Online QoS estimation for vehicular radio environments
European Signal Processing Conference (EUSIPCO)(2022)
摘要
Quality of service (QoS) estimation is a key enabler in wireless networks. This has been facilitated by the increasing capabilities of machine learning (ML). However, ML algorithms often underperform when presented with non-stationary data, which is typically the case for radio environments. In such environments, ML schemes might require extra signaling for retraining. In this paper, we propose an approach to online QoS estimation, where a trained model can be taken as a base estimator and fine-tuned with information from the user equipment (UE) and the cell itself. The proposed approach is based on the Adaptive Random Forest (ARF) algorithm, which uses streaming data and reacts on changes under concept drift, i.e., to changes in the data's statistical properties. This effectively allows to retrain parts of the ML model as vehicular UEs visit diverse radio environments. We evaluate this method with real data from an extensive measurement campaign in a cellular test network that covered diverse radio environments.
更多查看译文
关键词
vehicular radio environments,qos,estimation
AI 理解论文
溯源树
样例
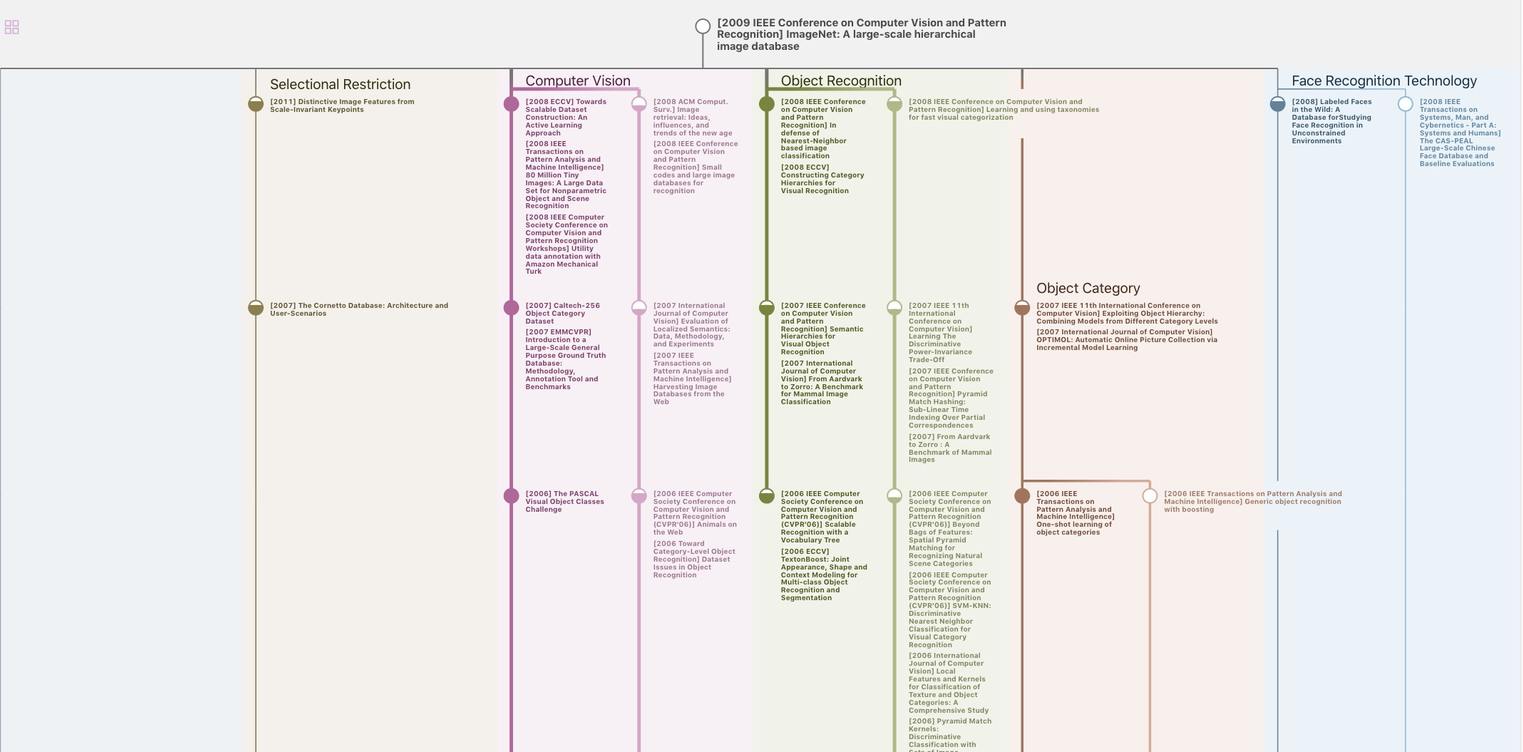
生成溯源树,研究论文发展脉络
Chat Paper
正在生成论文摘要