Two-stage framework for automatic diagnosis of multi-task in essential tremor via multi-sensory fusion parameters
Journal of King Saud University - Computer and Information Sciences(2022)
摘要
Essential tremor (ET) is one of the most common movement disorders in adults, and its early assessment and diagnosis are crucial for disease management in movement disorders. Nowadays, the severity of tremors can only be diagnosed and evaluated by laboratory tests. However, there are certain subjective factors in traditional assessment methods by the naked eye of a neurologist, which often leads to some biases. This study proposes a novel multi-modal signals-based automated quantitative assessment system for tremor severity. Specifically, we develop a two-stage framework that performs posture pattern recognition on the raw data, then extracts kinematic parameters to build an individualized model for each task. Besides, we established a strict clinical paradigm, including 121 ET patients, finely evaluated by a committee of neurologists to build a high-quality database. The models' performances showed that most of the kinematic parameters designed in this study could effectively map the severity of the tremor. The F1 score for classification of the posture task based on deep learning networks was 99.02%, and the quantification of symptom scores based on machine learning models ranged from 94.77 to 99.00%. These results demonstrate the effectiveness of the proposed framework can automatically provide objective and accurate scores for ET symptom assessment.
更多查看译文
关键词
Essential tremor,Automatic diagnosis,Multi-sensory fusion,Two-stage,Deep learning
AI 理解论文
溯源树
样例
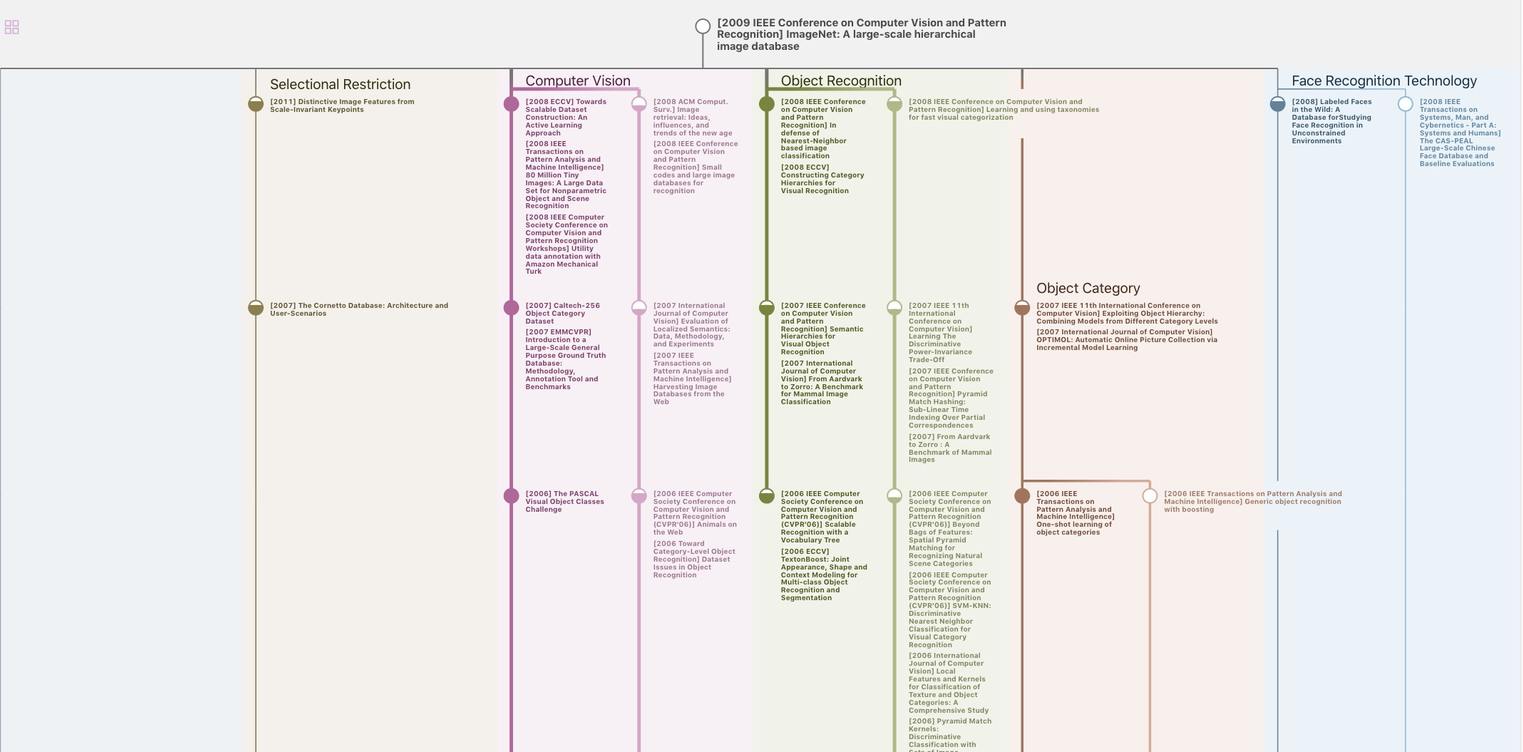
生成溯源树,研究论文发展脉络
Chat Paper
正在生成论文摘要