Multi-agent deep reinforcement learning based distributed control architecture for interconnected multi-energy microgrid energy management and optimization
Energy Conversion and Management(2023)
摘要
Environmental and climate change concerns are pushing the rapid development of new energy resources (DERs). The Energy Internet (EI), with the power-sharing functionality introduced by energy routers (ERs), offers an appealing alternative for DER systems. However, previous centralized control schemes for EI systems that follow a top-down architecture are unreliable for future power systems. This study proposes a distributed control scheme for bottom-up EI architecture. Second, model-based distributed control methods are not sufficiently flexible to deal with the complex uncertainties associated with multi-energy demands and DERs. A novel model-free/data-driven multiagent deep reinforcement learning (MADRL) method is proposed to learn the optimal operation strategy for the bottom-layer microgrid (MG) cluster. Unlike existing single-agent deep reinforcement learning methods that rely on homogeneous MG settings, the proposed MADRL adopts a form of decentralized execution, in which agents operate independently to meet local customized energy demands while preserving privacy. Third, an attention mechanism is added to the centralized critic, which can effectively accelerate the learning speed. Considering the bottom-layer power exchange request and the predicted electricity price, model predictive control of the upper layer determines the optimal power dispatching between the ERs and main grid. Simulations with other alternatives demonstrate the effectiveness of the proposed control scheme.
更多查看译文
关键词
Multiagent deep reinforcement learning,Energy management,Energy Internet,Bottom-up,Distributed control
AI 理解论文
溯源树
样例
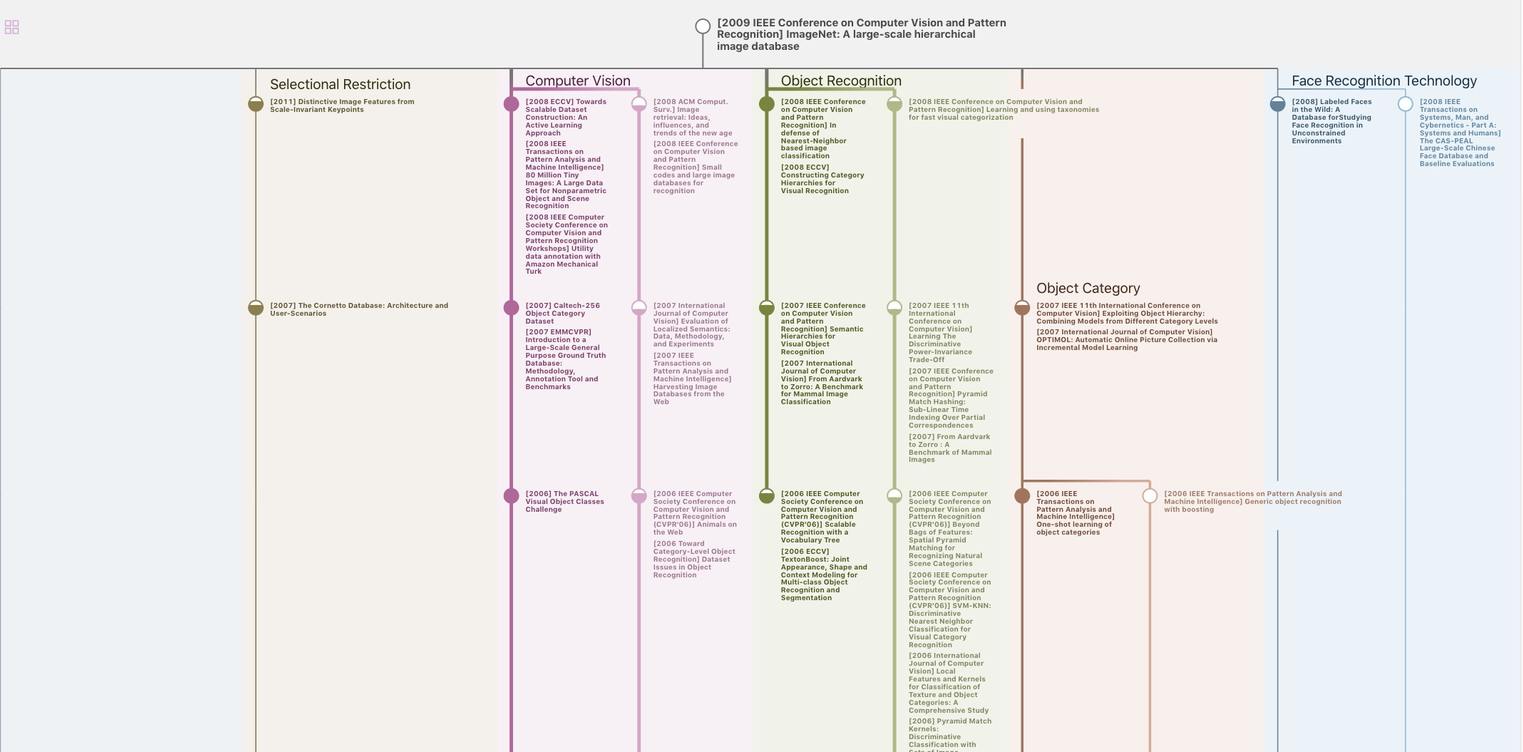
生成溯源树,研究论文发展脉络
Chat Paper
正在生成论文摘要