Cardiac Arrhythmia Classification from 12-lead Electrocardiogram Using a Combination of Deep Learning Approaches.
International Convention on Information and Communication Technology, Electronics and Microelectronics (MIPRO)(2022)
摘要
Traditionally, electrocardiogram (ECG) signals are recorded and monitored over a period of time and finally analyzed by an expert. Automatic classification of cardiac arrhythmias has the potential to improve diagnostics. In this work, we explore the use of representation learning from ECG signals for cardiac arrhythmia classification. The dataset consisting of five cardiac rhythm types was created from the CPSC, CPSC-Extra, and The Georgia 12-lead ECG Challenge databases. We use a sophisticated deep learning approach for representation learning and classification, namely a combination of a Convolutional Auto-Encoder (CAE) and a Long Short-Term Memory (LSTM) classifier. CAE was used to compress the input signal that serves as input to the LSTM classifier. We also implemented a CAE-based data augmentation approach to balance the data distribution. The classification results reaching above 90% accuracy show that the use of the complex deep learning approach is suitable for addressing the problem.
更多查看译文
关键词
arrhythmia classification,ECG,deep learning,convolutional autoencoder,LSTM,data augmentation
AI 理解论文
溯源树
样例
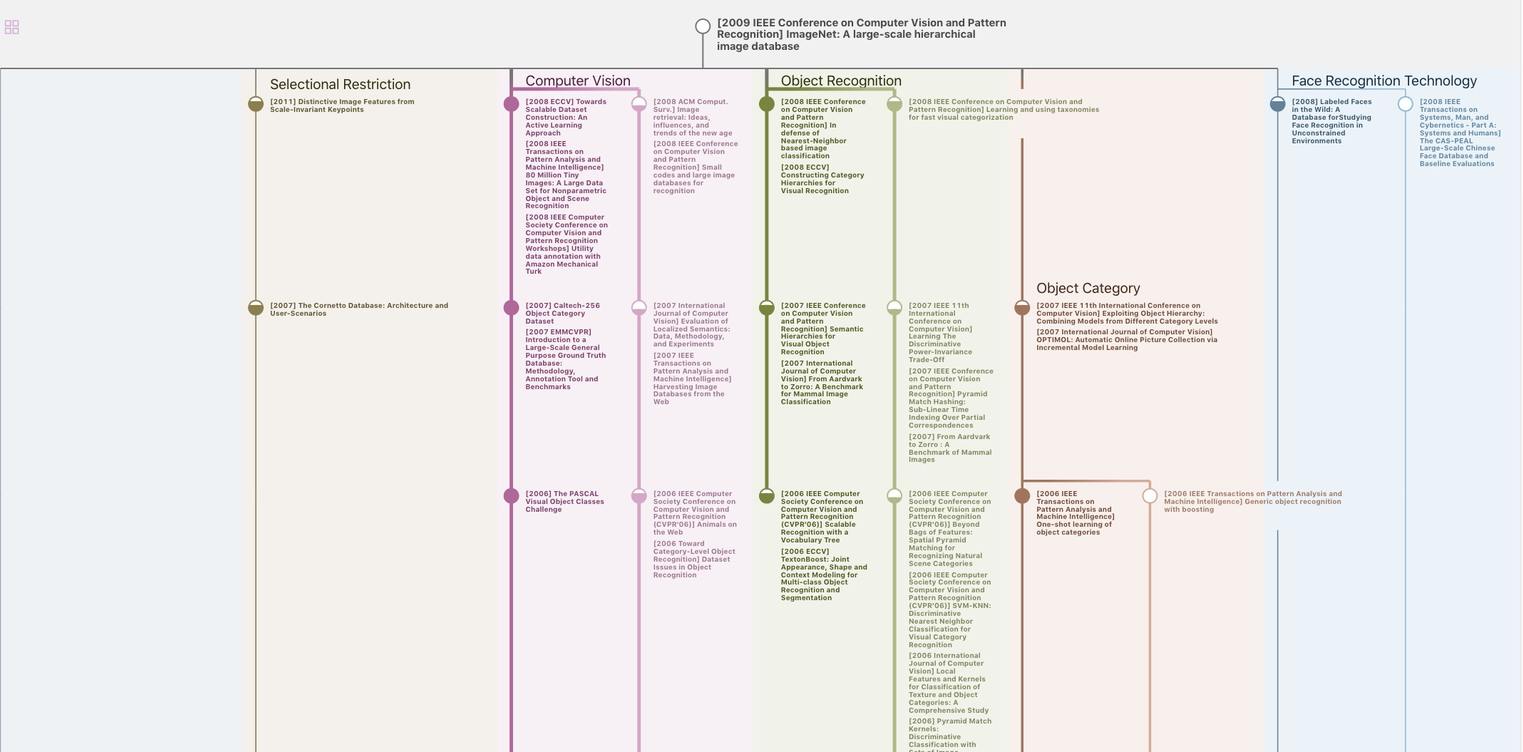
生成溯源树,研究论文发展脉络
Chat Paper
正在生成论文摘要