Sentiment Polarity and Emotion Detection from Tweets Using Distant Supervision and Deep Learning Models.
International Syposium on Methodologies for Intelligent Systems (ISMIS)(2022)
摘要
Automatic text-based sentiment analysis and emotion detection on social media platforms has gained tremendous popularity recently due to its widespread application reach, despite the unavailability of a massive amount of labeled datasets. With social media platforms in the limelight in recent years, it's easier for people to express their opinions and reach a larger target audience via Twitter and Facebook. Large tweet postings provide researchers with much data to train deep learning models for analysis and predictions for various applications. However, deep learning-based supervised learning is data-hungry and relies heavily on abundant labeled data, which remains a challenge. To address this issue, we have created a large-scale labeled emotion dataset of 1.83 million tweets by harnessing emotion-indicative emojis available in tweets. We conducted a set of experiments on our distant-supervised labeled dataset using conventional machine learning and deep learning models for estimating sentiment polarity and multi-class emotion detection. Our experimental results revealed that deep neural networks such as BiLSTM and CNN-BiLSTM outperform other models in both sentiment polarity and multi-class emotion classification tasks achieving an F1 score of 62.21% and 39.46%, respectively, an average performance improvement of nearly 2-3 percentage points on the baseline results.
更多查看译文
关键词
Sentiment polarity,Emotion detection,Distant supervision,Emoji,Deep learning,Twitter,Classification
AI 理解论文
溯源树
样例
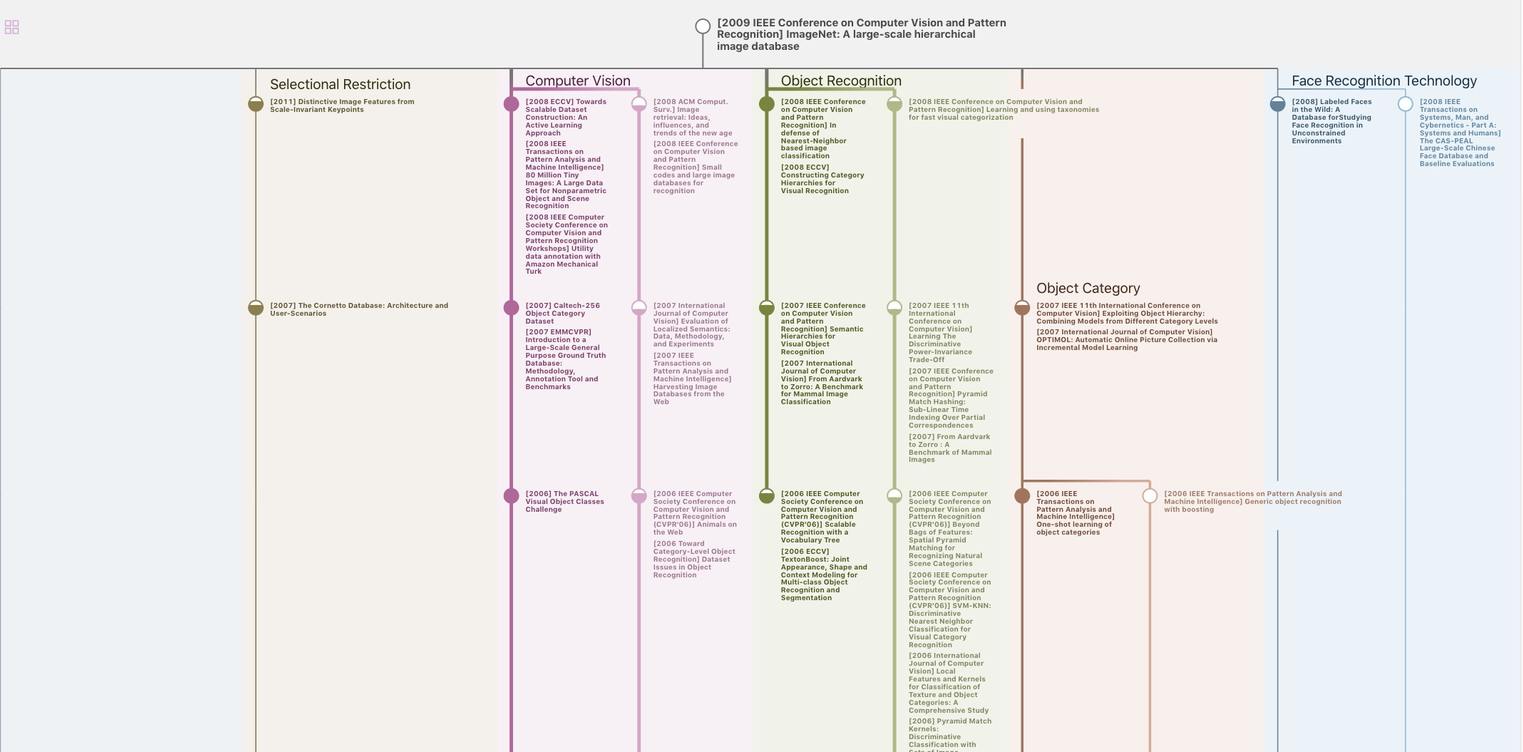
生成溯源树,研究论文发展脉络
Chat Paper
正在生成论文摘要