Spectral Super-Resolution for Hyperspectral Image Reconstruction Using Dictionary and Machine Learning.
IEEE International Geoscience and Remote Sensing Symposium (IGARSS)(2022)
摘要
Hyperspectral sensors measure the radiance spectrum across hundreds of wavelength channels with a resolution typically on the order of 10 nm represented by the full-width-half-maximum (FWHM). The spectra are used in the study of surface materials in the biological, geological and oceanographic sciences to name a few, utilizing quantitative spectroscopic techniques. The instruments developed to measure such data are expensive due to the increased number of bands, and create large datasets that can be difficult to downlink for a given instance. Repeat cycle of space-borne hyperspectral observations of the earth surface is also less than those of multi-spectral sensors. It becomes incumbent to develop mechanisms that could be cost-effective and give desired results. With this aim, spectral Super-Resolution (SR) is attempted on the Airborne Visible and InfraRed Imaging Spectrometer (AVIRIS) data to reconstruct the hyperspectral band radiance from equally-spaced narrow multi-spectral bands using dictionary learning, followed by denoising using machine learning. The hyperspectral band radiance are first estimated from 30 selected input multi-spectral bands using dictionary trained through K-Singular Value Decomposition (K-SVD), followed by denoising using Random Forest Regression. An overall Signal-to-Noise Ratio (SNR) of 31.58dB is observed from reconstruction after denoising using Random Forest.
更多查看译文
关键词
Spectral Super-Resolution, Signal and Image Processing, Image Reconstruction, Dictionary Learning
AI 理解论文
溯源树
样例
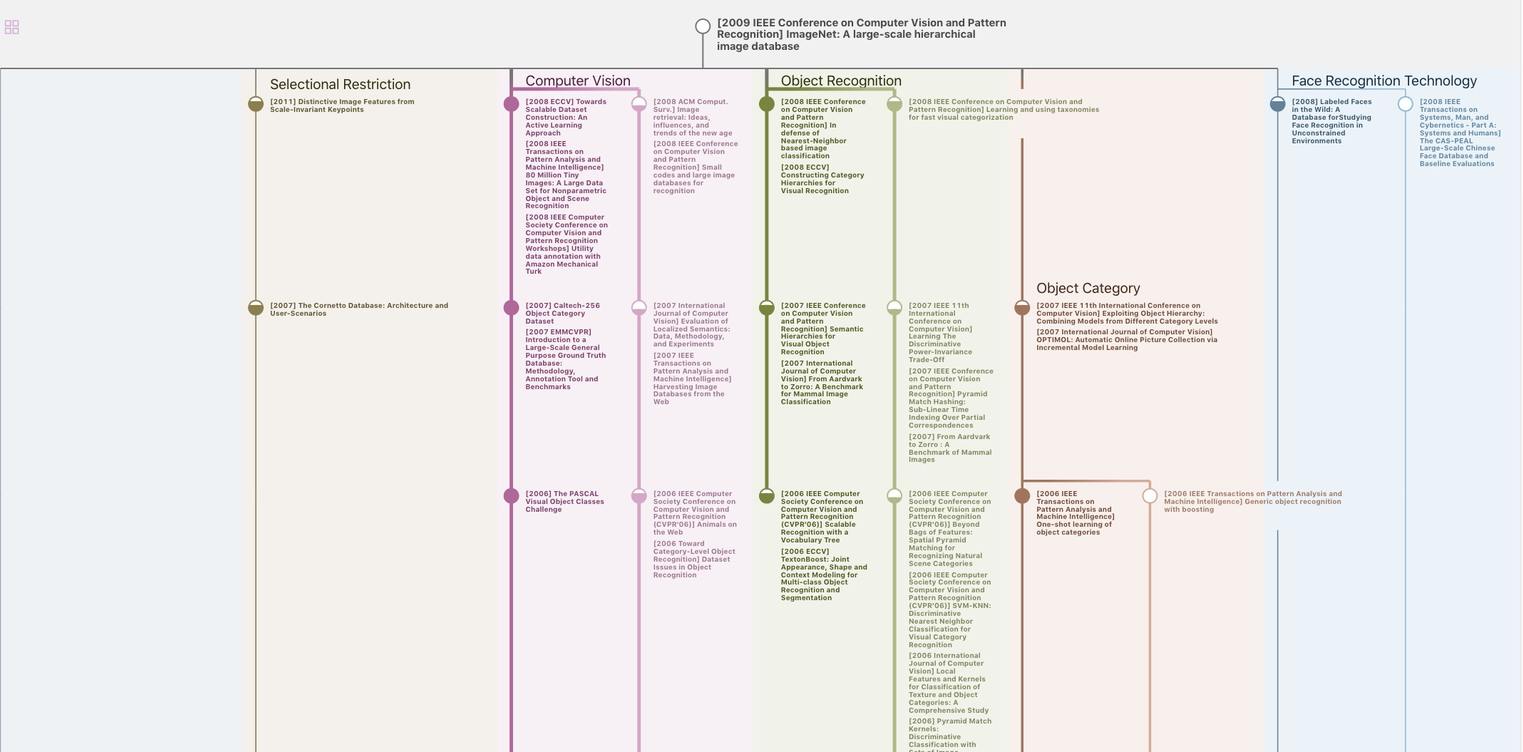
生成溯源树,研究论文发展脉络
Chat Paper
正在生成论文摘要