Learning Uncertainty-Aware Label Transition for Weakly Supervised Solar Panel Mapping with Aerial Images.
IEEE International Geoscience and Remote Sensing Symposium (IGARSS)(2022)
摘要
Weakly supervised solar panel mapping has shown its advantages in automatically detecting solar panels from remote sensing images with low annotation costs. Considering the noisy nature of pseudo labels (PLs), which are frequently employed in weakly supervised methods, we propose to introduce uncertainty measure to guide the estimation of noise levels in PLs and develop a novel method based on uncertainty-aware label transition (UALT). The proposed method consists of three parts: uncertainty estimation network, uncertaintyaware label transition network, and target mapping network with forward correction. We first generate heteroscedastic uncertainty by learning an estimator under Bayes formalism. Then, with the uncertainty as guidance, a label transition network is trained to learn the mapping between clean labels, and Bayes optimal labels and predict the instance-dependent transition matrix. Finally, the transition matrix is employed in the forward correction process, where the target mapping network produces clean predictions for solar panels. Comparative experiments with six state-of-the-art weakly supervised methods on an aerial image data set show the superiority of the proposed UALT, especially in mapping accuracy and discovering small-scale objects.
更多查看译文
关键词
Solar panel mapping,weakly supervised learning,uncertainty estimation,label transition
AI 理解论文
溯源树
样例
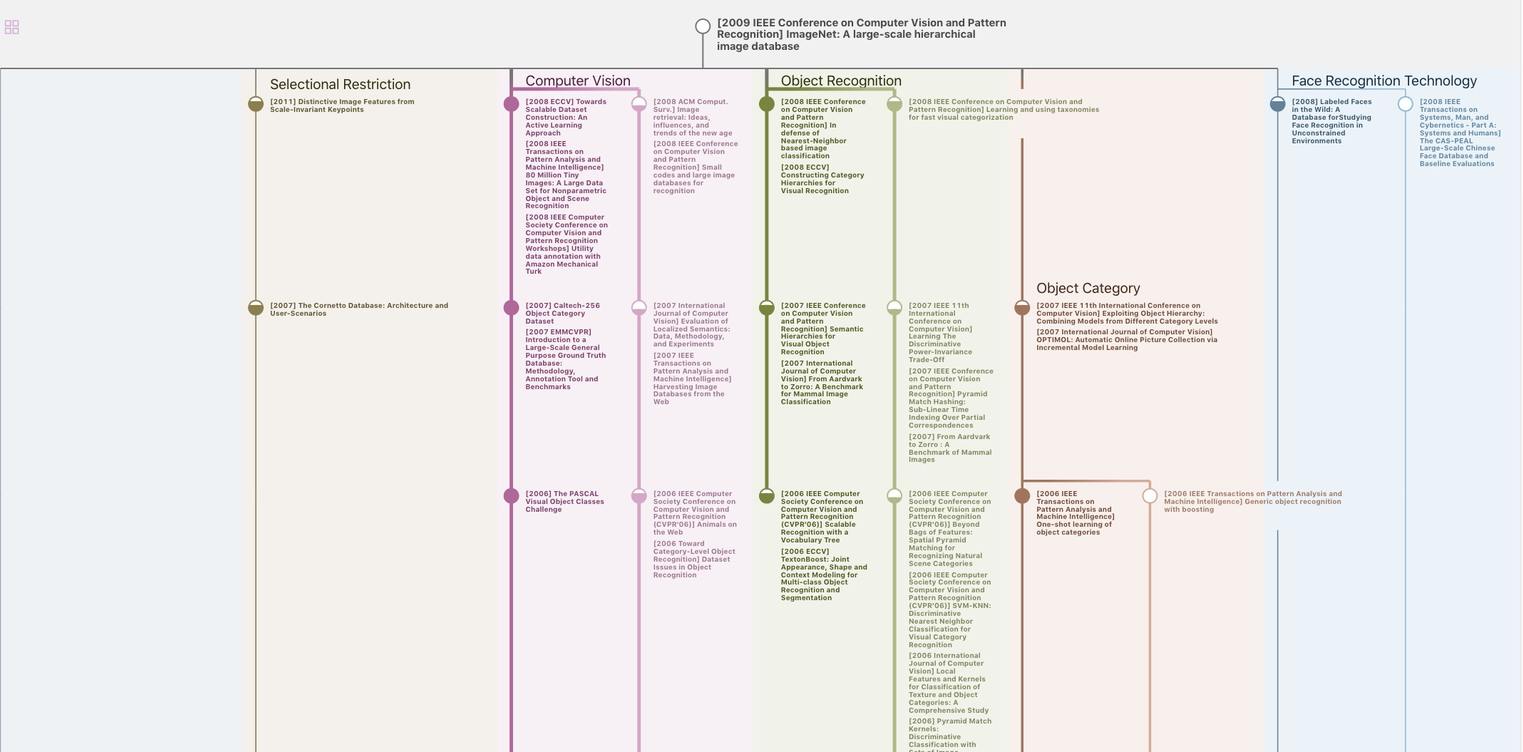
生成溯源树,研究论文发展脉络
Chat Paper
正在生成论文摘要