Apples-to-spikes: The first detailed comparison of LASSO solutions generated by a spiking neuromorphic processor
International Conference on Neuromorphic Systems (ICONS)(2022)
摘要
The Locally Competitive Algorithm (LCA) is a model of simple cells in the primary visual cortex, based on convex sparse coding via recurrent lateral competition between neighboring neurons. Previous work implemented spiking LCA (S-LCA) on the Loihi neuromorphic processor in which the lateral connections were constrained to be inhibitory, unlike non-spiking, analog LCA (A-LCA) where both excitatory and inhibitory connections are present. In the absence of lateral excitation, an implementation of S-LCA on the Loihi neuromorphic processor inferred sparse representations of image patches that were close to the global minimum, but an examination of the individual neural activations (i.e. solution) was not performed. In this work, we first prove that the constraints placed on the lateral connections in the previous S-LCA implementation were unnecessarily restrictive, and we develop an S-LCA implementation with both excitatory and inhibitory lateral connections. We implemented this improved S-LCA with both inhibitory and excitatory lateral connections on Loihi and show that the resulting sparse latent representations were much closer to those inferred by A-LCA. Specifically, we perform the first comparison of individual neuron activations between S-LCA and A-LCA and show that the final solution of our S-LCA converges to that of A-LCA. To date, this work provides one of the only instances in which a spiking algorithm implemented on modern neuromorphic hardware and performing a realistic task has exhibited such close behavior to its non-spiking counterpart.
更多查看译文
关键词
neuromorphic computing, sparse coding, computer vision, spiking neural networks
AI 理解论文
溯源树
样例
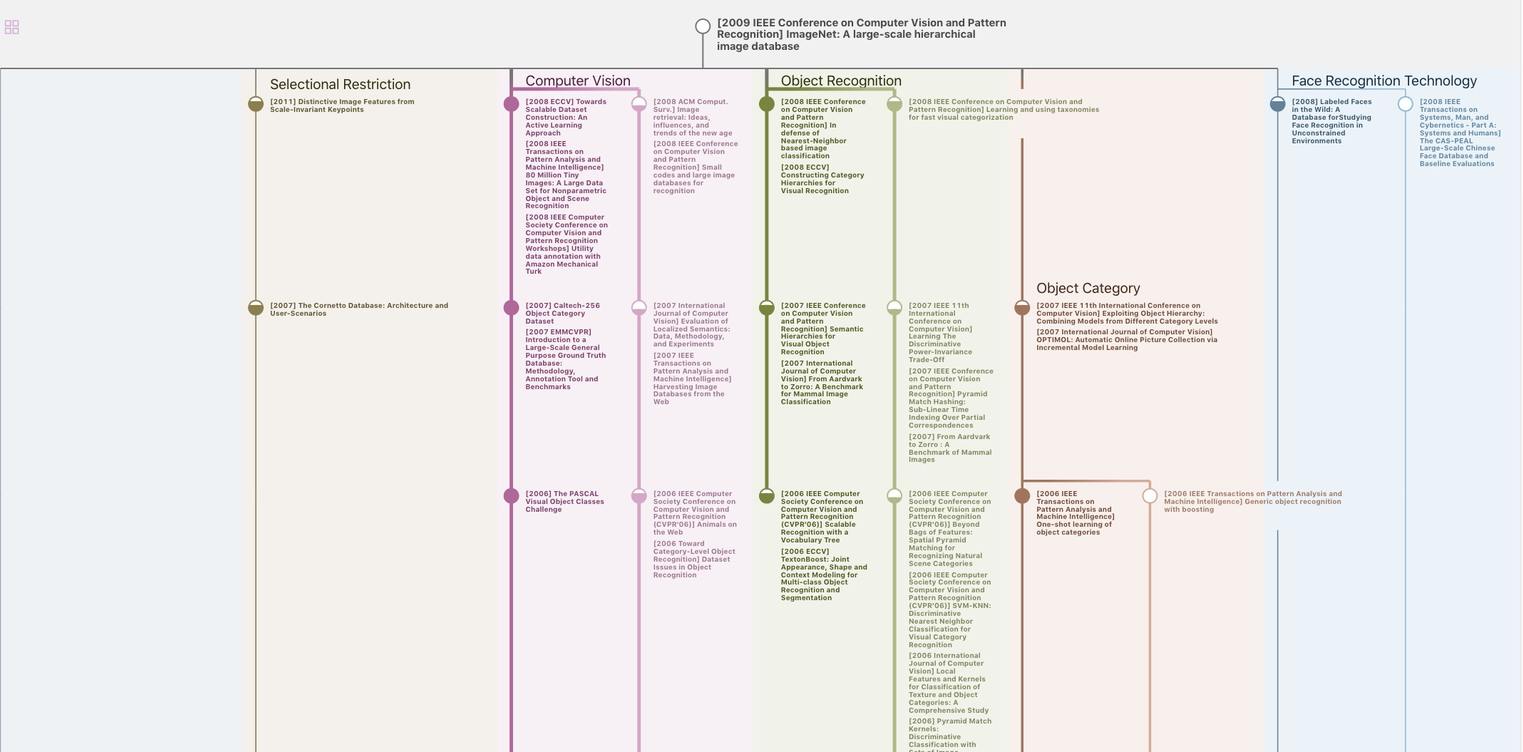
生成溯源树,研究论文发展脉络
Chat Paper
正在生成论文摘要