Motion artifact correction in fetal MRI based on a Generative Adversarial network method
Biomedical Signal Processing and Control(2023)
摘要
Fetal MR imaging is subject to artifacts, where the most common type is caused by motion. These artifacts can appear as blurring and/or ghosting in the affected sequences. Currently if the motion artifact is severe or covers essential fetal tissue, the sequence acquisition must be repeated for diagnostic decision-making. We propose a novel deep learning network to reduce and remove motion artifacts in fetal MRIs. It follows a Generative Adversarial Network (GAN) framework where the Generator consists of an Autoencoder structure containing Residual blocks with Squeeze and Excitation (SE), and the Discriminator uses a sequential Convolutional Neural Network (CNN) design. The loss function is composed of weighted subcomponents involving WGAN, L1, and perceptual losses. The proposed network was trained on a synthetically created motion artifact dataset, and further validated on real motion-degraded images. The creation of the synthetic dataset consisted of randomly modifying the k-space of each scan. On the synthetic dataset, the proposed network achieved an average SSIM and PSNR of 93.7 % and 33.5 dB respectively. For the real motion affected dataset, the proposed network attained an average BRISQUE score of 21.1. These results outperformed current state-of-the-art techniques including BM3D, RED-Net, NLM filtering, and WGAN-VGG. The presented network facilitates rapid and accurate post-processing for fetal MRI. It can also improve diagnostic accuracy and can save time and money by reducing the number of rescans caused by severe motion artifacts.
更多查看译文
关键词
Deep Learning1,Fetal Magnetic Resonance Imaging2,Generative Adversarial Network3,Image Denoising4,Motion Artifacts5
AI 理解论文
溯源树
样例
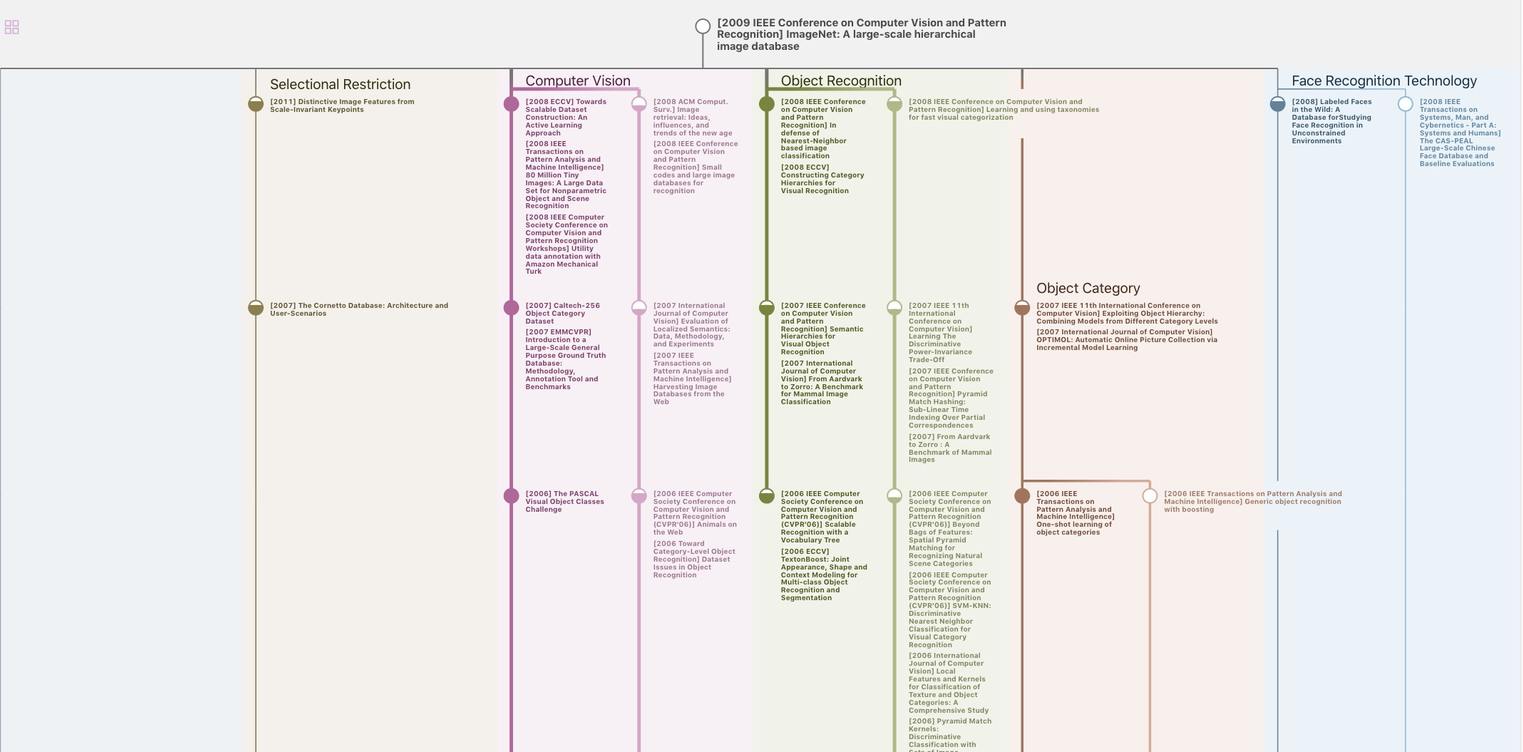
生成溯源树,研究论文发展脉络
Chat Paper
正在生成论文摘要