Ensemble Outperforms Single Models in Brain Tumor Segmentation.
International Workshop on Brainlesion: Glioma, Multiple Sclerosis, Stroke and Traumatic Brain Injuries (BrainLes)(2021)
摘要
Brain tumor segmentation remains an open and popular challenge, for which countless medical image segmentation models have been proposed. Based on the platform that BraTS challenge 2021 provided for researchers, we implemented a battery of cutting-edge deep neural networks, such as nnU-Net, UNet++, CoTr, HRNet, and Swin-Unet to directly compare performances amongst distinct models. To improve segmentation accuracy, we first tried several modification techniques (e.g., data augmentation, region-based training, batch-dice loss function, etc.). Next, the outputs from the five best models were averaged using a final ensemble model, of which four models in the committee were organized in different architectures. As a result, the strengths of every single model were amplified by the aggregation. Our model took one of the best performing places in the Brain Tumor Segmentation (BraTS) 2021 competition amongst over 1200 excellent researchers from all over the world, which achieved Dice score of 0.9256, 0.8774, 0.8576 and Hausdor Distances (95%) of 4.36, 14.80, 14.49 for whole tumor, tumor core, and enhancing tumor respectively.
更多查看译文
关键词
Brain tumor segmentation,Ensemble learning,nnU-Net,UNet plus,CoTr,HRNet
AI 理解论文
溯源树
样例
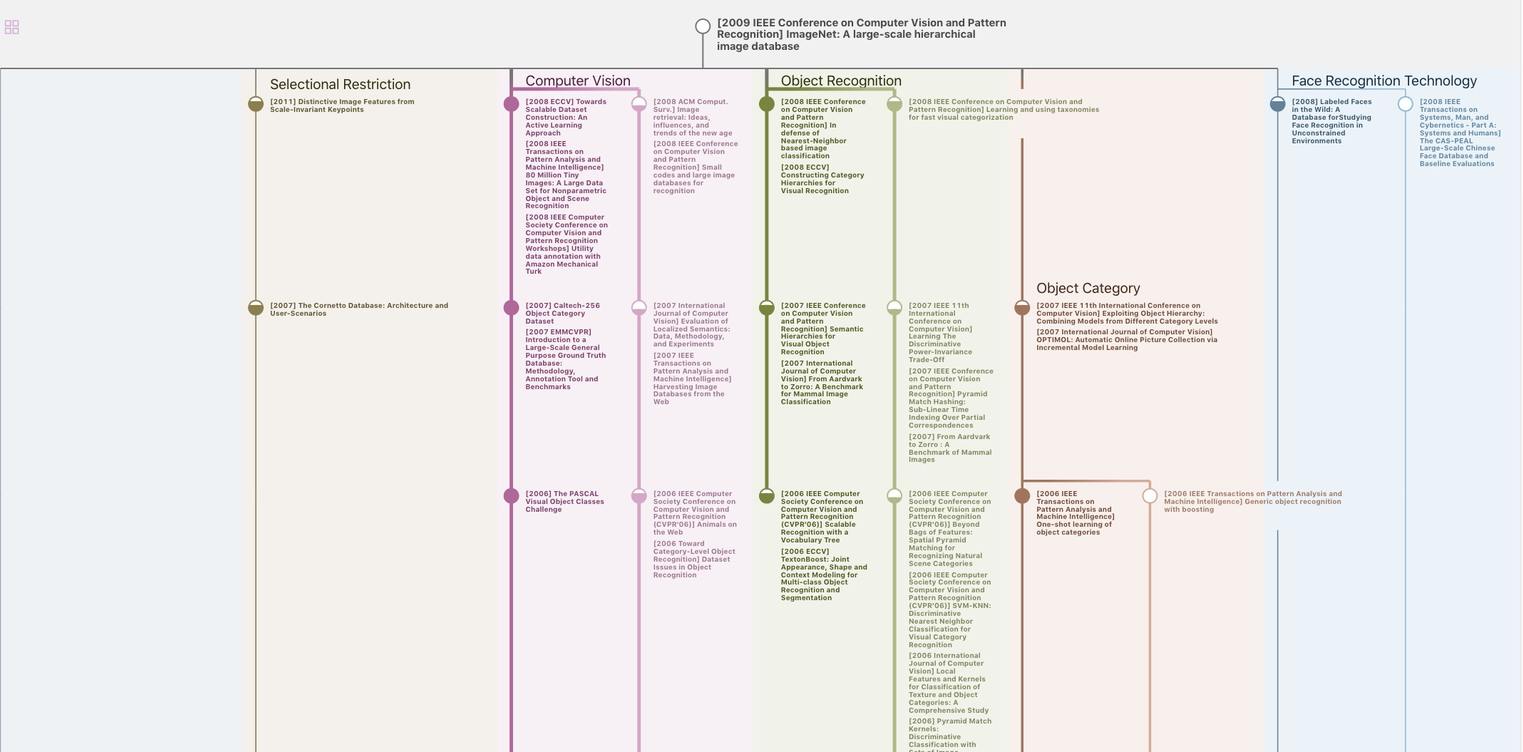
生成溯源树,研究论文发展脉络
Chat Paper
正在生成论文摘要