Student Dropout Prediction using 1D CNN-LSTM with Variational Autoencoder Oversampling
2022 IEEE Latin American Conference on Computational Intelligence (LA-CCI)(2022)
摘要
Student dropout represents a social, resource and time loss for everyone involved. By identifying students with the potential to evade, it is possible to take the necessary measures to prevent that from happening. This paper investigates different techniques for classification using a real students dataset that is multivariate, sequential and imbalanced. To address imbalance, an approach using weighted loss function and an approach using synthetic data generated through a combination of variational autoencoder and the Adaptive Synthetic (ADASYN) technique were investigated. In addition, we propose two models for predicting evasion using LSTM and 1D CNN networks to take advantage of the multivariate sequential property. The experiments showed that the approach only with LSTM architecture in the dynamic module using the preprocessed original dataset and without the use of weighted loss function obtained the best mean sensitivity (0.9676) among the investigated proposals. The LSTM architecture in the dynamic module using the preprocessed original dataset with weighted loss function had better specificity (0.9558) and area under curve (0.9735). Both had the same mean accuracy of 0.9549.
更多查看译文
关键词
student dropout,prediction,imbalance,autoencoder,LSTM network,convolutional network
AI 理解论文
溯源树
样例
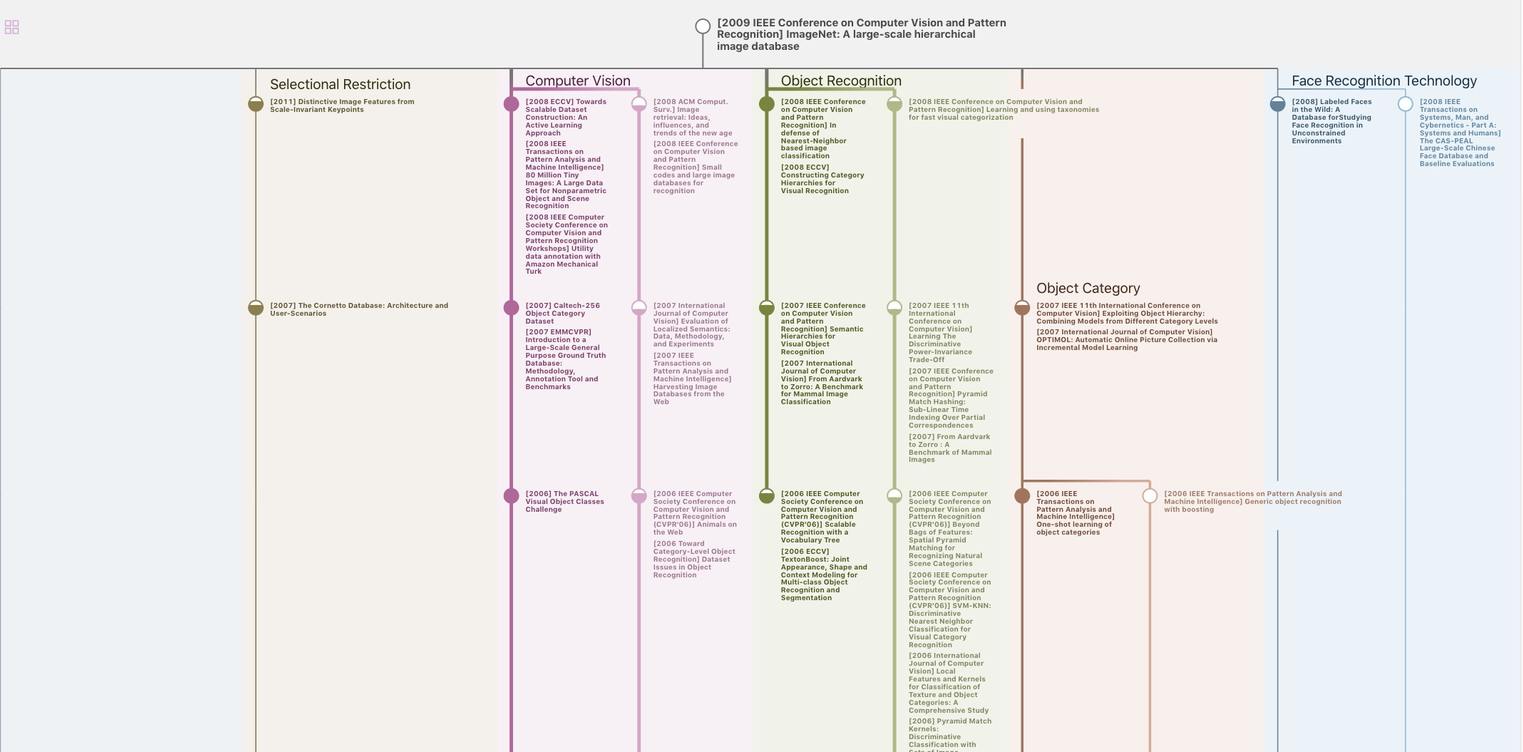
生成溯源树,研究论文发展脉络
Chat Paper
正在生成论文摘要