Knowledge-Driven Transfer Learning for Tree Species Recognition
2022 17th International Conference on Control, Automation, Robotics and Vision (ICARCV)(2022)
摘要
Deep learning methods on remote sensing data are an attractive approach in place of human observation for automating recognition of hundreds of thousands of tree species in nature. However, this approach requires a large amount of training data for each species, while actual data are scarce - only a small subset of tree species data can be acquired, notwithstanding the unknown, new species. To overcome the data scarcity challenge and to enable versatile recognition of known and unknown species, we propose a knowledge-driven transfer learning framework for tree species profiling, where a base model of multitasking graph neural network is trained on synthetic species data, which are generated from the universal botany domain knowledge and limited field measurement data. This base model is then transferred to a new multitasking graph neural network model to train on real tree data of limited availability. Our proposed species recognition framework was tested for profiling tree species by classifying a few species profile parameters and showed a significant improvement in the prediction accuracy in comparison to deep learning models trained on just real tree data.
更多查看译文
关键词
graph neural network,multitask modelling,transfer learning,tree species recognition,LiDAR point cloud
AI 理解论文
溯源树
样例
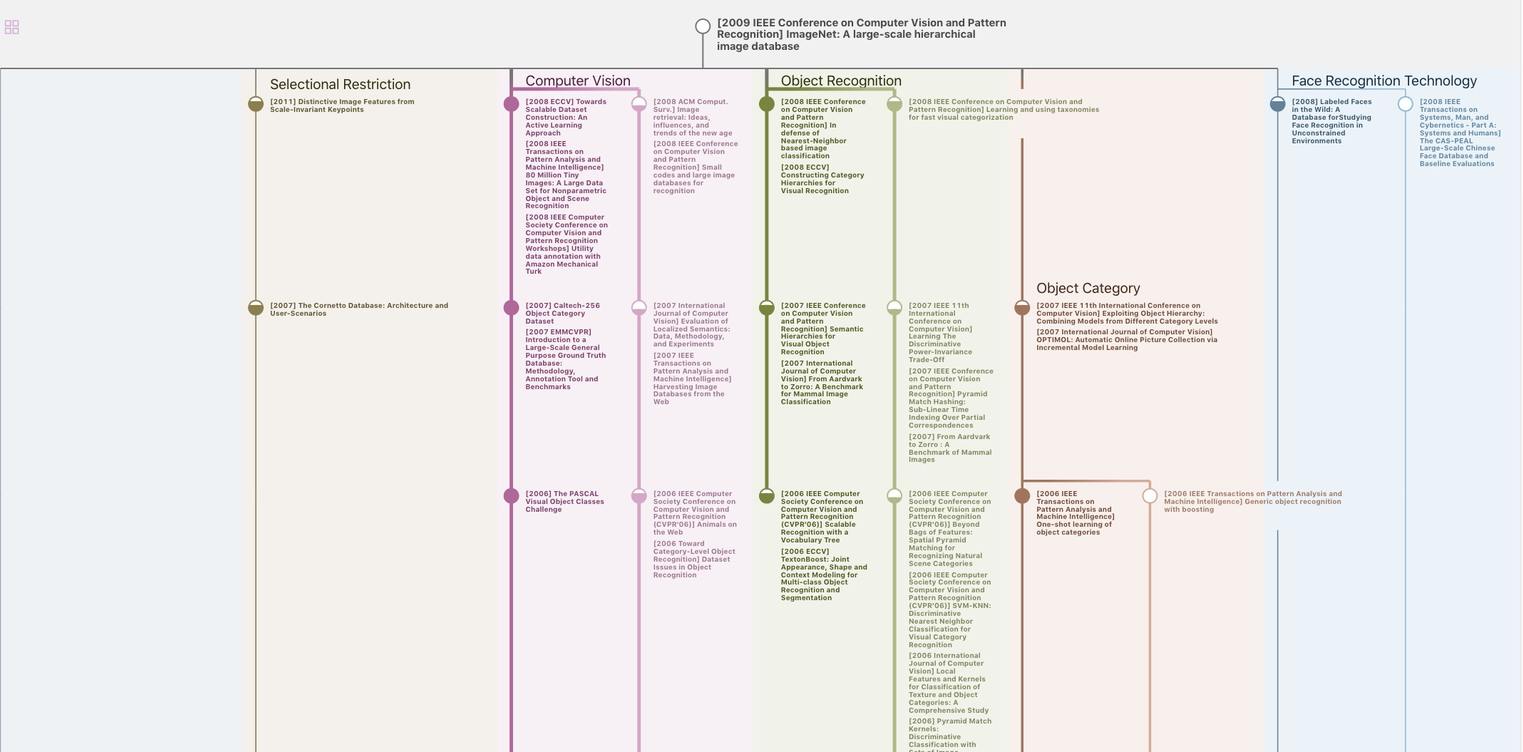
生成溯源树,研究论文发展脉络
Chat Paper
正在生成论文摘要