Exploring the Impact of Gender Bias Mitigation Approaches on a Downstream Classification Task.
International Syposium on Methodologies for Intelligent Systems (ISMIS)(2022)
摘要
Natural language models and systems have been shown to reflect gender bias existing in training data. This bias can impact on the downstream task that machine learning models, built on this training data, are to accomplish. A variety of techniques have been proposed to mitigate gender bias in training data. In this paper we compare different gender bias mitigation approaches on a classification task. We consider mitigation techniques that manipulate the training data itself, including data scrubbing, gender swapping and counterfactual data augmentation approaches. We also look at using de-biased word embeddings in the representation of the training data. We evaluate the effectiveness of the different approaches at reducing the gender bias in the training data and consider the impact on task performance. Our results show that the performance of the classification task is not affected adversely by many of the bias mitigation techniques but we show a significant variation in the effectiveness of the different gender bias mitigation techniques.
更多查看译文
关键词
Gender bias,Training data,Classification
AI 理解论文
溯源树
样例
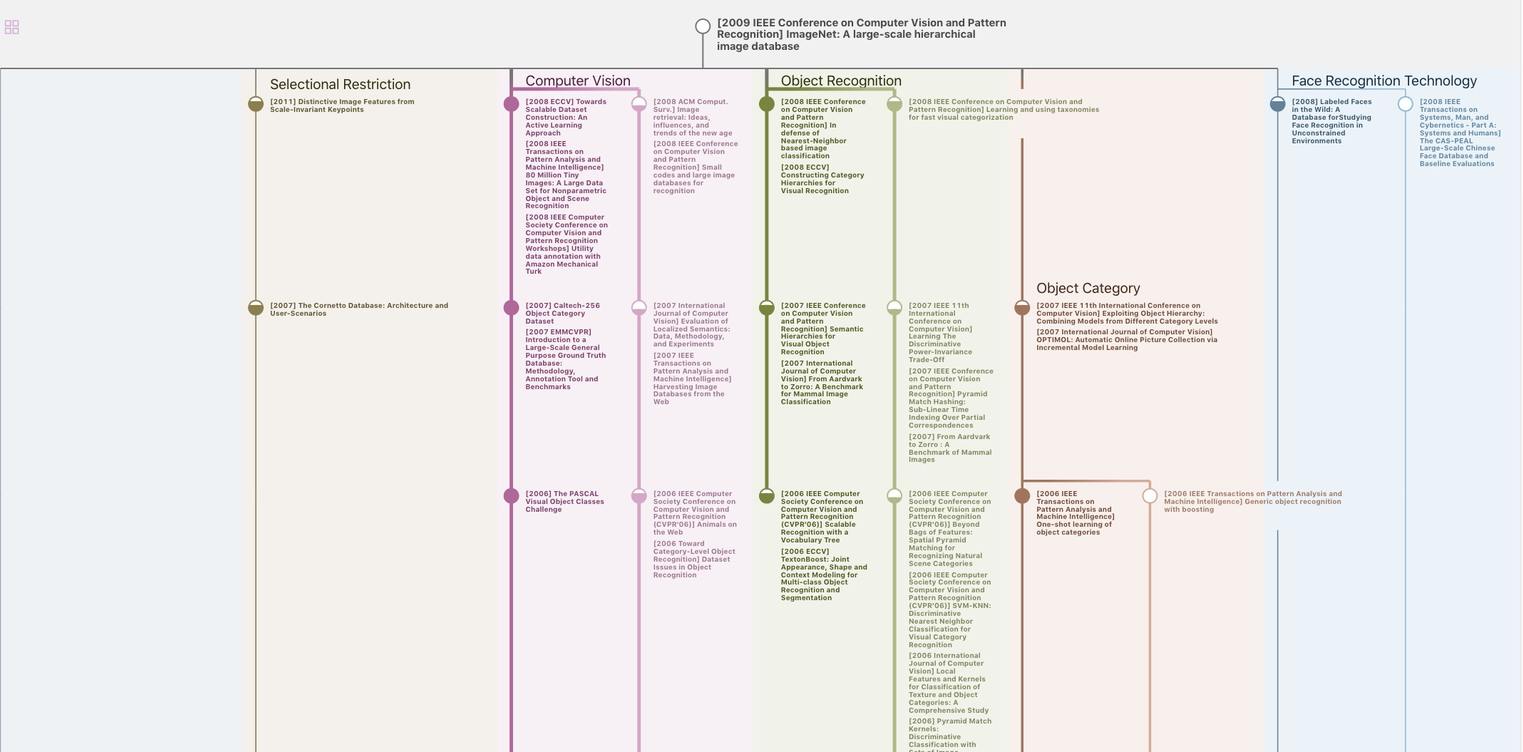
生成溯源树,研究论文发展脉络
Chat Paper
正在生成论文摘要