GP-NFSP: Decentralized task offloading for mobile edge computing with independent reinforcement learning
Future Generation Computer Systems(2023)
摘要
In Mobile Edge Computing (MEC), offloading tasks from mobile devices to edge servers may accelerate the processing speed and save the energy of the devices, hence improving device users’ quality of experience. Recently, reinforcement learning (RL) is increasingly used for offload decision making. RL seeks long-term cumulative benefits and is proved useful for a sequence of decisions, thus is well suited for the work. Due to privacy and security concerns, mobile devices may be unwilling to expose their local information, leading to a fully decentralized MEC environment. Independent RL (IRL) emerges as a promising solution for this scenario. However, IRL solutions are faced with the non-stationarity issue, which arises when the components are changing their policies. In this paper, we proposing adopting the Neural Fictitious Self-Play (NFSP) architecture for offload decision making. NFSP explicitly tackles the non-stationarity issue with the built-in self-play mechanism, and uses a mixed strategy consisting of deep RL and the past average strategy, which is approximated by supervised deep learning. Furthermore, we use the Proximal Policy Optimization (PPO) algorithm as the RL component and exploit the Gated Recurrent Unit (GRU) to deal with the partial-observability issue in fully decentralized MEC. We conduct extensive simulation experiment, the result of which shows that our method outperforms the raw IRL approaches, validating the effectiveness of our proposed method.
更多查看译文
关键词
Mobile edge computing,Computation offloading,Deep reinforcement learning,Neural fictitious self-play,Proximal policy optimization,Quality of experience
AI 理解论文
溯源树
样例
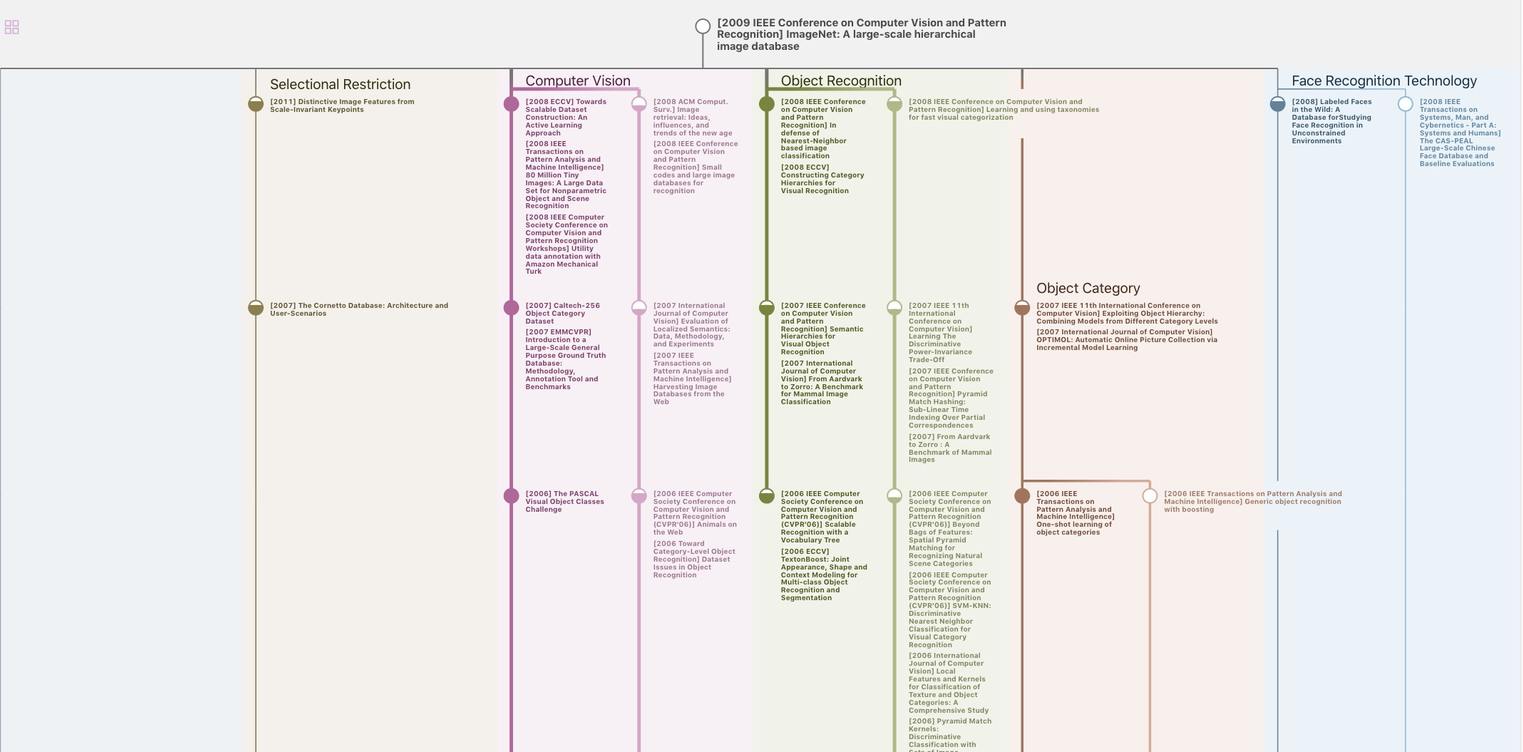
生成溯源树,研究论文发展脉络
Chat Paper
正在生成论文摘要