Faster Ship Detection Algorithm in Large-Scene SAR Images.
IEEE International Geoscience and Remote Sensing Symposium (IGARSS)(2022)
摘要
In recent years, deep learning has achieved great accuracy in SAR image ship detection tasks. However, for large-scene SAR images, the sliding window method will generate a large number of slices sent to the network, resulting in a waste of GPU resources. To solve this problem, we proposed a ship detection algorithm based on CFAR, which significantly reduces the number of slices and false alarms on land. Firstly, the sea regions to be detected are obtained under the prior guidance of Global Self-consistent Hierarchical High-resolution Shorelines(GSHHS), and remove major land areas in the SAR image. Secondly, the ship candidate region generation algorithm based on block G0-CFAR is implemented to obtain the candidate slices. Finally, the Yolov5 model is used to detect ships from the candidate slices. The validation experiment of sentinel-1 and Gaofen-3 largescene SAR images shows that the proposed method can detect the ships in large-scene SAR images with 88% detection accuracy within 97s, and the number of image slices fed into GPU is greatly reduced. It has certain practical significance for ship detection in large-scene SAR images.
更多查看译文
关键词
Ship Detection,CFAR,Yolo,Large-Scene,Synthetic Aperture Radar
AI 理解论文
溯源树
样例
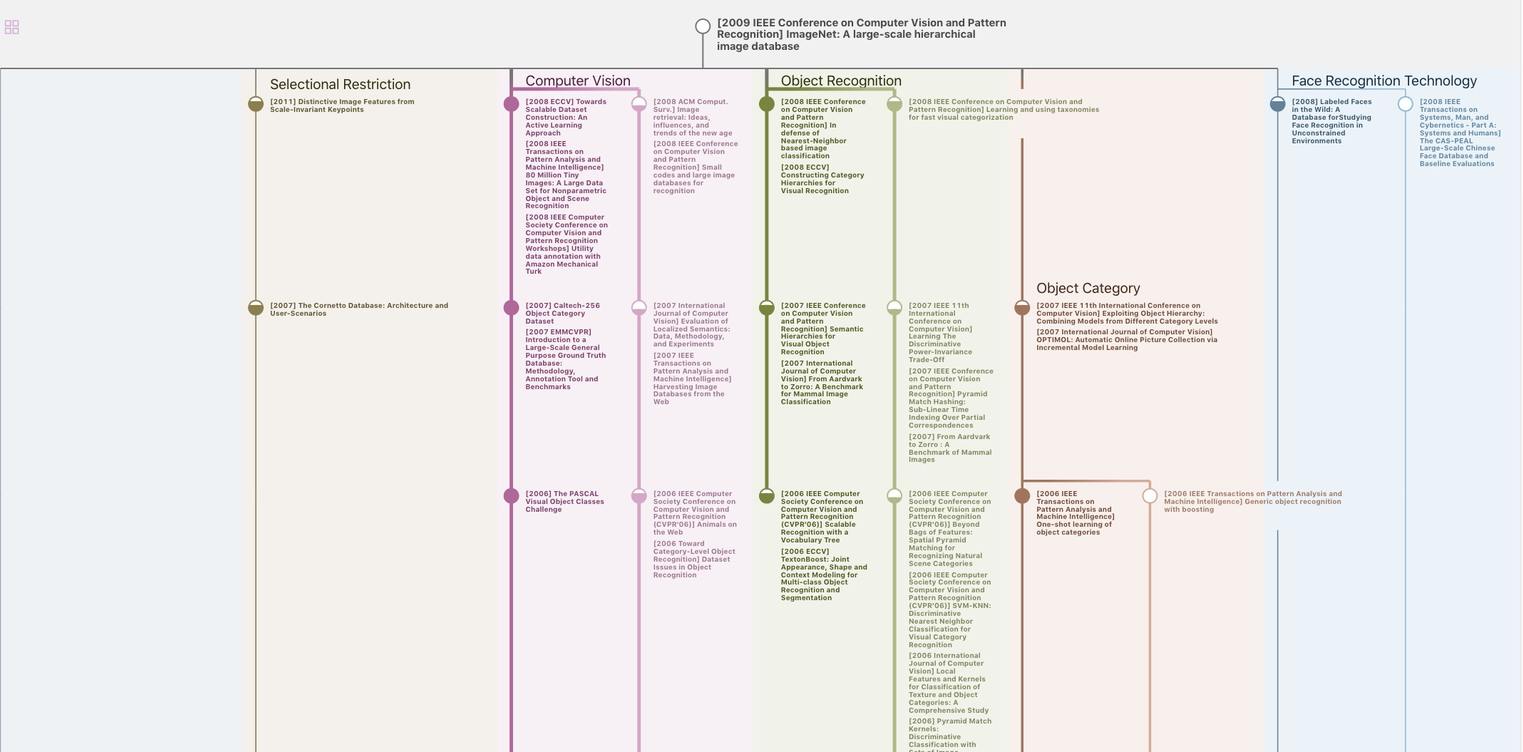
生成溯源树,研究论文发展脉络
Chat Paper
正在生成论文摘要