Think Fast: Time Control in Varying Paradigms of Spiking Neural Networks
International Conference on Neuromorphic Systems (ICONS)(2022)
摘要
The state-of-the-art in machine learning has been achieved primarily by deep learning artificial neural networks. These networks are powerful but biologically implausible and energy intensive. In parallel, a new paradigm of neural network is being researched that can alleviate some of the computational and energy issues. These networks, spiking neural networks (SNNs), have transformative potential if the community is able to bridge the gap between deep learning and SNNs. However, SNNs are notoriously difficult to train and lack precision in their communication. In an effort to overcome these limitations and retain the benefits of the learning process in deep learning, we investigate novel ways to translate between them. We construct several network designs with varying degrees of biological plausibility. We then test our designs on an image classification task and demonstrate our designs allow for a customized tradeoff between biological plausibility, power efficiency, inference time, and accuracy.
更多查看译文
关键词
neuro-inspired artificial intelligence, machine learning, neuromorphic computing
AI 理解论文
溯源树
样例
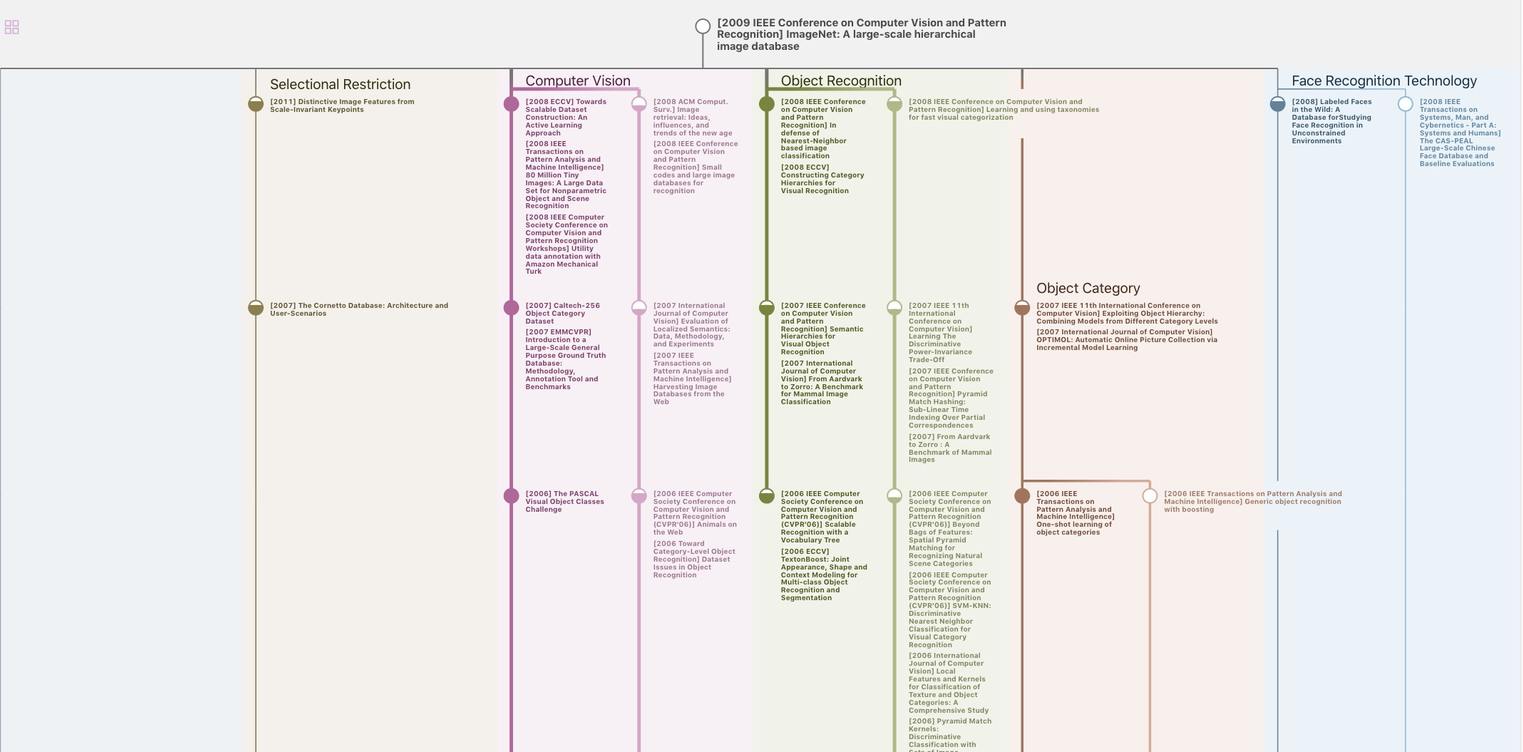
生成溯源树,研究论文发展脉络
Chat Paper
正在生成论文摘要