Combining traditional machine learning and anomaly detection for several imbalanced Android malware dataset's classification.
International Conference on Machine Learning Technologies (ICMLT)(2022)
摘要
As the number of mobile devices has exploded in recent years, so has the amount of advanced Android malware. Among the popular Android malware on the market today, click fraud malware, adware, banking Trojans, spyware, etc. are usually disguised and hidden in the heap of good Android applications. These advanced malwares lurk in the third-party application market trusted by users, and potentially endanger the security of the user's smart device causing privacy or economic loss. Therefore, this paper leverages and combines traditional machine learning and anomaly detection methods to detect specific classes of Android malware in three highly imbalanced Android datasets (entertainment + social app vs. click fraud malware + adware; financial services app vs. banking trojan; communication app vs. spyware). The experiment results show that our proposed combined methods have great performance on the three sub-datasets, achieving the average f1-score over 0.98 on three imbalanced datasets, which performs better than the traditional machine learning algorithm used alone. In addition, we use the combined methods to analyze the correlation between the top features of the dataset, and provide interpretable insights for other researchers focusing on Android malware classification in the coming future.
更多查看译文
关键词
Android malware, machine learning, data mining, static analysis, mobile security
AI 理解论文
溯源树
样例
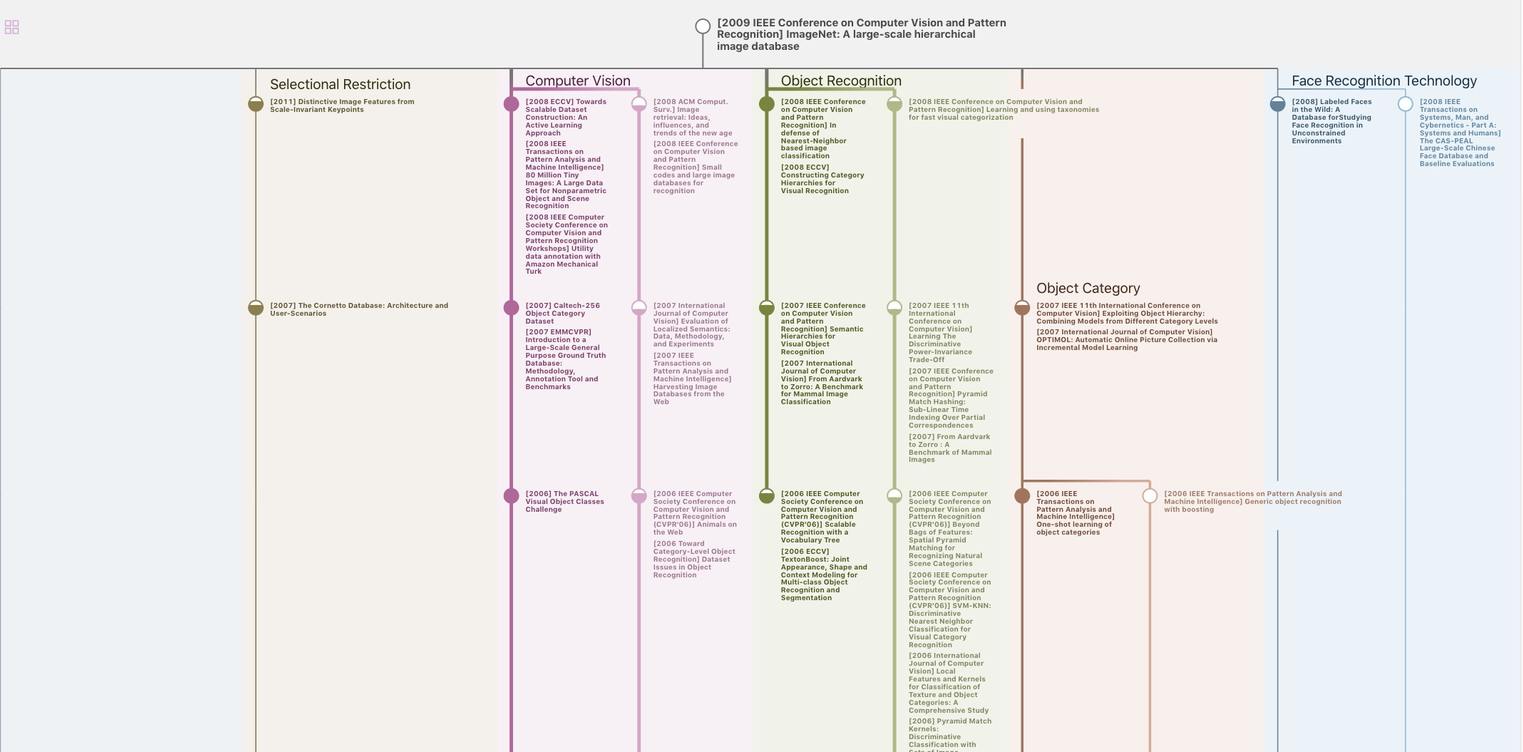
生成溯源树,研究论文发展脉络
Chat Paper
正在生成论文摘要