LCN: Label Correction Based on Network Prediction for Cross-Modal Retrieval with Noisy Labels
2022 Asia-Pacific Signal and Information Processing Association Annual Summit and Conference (APSIPA ASC)(2022)
摘要
Cross-modal retrieval enables users to obtain information related to the input query by crossing different modalities (e.g., from a text to an image). Recently, the research community has tackled the challenging situation where noisy labels are included in the training data. The state-of-the-art method trains the network so that weights for correct labels in the loss function can be increased. However, we newly found that the network is finally overfitted to the remaining noisy labels as training progresses. Motivated by this finding, this paper proposes a method called Label Correction based on Network prediction (LCN) to correct noisy labels, unlike the state-of-the-art method that leaves noisy labels on training. Specifically, we assume that noisy labels are irrelevant to the data features. Based on this assumption, we can consider predicted labels (obtained by network prediction) that are relevant to data features are more reliable than given labels. Moreover, a novel scheme for adjusting the number of the predicted labels used for training is introduced into our method. This is useful for solving the problem that the network prediction is ambiguous in the early stages of training. Experimental results show that LCN dramatically alleviates the remaining noisy labels to achieve high performance in cross-modal retrieval in the real-world scenario.
更多查看译文
关键词
label correction,labels,network prediction,retrieval,cross-modal
AI 理解论文
溯源树
样例
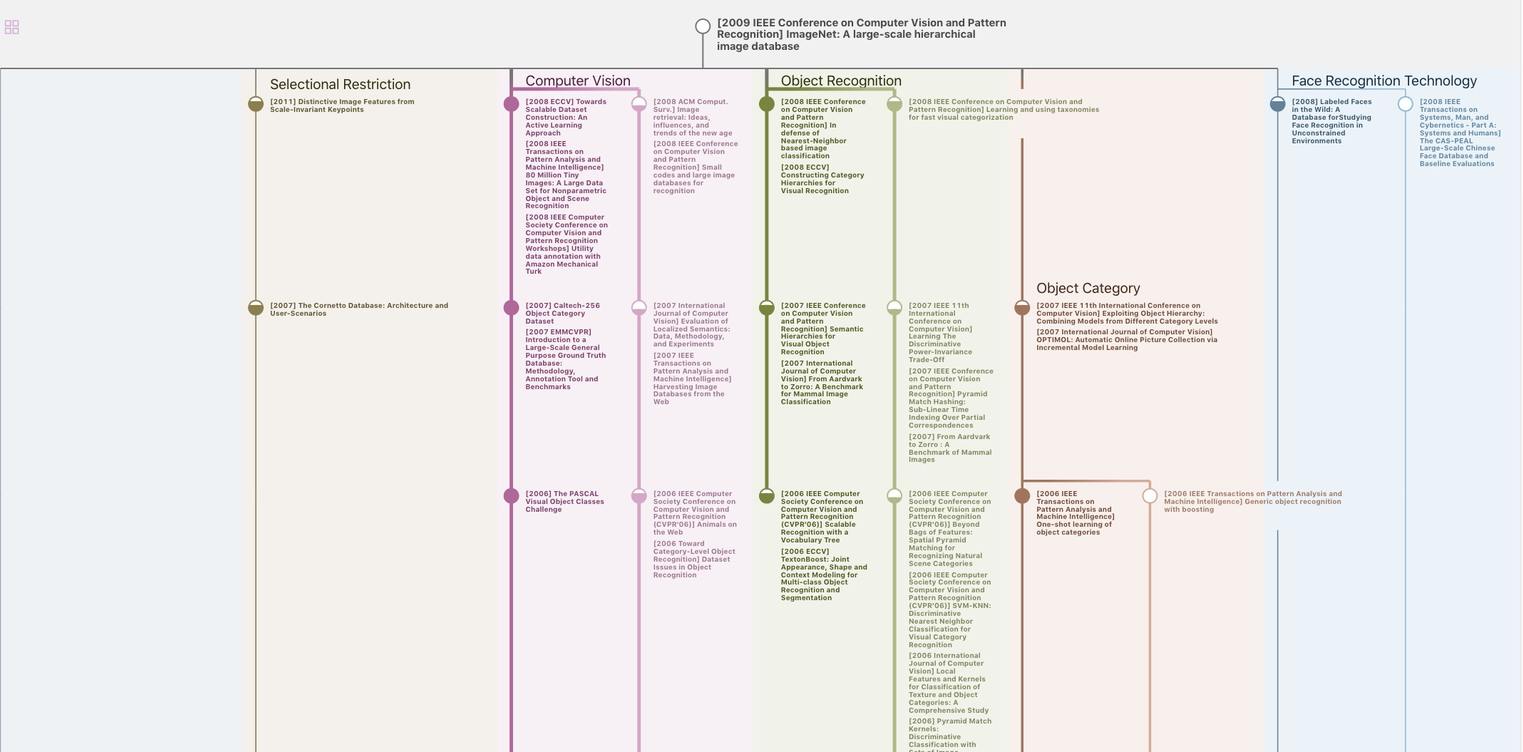
生成溯源树,研究论文发展脉络
Chat Paper
正在生成论文摘要