Comparison of Transformer-Based Models Trained in Turkish and Different Languages on Turkish Natural Language Processing Problems.
Signal Processing and Communications Applications Conference (SIU)(2022)
摘要
Transformer-based pre-trained language models have yielded successful results in natural language processing (NLP) problems in recent years. In these approaches, the models are trained in an unsupervised manner using a large corpus, with the use of mechanisms such as masking and next sentence prediction. In sub-NLP problems, these models are fully or partially updated with a fine-tuning approach, or the vectors obtained from these models are directly mapped to the output with neural network layers added. In this study, transformer-based BERT, RoBERTa, ConvBERT and Electra models, which have given successful results for different languages and problems in the literature, have been applied to Turkish sentiment analysis, text classification and named entity recognition problems, and the results are presented comparatively. One of the contributions of the study is to train the RoBERTa model on a total of 38 GB Turkish corpus to be shared as open source for use in Turkish problems in the field of NLP. Experimental results have shown that the RoBERTaTurk model gives comparable results to the other transformer-based models in Turkish NLP problems. In addition, language models trained in different languages were also tested on Turkish classification problems. According to the obtained results, as the size of the labeled training set increases, the success of the language models trained in different languages becomes close to the success of the language models trained for the Turkish language. It has also been shown that Turkish language models give better results when the number of labeled data is low.
更多查看译文
关键词
different languages,transformer-based
AI 理解论文
溯源树
样例
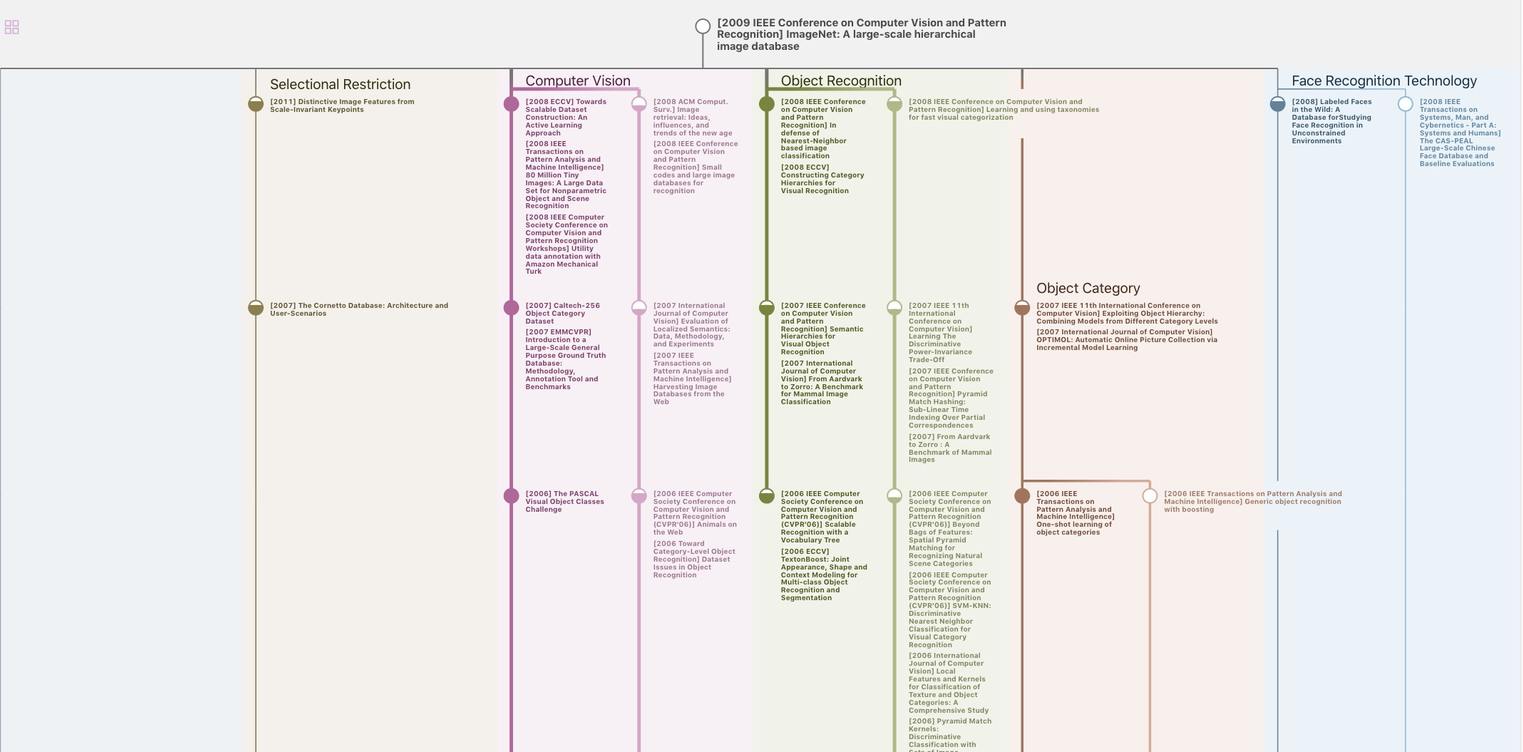
生成溯源树,研究论文发展脉络
Chat Paper
正在生成论文摘要