Fast Feature Extraction from Large Scale Connectome Data Sets Using Zero Crossing Counts Over Graphs.
European Signal Processing Conference (EUSIPCO)(2022)
摘要
Large scale connectome data sets based on sophisticated fMRI recordings are becoming prominent in the advent of big-data-in-neuroscience era. In this work, we present a fast and efficient connectome analysis method for feature extraction using randomly generated data over structural connectomes. The proposed approach is based on recursive filtering of the graph data and zero-crossing counting over the connectomes (graphs). The simplicity of the proposed method, namely simple Higher Order Crossings over graph sequence (
$sHOC_{g}$
), and its robust mathematical grounds, allow to discriminate subjects based on their structural wiring with high accuracy and dramatically faster estimation times (over 200 times faster) compared to state of the art graph kernel approaches.
更多查看译文
关键词
graph filtering,zero crossings,large scale connectome data set,graph classification
AI 理解论文
溯源树
样例
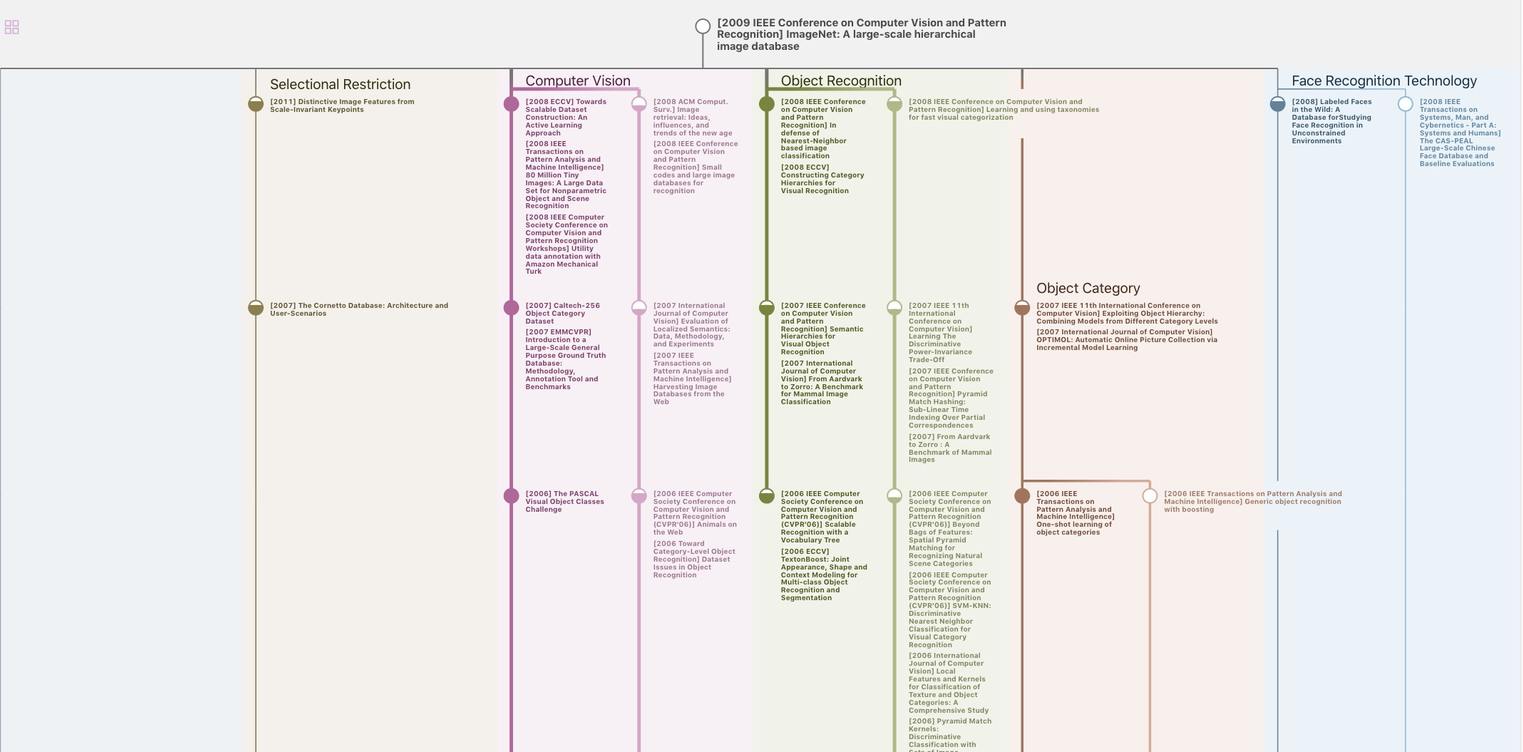
生成溯源树,研究论文发展脉络
Chat Paper
正在生成论文摘要