Efficient Bayesian Learning of Sparse Deep Artificial Neural Networks.
International Symposium on Intelligent Data Analysis (IDA)(2022)
摘要
In supervised Machine Learning (ML), Artificial Neural Networks (ANN) are commonly utilized to analyze signals or images for a variety of applications. They are increasingly performing as a strong tool to establish the relationships among data and being successfully applied in science due to their generalization ability, noise and fault tolerance. One of the most difficult aspects of using the learning process is optimization of the network weights. A gradient-based technique with a back-propagation strategy is commonly used for this optimization stage. Regularization is commonly employed for the benefit of efficiency. This optimization gets difficult when non-smooth regularizers are applied, especially to promote sparse networks. Due to differentiability difficulties, traditional gradient-based optimizers cannot be employed. In this paper, we propose an MCMC-based optimization strategy within a Bayesian framework. An effective sampling strategy is designed using Hamiltonian dynamics. The suggested strategy appears to be effective in allowing ANNs with modest complexity levels to achieve high accuracy rates, as seen by promising findings.
更多查看译文
关键词
efficient bayesian learning,artificial neural networks,neural networks
AI 理解论文
溯源树
样例
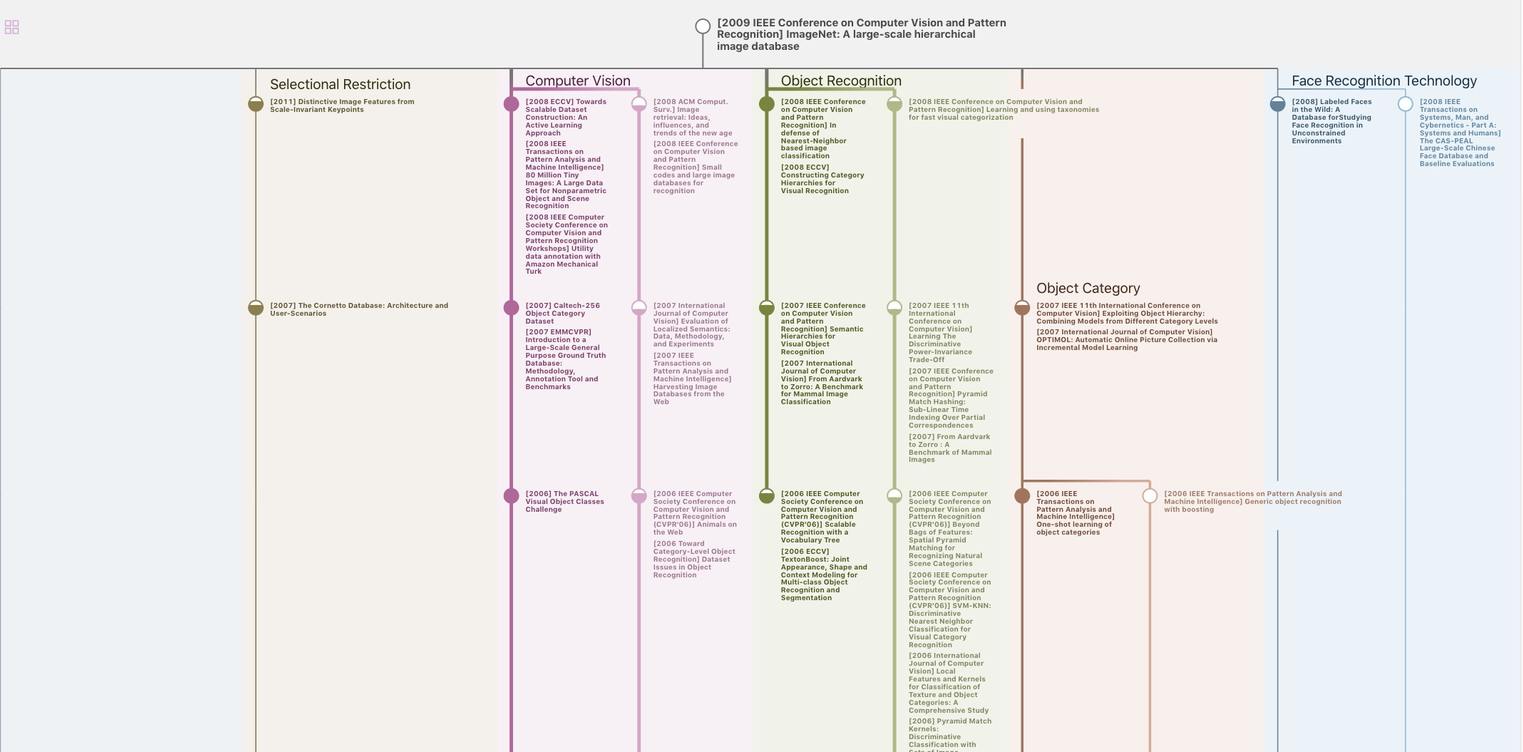
生成溯源树,研究论文发展脉络
Chat Paper
正在生成论文摘要