Clustering via multiple kernel k -means coupled graph and enhanced tensor learning
Applied Intelligence(2022)
摘要
Kernel k -means based and spectral clustering (SC) based multi-kernel clustering (MKC) has been widely used in recent years due to the efficiency in grouping nonlinear data. However, (1) the methods based on the above two categories only focus on clustering indicator matrix learning or graph learning, few of them have noticed the connection between the two; and (2) it is hard for existing methods to consider the high-order similarities of all pre-defined base kernels, which leads to the waste of inter-kernel information. To solve these problems, we propose kernel k -means coupled graph and enhanced tensor learning (KKG-ETL). Concretely, a new graph learning paradigm, kernel k -means coupled graph (KKG), is proposed to establish the theoretical relation between clustering indicator matrix and affinity graph. Therefore, a better candidate affinity graph can be obtained for each base kernel. Then, enhanced tensor learning (ETL) is proposed to capture the high-order similarities of all candidate graphs via an auto-weighted Schatten p -norm. In this framework, we integrate the indication properties of kernel k -means and the manifold excavation capability of SC, while exploring the high-order similarities among all kernels. Comprehensive experiments on 8 widely used datasets verify the validity and feasibility of our proposed KKG-ETL.
更多查看译文
关键词
Multiple kernel clustering, Kernel k-means, Spectral clustering, Tensor learning, Schatten p-norm
AI 理解论文
溯源树
样例
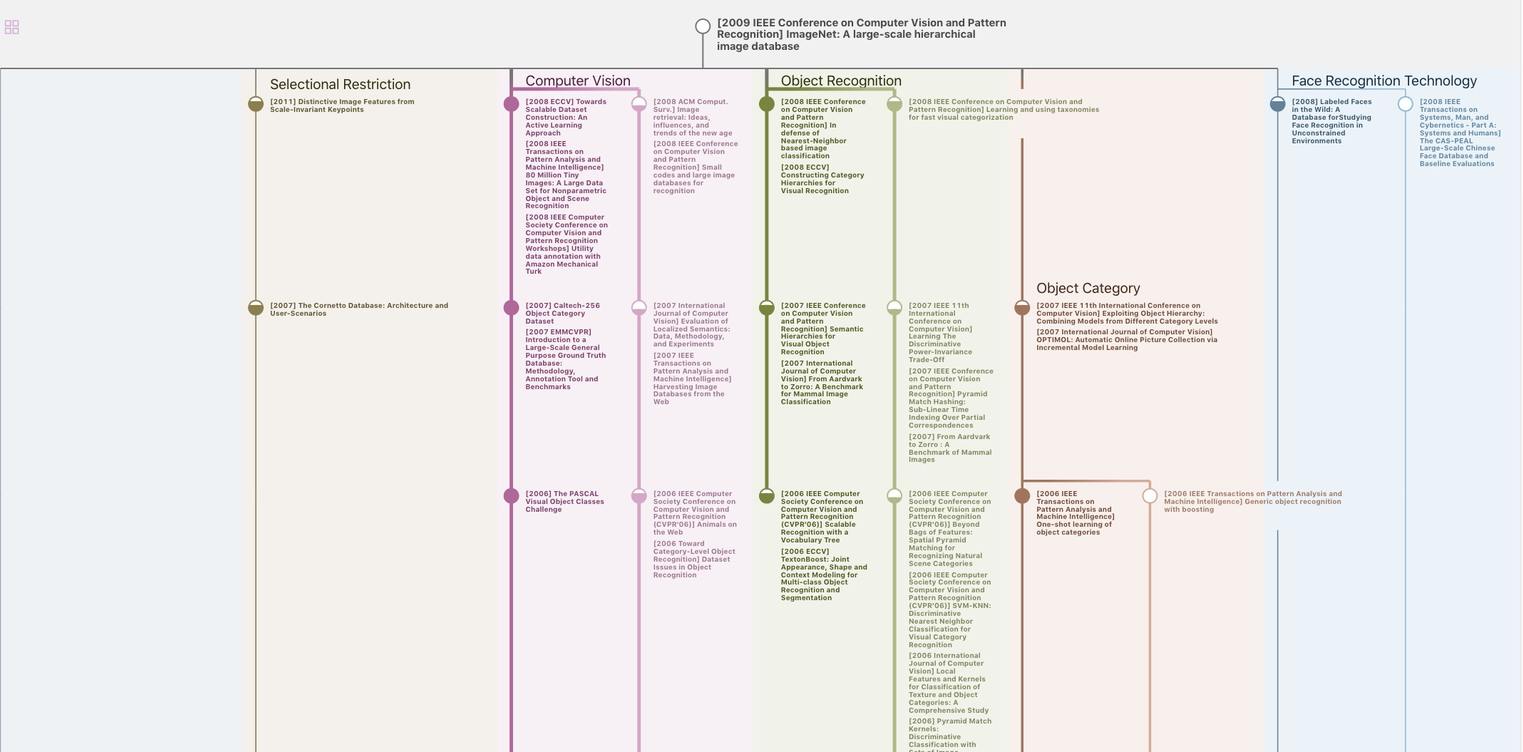
生成溯源树,研究论文发展脉络
Chat Paper
正在生成论文摘要