Evolving multi-user fuzzy classifier system with advanced explainability and interpretability aspects
Information Fusion(2023)
摘要
Evolving classifiers and especially evolving fuzzy classifiers have been established as a prominent technique for addressing the recent demands in building classifiers in an incremental online manner, based on target labels typically provided by a single user. We present a framework for an interactive evolving multi-user fuzzy classifier system with advanced explainability and interpretability aspects (EFCS-MU-AEI). Multiple users may provide their label feedback based on which own users’ classifiers are incrementally trained with evolving learning concepts. Its classification outputs are amalgamated by a specific ensembling scheme, respecting (i.) uncertainty in the class labels due to labeling ambiguities among the users and (ii.) different experience levels of the users as voting weights. A major focus thereby is concentrated on the explainability of classification outputs for the purpose to increase the quality (consistency and certainty) of the user (labeling) feedbacks. It is established to show reasons why certain decisions have been made and with which certainty levels and rule coverage degrees. The reasons are deduced from the most active rules, which are reduced in their length by a statistically-motivated instance-based feature importance level concept. Another major focus lies on the interpretability of extracted rules in order to represent understandable knowledge contained in the classification problem and especially to realize the labeling behaviors of different users for different parts of the feature space (= different sample groups). A specific incremental feature weighting technique, respecting label uncertainties from multiple users and sample forgetting weights (for handling drifts), as well as a fuzzy set merging process are proposed to aim for a high compactness and transparency of the rules.
更多查看译文
关键词
On-line interactive multi-user classification system,Evolving fuzzy classifiers,Explainability,Interpretability,Drift handling
AI 理解论文
溯源树
样例
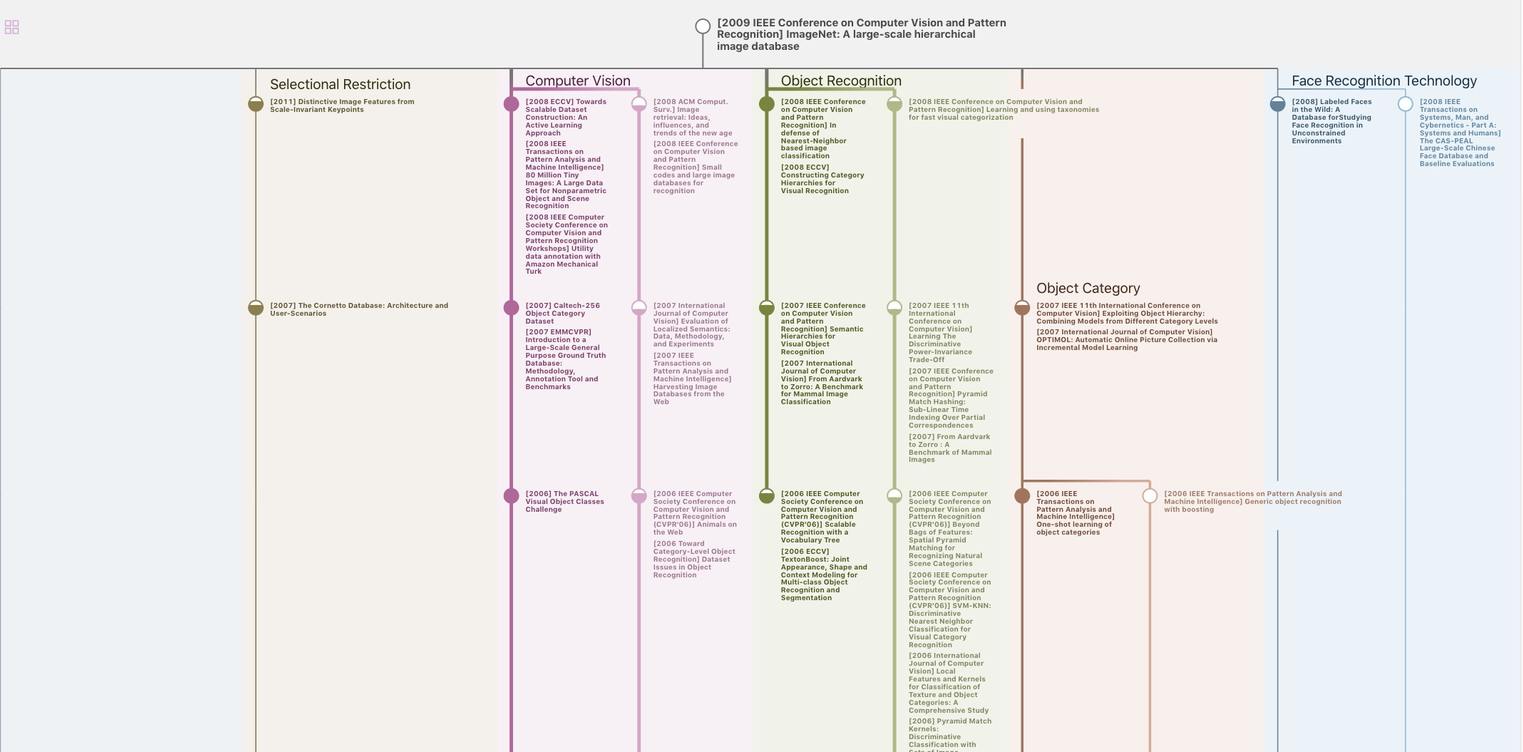
生成溯源树,研究论文发展脉络
Chat Paper
正在生成论文摘要