An Improved LST-KSVC Based on Energy Model
ENGINEERING LETTERS(2022)
摘要
Least squares twin support vector classification for K-class (LST-KSVC) [1] is an efficient multiclass classifier that incorporates least squares strategy into twin support vector classification for K-class (Twin-KSVC) [2]. Because of its excellent classification performance, LST-KSVC has been applied in many fields. However, the LST-KSVC has some drawbacks: (1) It only implements empirical risk minimization (ERM), which reduces its generalization performance. (2) It is sensitive to noise and outliers. (3) The inverse matrices need to be calculated, which is impossible for many large-scale engineering problems. (4) For the nonlinear case, the LST-KSVC needs to reconstruct primal problems using the approximate kernel-generated surface (AKGS) and does not directly use kernel tricks as in the support vector machine (SVM) [3]. To address these shortcomings, an improved LST-KSVC based on energy model, which is called ELST-KSVC, is proposed in this paper. First, a regularization term is introduced into LST-KSVC to implement structural risk minimization (SRM). Second, energy parameters are introduced into LST-KSVC to reduce the effect of noise and outliers. Third, the dual problems are reconstructed to avoid inverse matrices. Furthermore, the sequential minimal optimization (SMO) algorithm is used to efficiently train subclassifiers. Finally, ELST-KSVC can directly use kernel tricks for nonlinear cases. Experimental results show that the ELST-KSVC has better generalization performance and higher learning speed.
更多查看译文
关键词
multiclass classification,LST-KSVC,Twin-KSVC,SMO
AI 理解论文
溯源树
样例
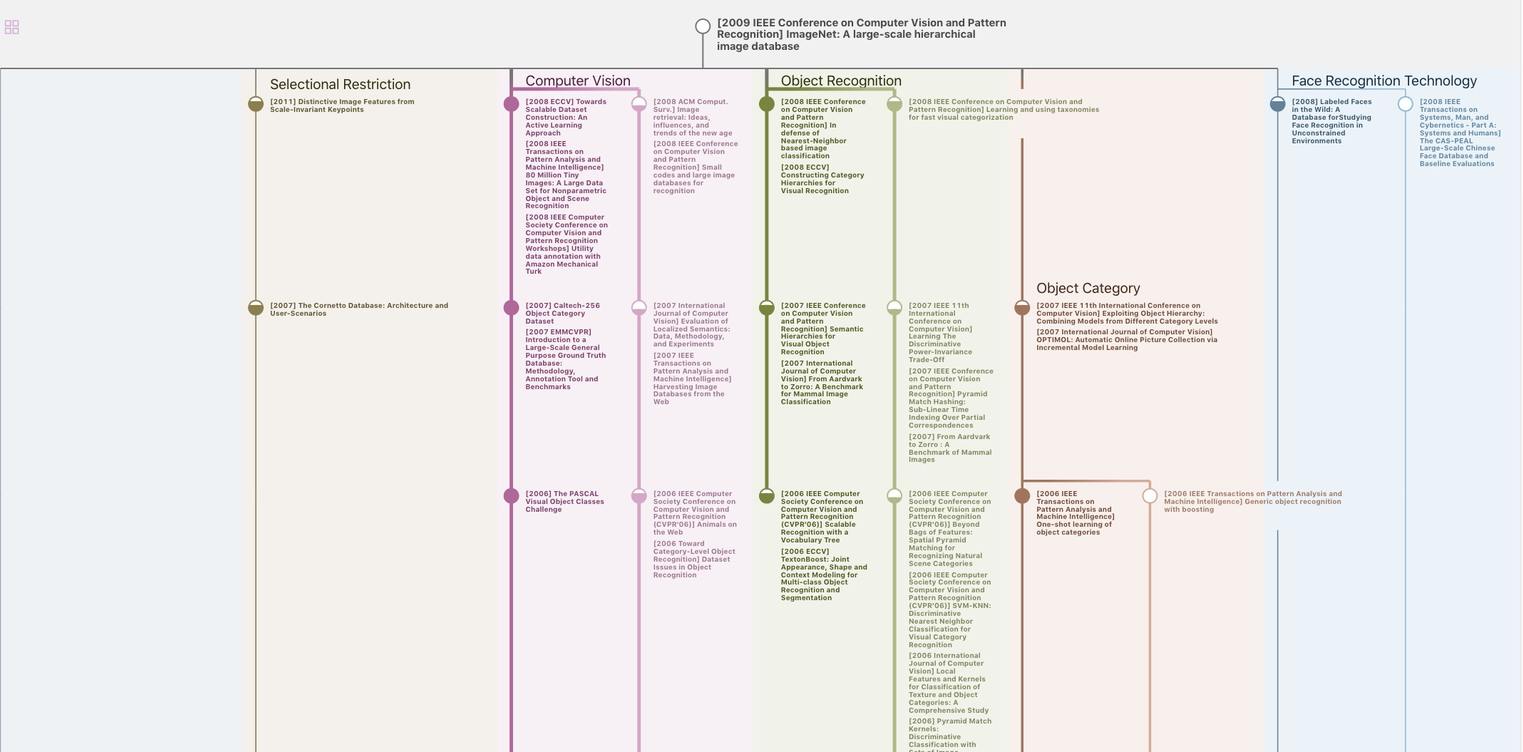
生成溯源树,研究论文发展脉络
Chat Paper
正在生成论文摘要