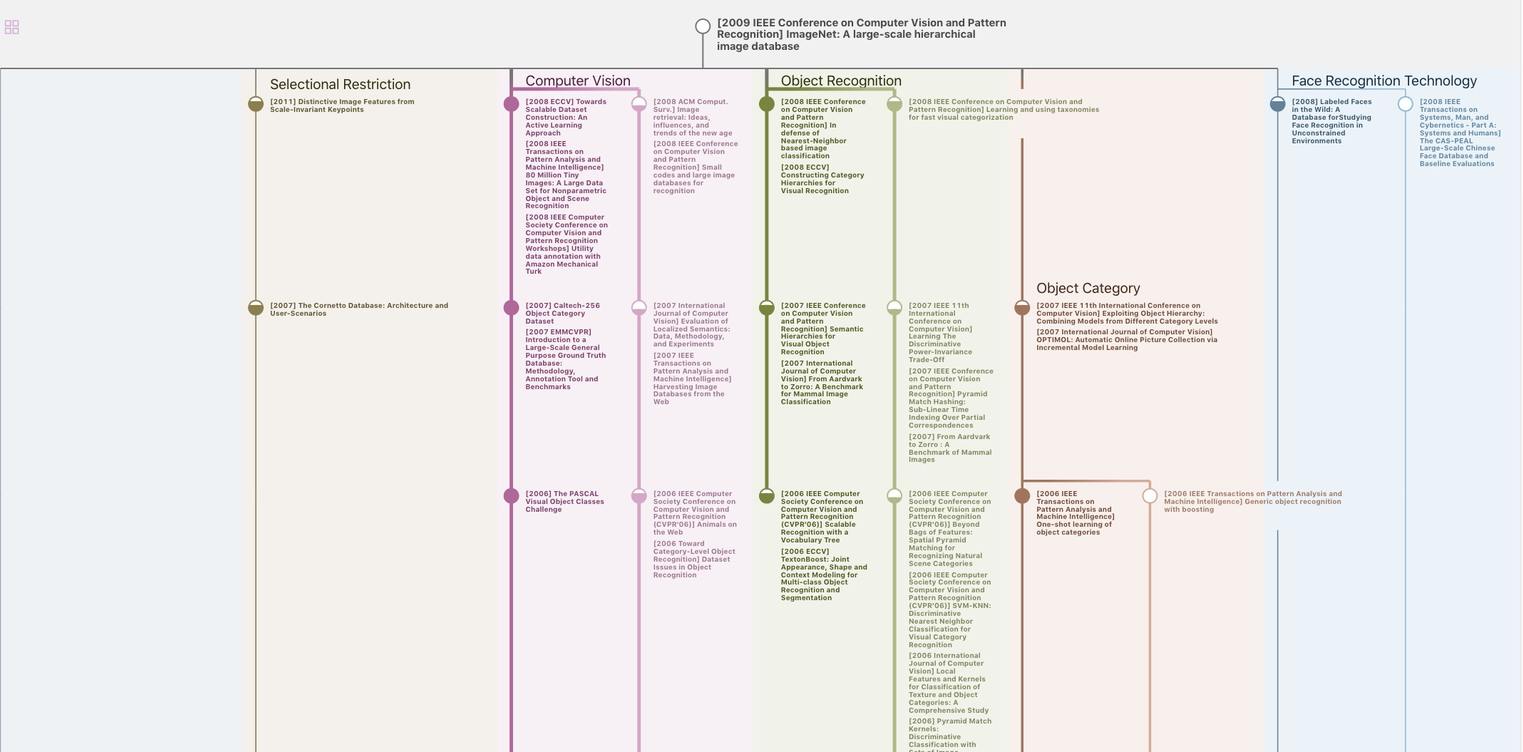
生成溯源树,研究论文发展脉络
Chat Paper
正在生成论文摘要