Conditional Variational Graph Autoencoder for Air Quality Forecasting
European Signal Processing Conference (EUSIPCO)(2022)
摘要
To control air pollution and mitigate its negative effect on health, it is of the utmost importance to have accurate real-time forecasting models. Existing deep-learning-based air quality forecasting models typically deploy temporal and-less often-spatial modules. Yet, data scarcity emerges as a real issue in this domain, a problem that can be solved by capturing the data distribution. In this work, we address data scarcity by proposing a novel conditional variational graph autoencoder. Our model is able to forecast air pollution by efficiently encoding the spatio-temporal correlations of the known data. Additionally, we leverage dynamic context data such as weather or satellite images to condition the model's behaviour. We formulate the problem as a context-aware graph-based matrix completion task and utilize street-level data from mobile stations. Experiments on real-world air quality datasets show the improved performance of our model with respect to state-of-the-art approaches.
更多查看译文
关键词
Air quality forecasting, conditional variational graph autoencoders, context-aware graph-based matrix completion, deep learning
AI 理解论文
溯源树
样例
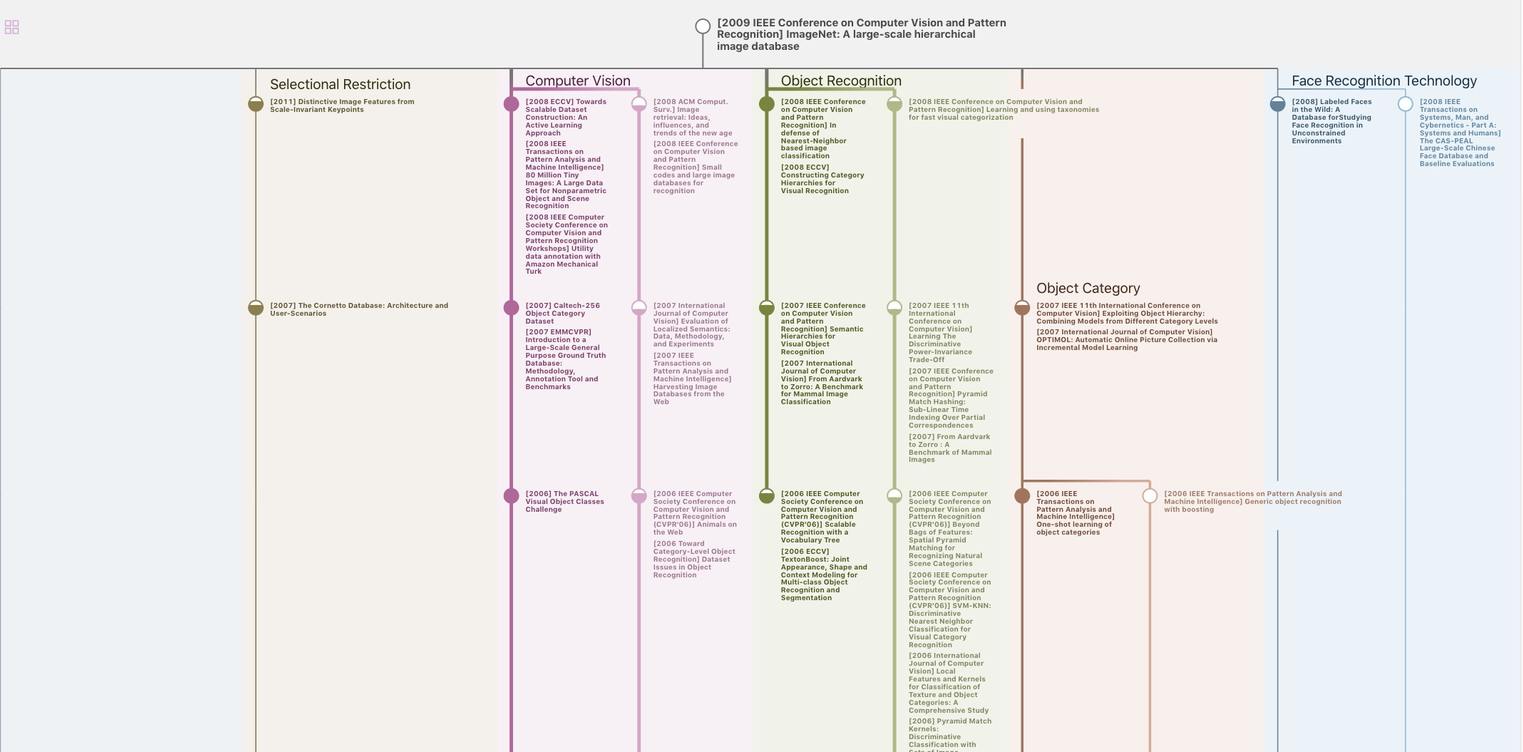
生成溯源树,研究论文发展脉络
Chat Paper
正在生成论文摘要