Implementing a Metadata Manager for Machine Learning with the Asset Administration Shell.
IEEE International Conference on Emerging Technologies and Factory Automation (ETFA)(2022)
摘要
With the rise of Industry 4.0, businesses are increasingly turning to Machine Learning to leverage data for improving quality and productivity. However, one open challenge when embracing Machine Learning in this context is the integration of cloud infrastructures, as well as the heterogeneity of data, interfaces, and protocols in the production environment. To address this, we are developing a framework that aims to simplify the adoption of Machine Learning techniques for heterogeneous industrial automation systems. One of the core features of this framework is the ability to handle data about production devices - a scenario that is naturally suited to the use of Asset Administration Shells. However, the implementation of a system that uses Asset Administration Shells comes with its own set of challenges, such as the abstraction of details from users and the representation of device topologies. Thus, this paper introduces the concepts and implementation of a Metadata Manager component in the aforementioned framework that uses Asset Administration Shells as its basis. We further examine the Metadata Manager's current structure with unit testing, derive planned extensions, and discuss future directions from the Industry 4.0 perspective.
更多查看译文
关键词
Asset Administration Shell, BaSyx, AASX Package Explorer, production plant metadata, digital twin
AI 理解论文
溯源树
样例
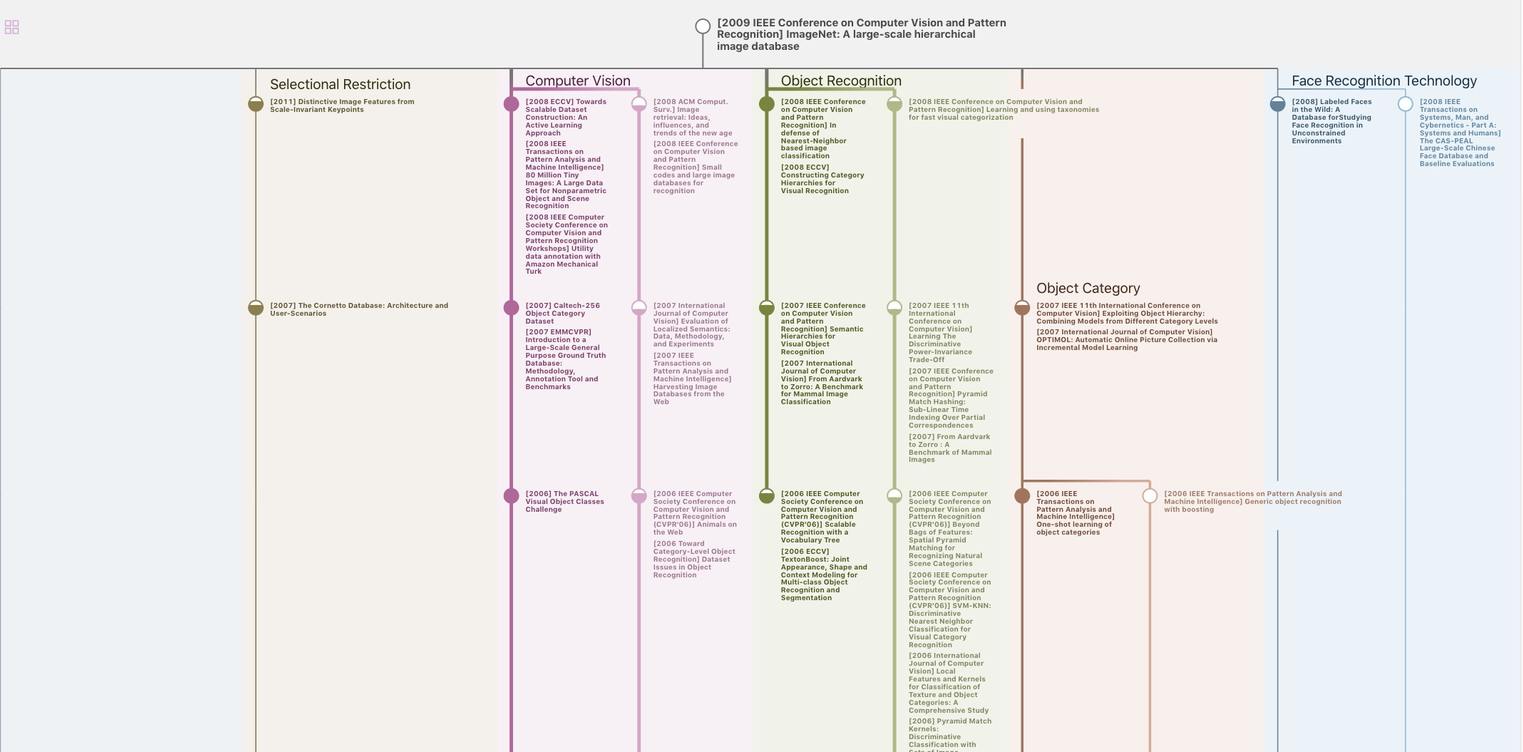
生成溯源树,研究论文发展脉络
Chat Paper
正在生成论文摘要