Systems View to Designing RF Fingerprinting for Real-World Operations.
Wireless Network Security (WISEC)(2022)
摘要
Great progress has been made recently in radio frequency (RF) machine learning (ML), including RF fingerprinting. Much of this work to date, however, has been limited in scope to proof-of-concept demonstrations or narrowly defined and tested under circumstances that only address part of the actual operational considerations. In this paper we expand this consideration for an RF fingerprinting application. In doing so, we build on our previous work developing our RiftNetT deep learning classifier to consider realistic operational and systems aspects to ensure the solution is robust to real-world signal environments and other tasks of an RF receiver. In particular, we show new results on how to handle difficult cases of signal interference, how to efficiently improve performance with the use of geolocation information, and ways to further reduce computational constraints for edge operation. In summary, this work presents moving beyond initial success in RF ML towards expanding the solution for eventual edge operation in a larger system.
更多查看译文
关键词
RF Fingerprint,Deep Learning,Machine Learning,RiftNet,Geolocation,Signal Interference
AI 理解论文
溯源树
样例
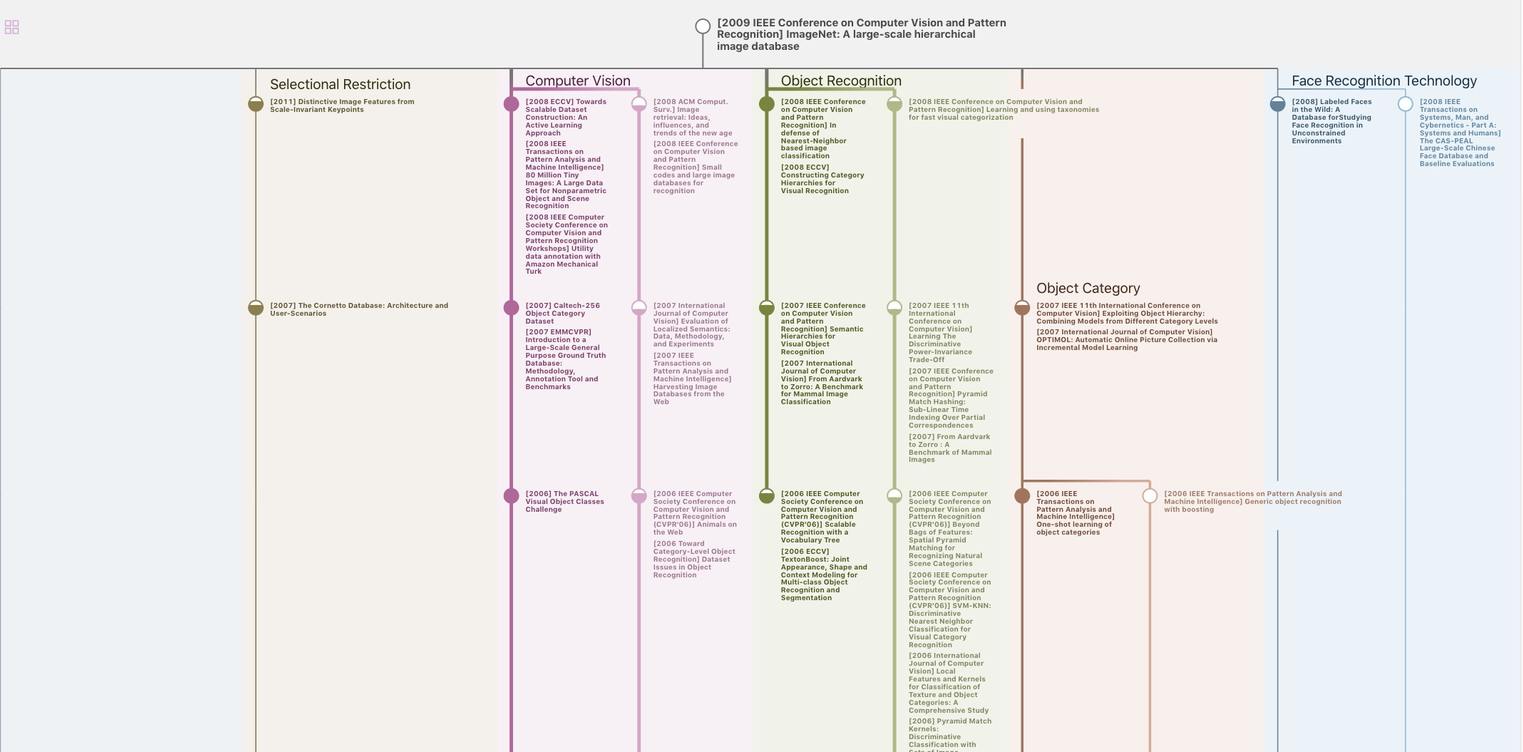
生成溯源树,研究论文发展脉络
Chat Paper
正在生成论文摘要