Thalassemia Diagnosis Through Medical Imaging: A New Artificial Intelligence-Based Framework
2022 International Conference on Smart Systems and Power Management (IC2SPM)(2022)
摘要
Thalassemia is the most common single-gene disorder throughout the world and represents a major public health problem resulting in abnormal ratios of hemoglobin subunits. It is widely spread throughout the Mediterranean region, Middle East, Southeast Asia, and some parts of Africa. There are different types of Thalassemia characterized by abnormal hemoglobin production. Most of the current hemoglobinopathy screening methods include High Performance Liquid Chromatography (HPLC), hemoglobin electrophoresis, screening of Polymerase Chain Reaction (PCR) mutations, and Deoxyribonucleic Acid (DNA) tests. However, all these methods are costly and require specialized instrumentation and trained technicians. Despite some studies used Artificial Intelligence (AI) they focused merely on Machine Learning (ML) and results were not optimal. This study aims to design a new AI-based framework for Thalassemia diagnosis using Deep Learning (DL) and a new combination of metrics for evaluation. This was achieved through the development and evaluation of a supervised semantic image segmentation model, and the implementation of different data engineering methods such as data annotation, augmentation, pre-processing, and preparation. Transfer learning was utilized and the Prediction Time Augmentation (PTA) was employed to get smoother and more accurate predictions. Quantitative results showed that, the mean Intersection Over Union (IoU) score of prediction of Thalassemia was 88% with PTA and 82% without PTA. Results also showed that as the combined metric of loss scores decreases the prediction of Thalassemia increases. Qualitative results showed that the final prediction of Thalassemia focuses on the Codocytes and labels the other unidentified cells as the background. Also, the resulting image was smoother and less bulky than the original annotated ground truth, and thus could be feasibly diagnosed. As a future prospect we aim to implement more algorithms and extend the diagnosis to include other diseases.
更多查看译文
关键词
Thalassemia,Codocyte,Prediction time augmentation,Combined Metric Loss,Intersection over Union,Medical Image Processing,Artificial Intelligence,Deep learning
AI 理解论文
溯源树
样例
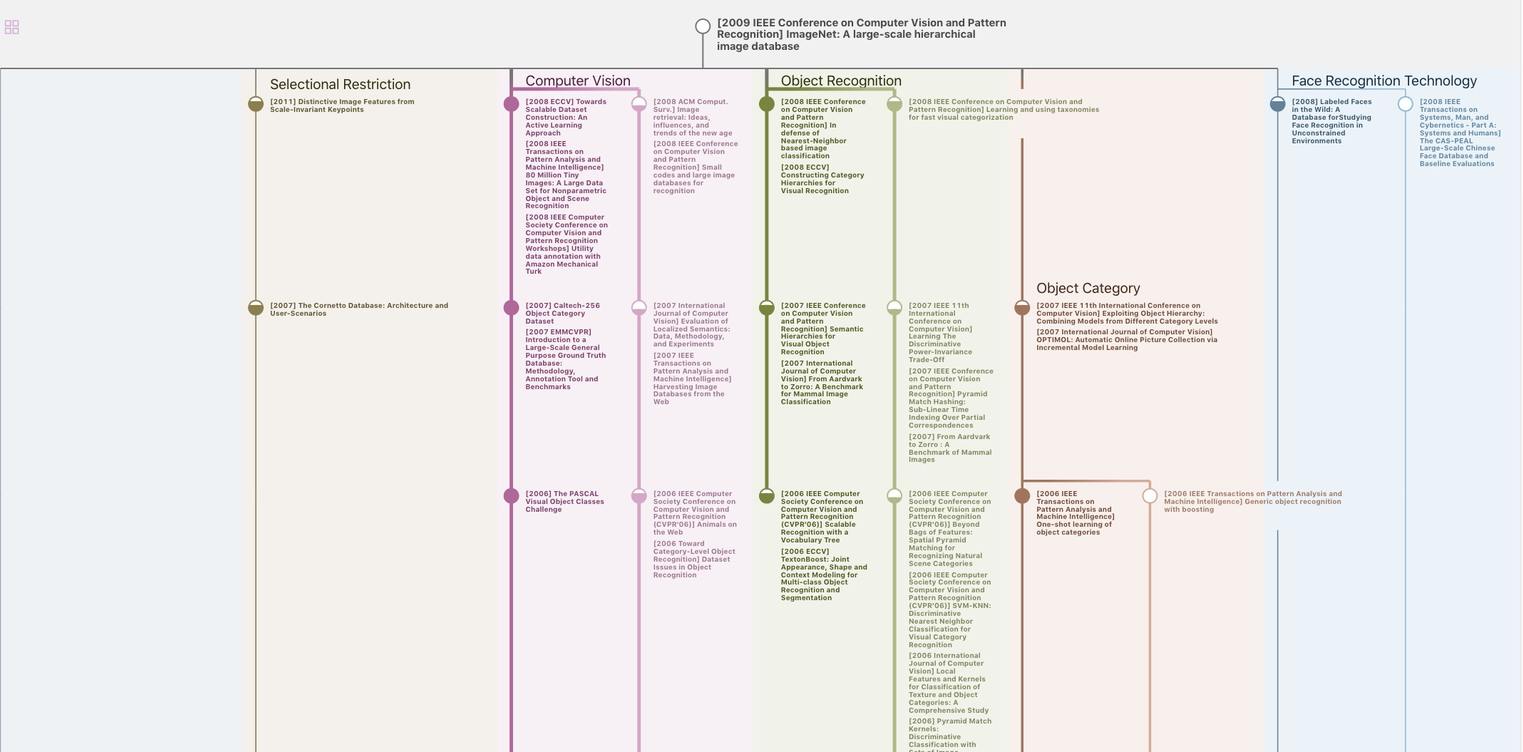
生成溯源树,研究论文发展脉络
Chat Paper
正在生成论文摘要