Mastering Diverse Domains through World Models
arxiv(2023)
摘要
General intelligence requires solving tasks across many domains. Current reinforcement learning algorithms carry this potential but are held back by the resources and knowledge required to tune them for new tasks. We present DreamerV3, a general and scalable algorithm based on world models that outperforms previous approaches across a wide range of domains with fixed hyperparameters. These domains include continuous and discrete actions, visual and low-dimensional inputs, 2D and 3D worlds, different data budgets, reward frequencies, and reward scales. We observe favorable scaling properties of DreamerV3, with larger models directly translating to higher data-efficiency and final performance. Applied out of the box, DreamerV3 is the first algorithm to collect diamonds in Minecraft from scratch without human data or curricula, a long-standing challenge in artificial intelligence. Our general algorithm makes reinforcement learning broadly applicable and allows scaling to hard decision-making problems.
更多查看译文
关键词
diverse domains,models,world
AI 理解论文
溯源树
样例
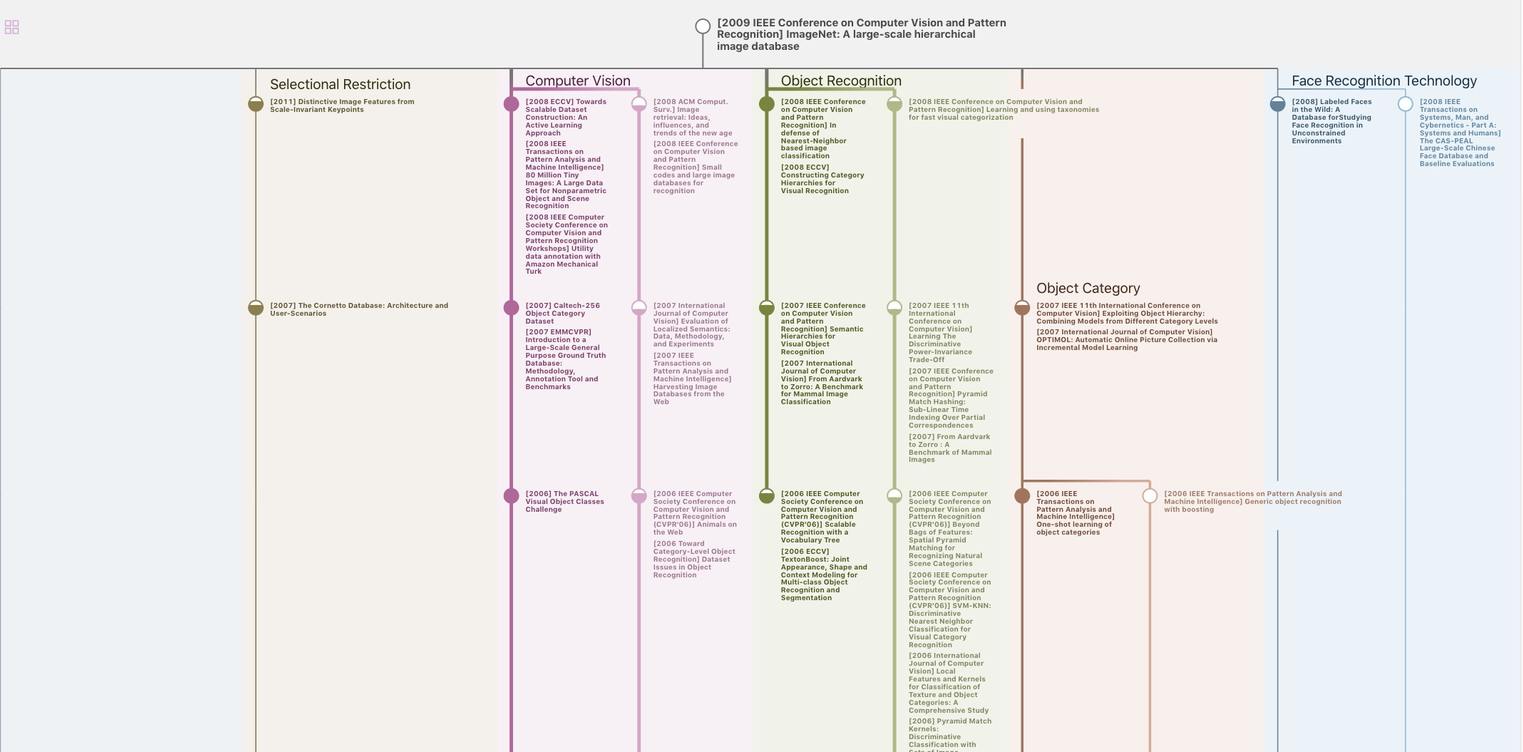
生成溯源树,研究论文发展脉络
Chat Paper
正在生成论文摘要