Training trajectories, mini-batch losses and the curious role of the learning rate
arxiv(2023)
摘要
Stochastic gradient descent plays a fundamental role in nearly all applications of deep learning. However its ability to converge to a global minimum remains shrouded in mystery. In this paper we propose to study the behavior of the loss function on fixed mini-batches along SGD trajectories. We show that the loss function on a fixed batch appears to be remarkably convex-like. In particular for ResNet the loss for any fixed mini-batch can be accurately modeled by a quadratic function and a very low loss value can be reached in just one step of gradient descent with sufficiently large learning rate. We propose a simple model that allows to analyze the relationship between the gradients of stochastic mini-batches and the full batch. Our analysis allows us to discover the equivalency between iterate aggregates and specific learning rate schedules. In particular, for Exponential Moving Average (EMA) and Stochastic Weight Averaging we show that our proposed model matches the observed training trajectories on ImageNet. Our theoretical model predicts that an even simpler averaging technique, averaging just two points a many steps apart, significantly improves accuracy compared to the baseline. We validated our findings on ImageNet and other datasets using ResNet architecture.
更多查看译文
关键词
training,learning,rate,mini-batch
AI 理解论文
溯源树
样例
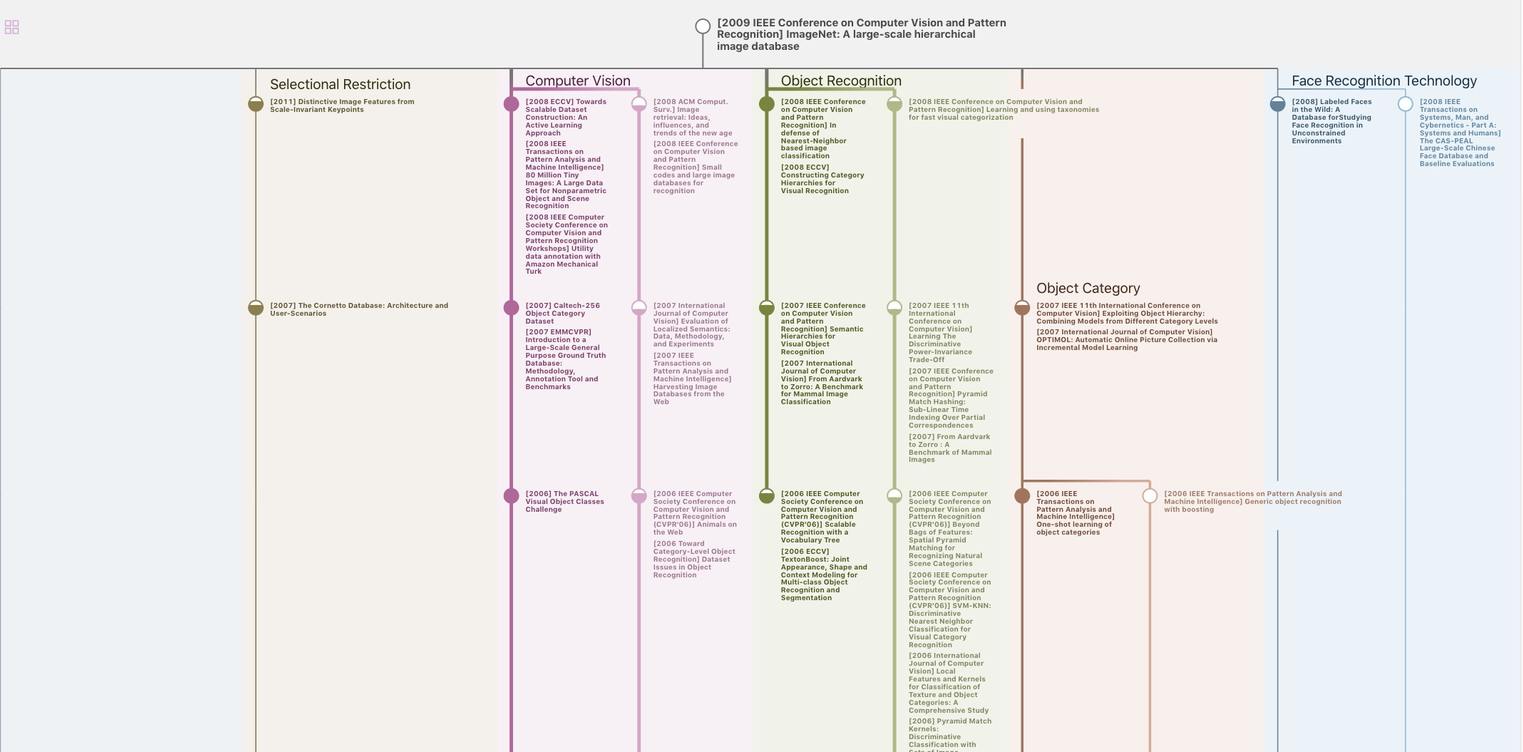
生成溯源树,研究论文发展脉络
Chat Paper
正在生成论文摘要