Effects of KNN Neighbor's Density and Distance on Forest-Stand-Level AGB Estimation
IEEE International Geoscience and Remote Sensing Symposium (IGARSS)(2022)
摘要
k nearest neighbor (kNN) algorithm, a non-parametric and one of the machine learning techniques had been popularly used for stand-level AGB estimation. kNN algorithm has two distinct parameters which are concerning the distance and the number of nearest neighbors (k) and the distance of target plots with reference plots. This study was conducted to assess the effects of the number of k, reference plots density, and distance of target plots with reference plots on the performance of the kNN algorithm in estimating stand-level AGB information. Using the surface reflectance of the Sentinel-2 image, the effects of the number of k (3, 5, 8, 11), reference plots density (high=3932 pixels, low=656 pixels), and the distance of the target and reference plots (2km, 5km, and 10km) to the prediction error of the kNN algorithm were assessed. The performance of the kNN was assessed using the RMSE and RMSPE as evaluation criteria. The 3-way factorial ANOVA was used to determine the main and interaction effects of the variables. The results showed that the number of k, reference plot density, and the distance of target plots with reference plots have a significant effect on the prediction error of the kNN algorithm. The PRMSE increases with the increasing distance and decreased with the increased number of k. Further, the PRMSE was low when the reference plot density was high. The PRMSE can be improved by as much as 13% when the reference plot density is sufficient and when an optimal number of k and distance are used for the parameters.
更多查看译文
关键词
non-parametric machine learning model, parameter adjustments, surface reflectance, optical satellite image
AI 理解论文
溯源树
样例
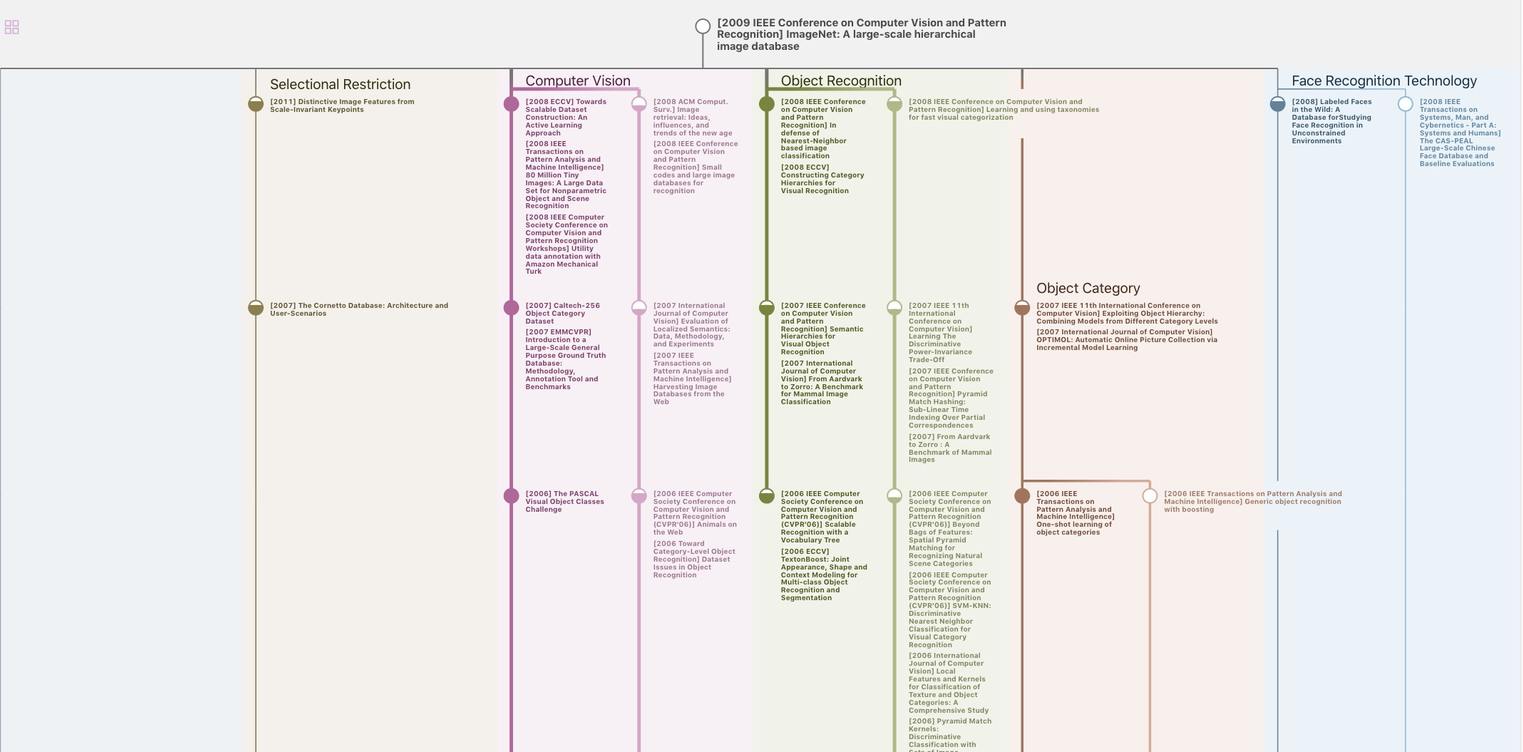
生成溯源树,研究论文发展脉络
Chat Paper
正在生成论文摘要