Gastrointestinal abnormality detection and classification using empirical wavelet transform and deep convolutional neural network from endoscopic images
Ain Shams Engineering Journal(2023)
摘要
With an intention to assist gastroenterologists, this work proposes an intelligent method to classify alimentary canal diseases such as Barrett’s, Esophagitis, Hemorrhoids, Polyps, and Ulcerative colitis by using empirical wavelet transform (EWT) and convolutional neural network (CNN). Here, a publicly available HyperKvasir dataset is used for the experimental work. The framework starts with several image pre-processing steps followed by the implementation of EWT. EWT helps to decompose the image into several modes and extract specific patterns in the images. These decomposed images are then fed into the proposed deep CNN for disease classification in two-levels. Finally, the proposed model is evaluated based on several performance metrics. The result shows 96.65% accuracy, 0.9298 Matthews correlation coefficient (MCC) in the first level, and 94.25% accuracy, 0.8108 MCC in the second level of classification. Lastly, to justify the efficacy of the proposed method, a comparative study is carried out with other contemporary techniques.
更多查看译文
关键词
Artificial intelligence,Discrete wavelet transform,Empirical wavelet transform,Convolutional neural network,Gastrointestinal tract
AI 理解论文
溯源树
样例
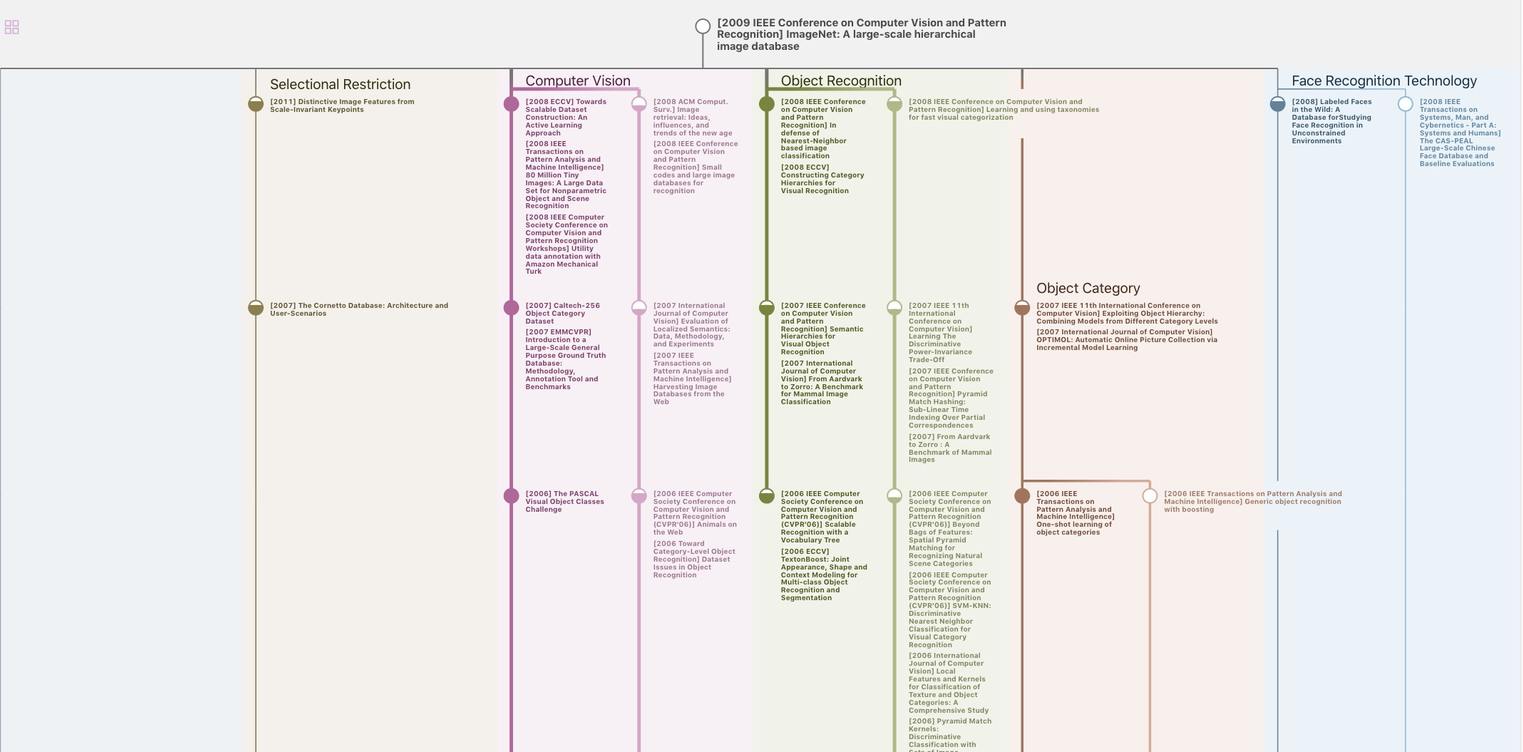
生成溯源树,研究论文发展脉络
Chat Paper
正在生成论文摘要