Hybrid Neural Network Based Models for Evapotranspiration Prediction Over Limited Weather Parameters
IEEE Access(2023)
摘要
Evapotranspiration can be used to estimate the amount of water required by agriculture projects and green spaces, playing a key role in water management policies that combat the hydrological drought, which assumes a structural character in many countries. In this context, this work presents a study on reference evapotranspiration (
$ET_{o}$
) estimation models, having as input a limited set of meteorological parameters, namely: temperature, humidity, and wind. Since solar radiation (SR) is an important parameter in the determination of
$ET_{o}$
, SR estimation models are also developed. These
$ET_{o}$
and SR estimation models compare the use of Artificial Neural Networks (ANN), Long Short Term Memory (LSTM), Gated Recurrent Unit (GRU), Recurrent Neural Network (RNN), and hybrid neural network models such as LSTM-ANN, RNN-ANN, and GRU-ANN. Two main approaches were taken for
$ET_{o}$
estimation: (i) directly use those algorithms to estimate
$ET_{o}$
, and (ii) estimate solar radiation first and then use that estimation together with other meteorological parameters in a method that predicts
$ET_{o}$
. For the latter case, two variants were implemented: the use of the estimated solar radiation as (ii.1) a feature of the neural network regressors, and (ii.2) the use of the Penman-Monteith method (a.k.a. FAO-56PM method, adopted by the United Nations Food and Agriculture Organization) to compute
$ET_{o}$
, which has solar radiation as one of the input parameters. Using experimental data collected from a weather station (WS) located in Vale do Lobo (Portugal), the later approach achieved the best result with a coefficient of determination
$(R^{2})$
of 0.977. The developed model was then applied to data from eleven stations located in Colorado (USA), with very distinct climatic conditions, showing similar results to the ones for which the models were initially designed (
$R^{2}>0.95$
), proving a good generalization. As a final notice, the reduced-set features were carefully selected so that they are compatible with free online weather forecast services.
更多查看译文
关键词
Artificial neural networks,evapotranspiration,public garden,smart irrigation,solar radiation
AI 理解论文
溯源树
样例
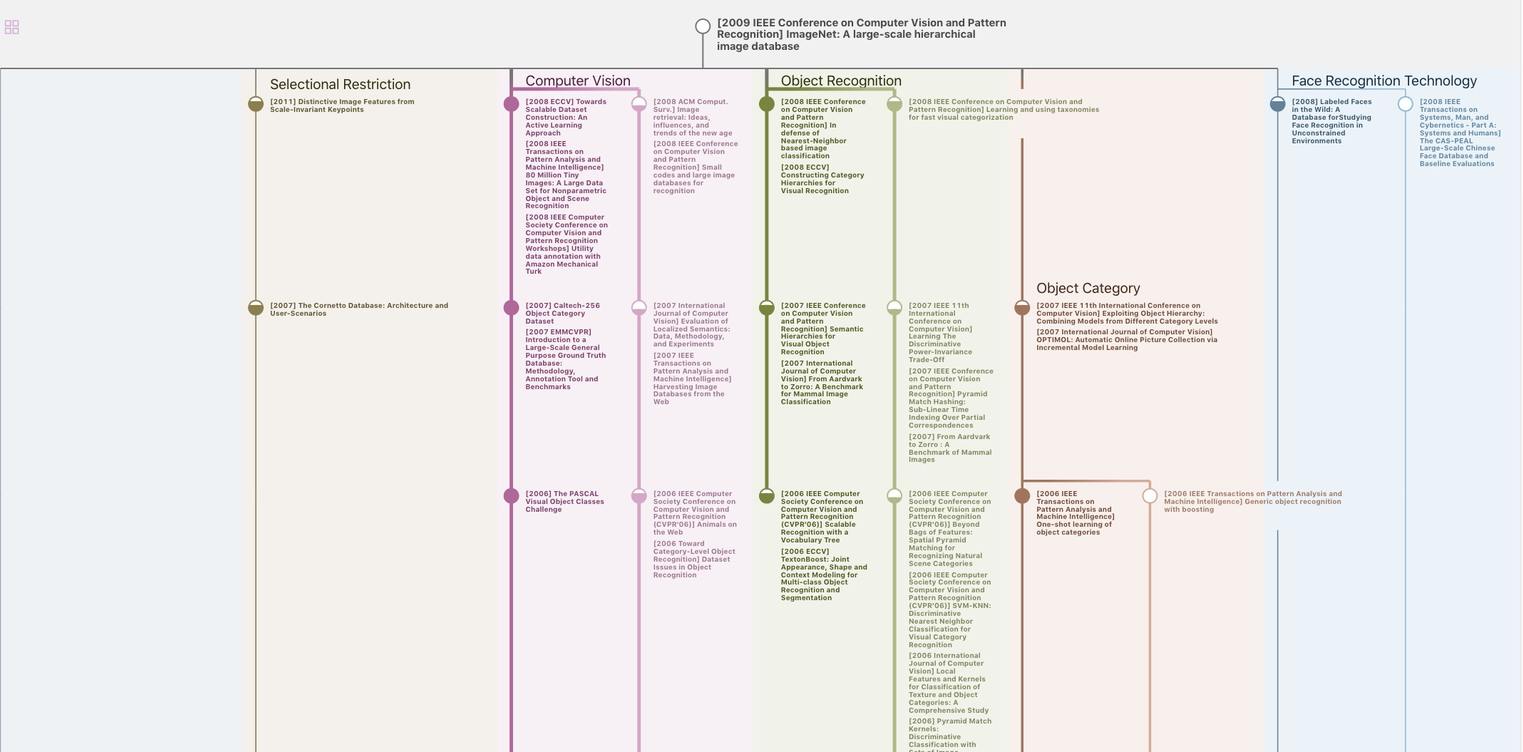
生成溯源树,研究论文发展脉络
Chat Paper
正在生成论文摘要