Learning from Imbalanced Data Using an Evidential Undersampling-Based Ensemble.
International Conference on Scalable Uncertainty Management (SUM)(2022)
摘要
In many real-world binary classification problems, one class tends to be heavily underrepresented when it consists of far fewer observations than the other class. This results in creating a biased model with undesirable performance. Different techniques, such as undersampling, have been proposed to fix this issue. Ensemble methods have also been proven to be a good strategy to improve the performance of the resulting model in the case of class imbalance. In this paper, we propose an evidential undersampling-based ensemble approach. To alleviate the issue of losing important data, our undersampling technique assigns soft evidential labels to each majority instance, which are later used to discard only the unwanted observations, such as noisy and ambiguous examples. Finally, to improve the final results, the proposed undersampling approach is incorporated into an evidential classifier fusion-based ensemble. The comparative study against well-known ensemble methods reveal that our method is efficient according to the G-Mean and F-Score measures.
更多查看译文
关键词
imbalanced data,learning,undersampling-based
AI 理解论文
溯源树
样例
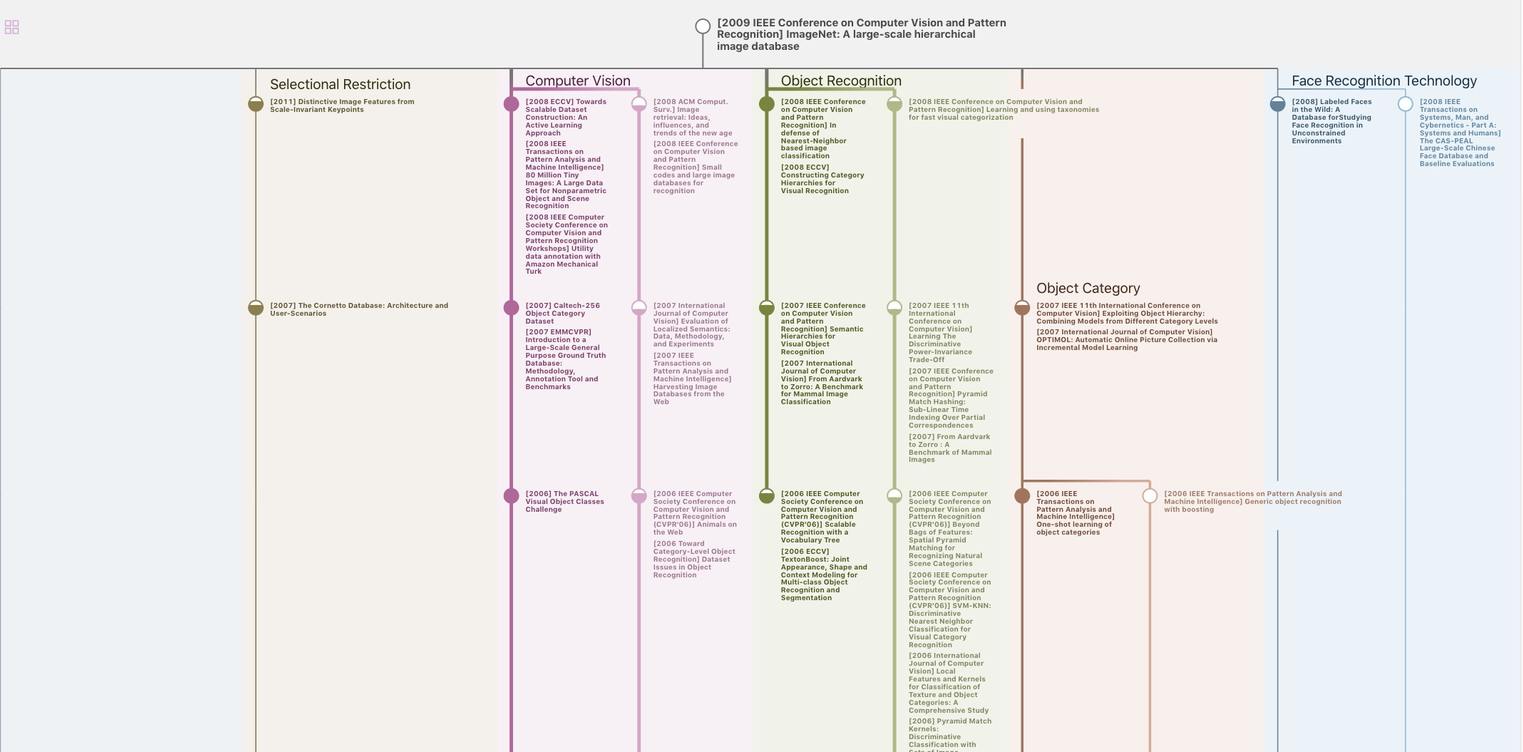
生成溯源树,研究论文发展脉络
Chat Paper
正在生成论文摘要