Calibrated Conversion Rate Prediction via Knowledge Distillation under Delayed Feedback in Online Advertising.
International Conference on Information and Knowledge Management (CIKM)(2022)
摘要
Prevailing calibration methods may fail to generalize well due to the pervasively delayed feedback issue in online advertising. That is, the labels of recent samples are more likely to be inaccurate because of the delayed feedback by users, while the old samples with complete feedback may suffer from the data shift compared to the recent ones. In this paper, we propose to calibrate conversion rate prediction models considering delayed feedback via the knowledge distillation technique. Specifically, we deploy a teacher model modeling by the samples with complete feedback to learn long-term conversion patterns and a student model modeling by the recent data to reduce the impact of data shift. We also devise a distillation loss to buoy the student model to learn from the teacher. Experimental results on two real-world advertising conversion rate prediction datasets demonstrate that our method can provide more calibrated predictions compared with the existing ones. We also exhibit that our method can be extended to different base models.
更多查看译文
关键词
knowledge distillation,advertising,conversion,delayed feedback,prediction
AI 理解论文
溯源树
样例
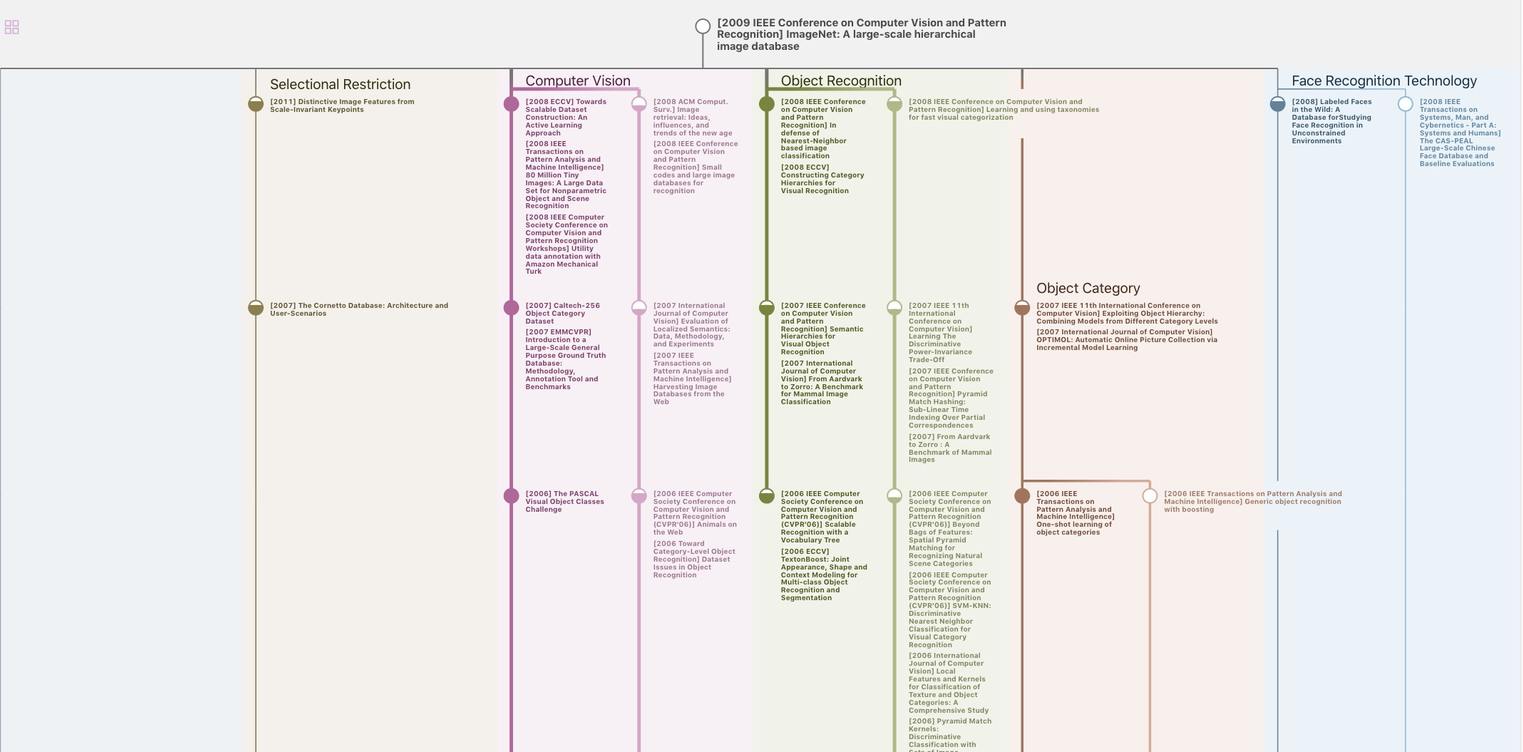
生成溯源树,研究论文发展脉络
Chat Paper
正在生成论文摘要