Graph neural networks for efficient learning of mechanical properties of polycrystals
Computational Materials Science(2023)
摘要
We present graph neural networks (GNNs) as an efficient and accurate machine learning approach to predict mechanical properties of polycrystalline materials. Here, a GNN was developed based on graph representation of polycrystals incorporating only fundamental features of grains including their crystallographic orientation, size, and grain neighbor connectivity information. We tested our method on modeling stiffness and yield strength of α-Ti microstructures, varying in their crystallographic texture. We find the GNN predicts both properties with high accuracy with mean relative errors of ∼1% for unseen microstructures from a given set of textures and <2% for microstructures of unseen texture, even when presented with limited training data. This accuracy is comparable to methods that require high-resolution three-dimensional (3D) microstructure data, such as 3D convolutional neural networks (3D-CNNs) and models that depend on the computation of spatial statistics. The present results show that graph-based deep learning is a promising framework for property prediction, especially considering the high cost associated with obtaining high-resolution 3D microstructure data and the general scarcity of experimental materials datasets.
更多查看译文
关键词
Titanium,Texture,Graphs,Deep learning,Strength,Stiffness
AI 理解论文
溯源树
样例
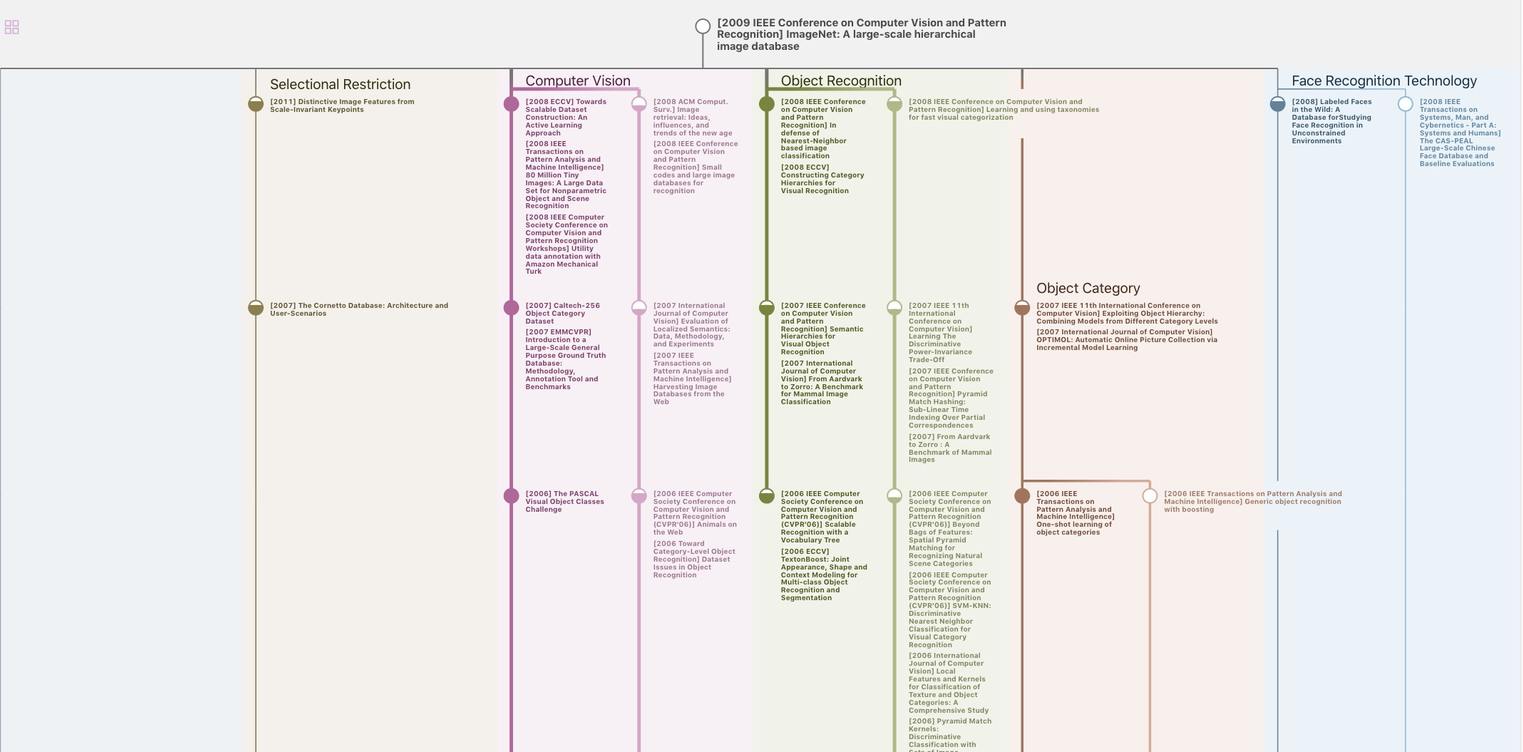
生成溯源树,研究论文发展脉络
Chat Paper
正在生成论文摘要