Physics-informed neural networks for data-driven simulation: Advantages, limitations, and opportunities
Physica A: Statistical Mechanics and its Applications(2023)
摘要
The last decade has seen a rise in the number and variety of techniques available for data-driven simulation of physical phenomena. One of the most promising approaches is Physics-Informed Neural Networks (PINNs), which can combine both data, obtained from sensors or numerical solvers, and physics knowledge, expressed as partial differential equations. In this work, we investigated the suitability of PINNs to replace current available numerical methods for physics simulations. Although the PINN approach is general and independent of the complexity of the underlying physics equations, a selection of typical heat transfer and fluid dynamics problems was proposed and multiple PINNs were comprehensibly trained and tested to solve them. When PINNs were used as learned simulators, the outcome of our experiments was not entirely satisfactory as not enough accuracy was achieved even though optimal configurations and long training times were used. The main cause for this limitation was found to be the lack of adequate activation functions and specialized architectures, since they proved to have a notable impact on the final accuracy of each model. In turn, PINN architectures showed an accurate behavior when used for parameter inference of partial differential equations from data.(c) 2022 Elsevier B.V. All rights reserved.
更多查看译文
关键词
Deep learning,Physics-Informed Neural Networks,Learned simulators,Data-driven simulations
AI 理解论文
溯源树
样例
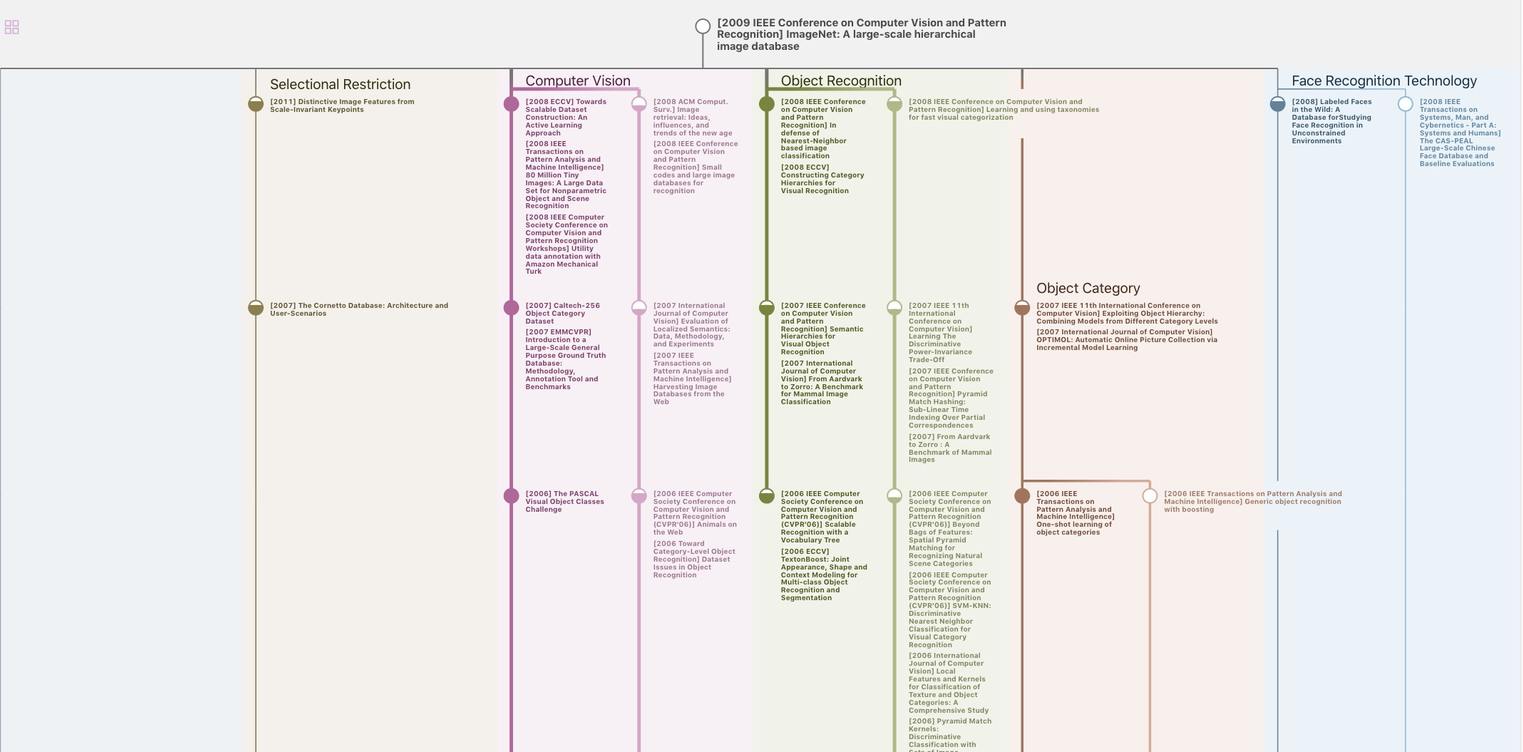
生成溯源树,研究论文发展脉络
Chat Paper
正在生成论文摘要