Fluorescence Microscopy Images Segmentation Based on Prototypical Networks with a Few Annotations.
Chinese Conference on Pattern Recognition and Computer Vision (PRCV)(2022)
摘要
The objects in fluorescence microscopy images (FMIs) are rather densely populated, and often have complex structures as well as large color variations. These issues result in time-consuming manual annotations for training a segmentation model. Few-shot segmentation (FSS) models reduce the requirements of annotations by using prototypical networks to generalize the object regions given only a few annotation masks. These methods, however, often fail to generalize to FMIs due to the domain shift from the images of source class to those of target class. To tackle these challenges, we adapt the prototypical networks from the few-shot learning scheme as our base model architecture to learn a compact feature representation of the object, with only a few annotated masks. Then, to solve the domain shift problem, we propose a resampling strategy to regularize the model training. Specifically, we construct the training set using not only the images form the source class, but also the support set from the target class by resampling the latter for regularizing the training. To distinguish our FMIs segmentation method from the conventional FSS models, we define it as a t raining r egularization strategy based on the p rototypical n etworks (TRPN). Experimental results on three public datasets, i.e. the ER, MITO and NUC, have confirmed the advantages of our method, which provides robust and accurate segmentation performance on FMIs only with a few annotation masks. Source code and data of this work are made publicly available at: https://github.com/cbmi-group/TRPN-model.
更多查看译文
关键词
fluorescence microscopy images segmentation,prototypical networks,few annotations
AI 理解论文
溯源树
样例
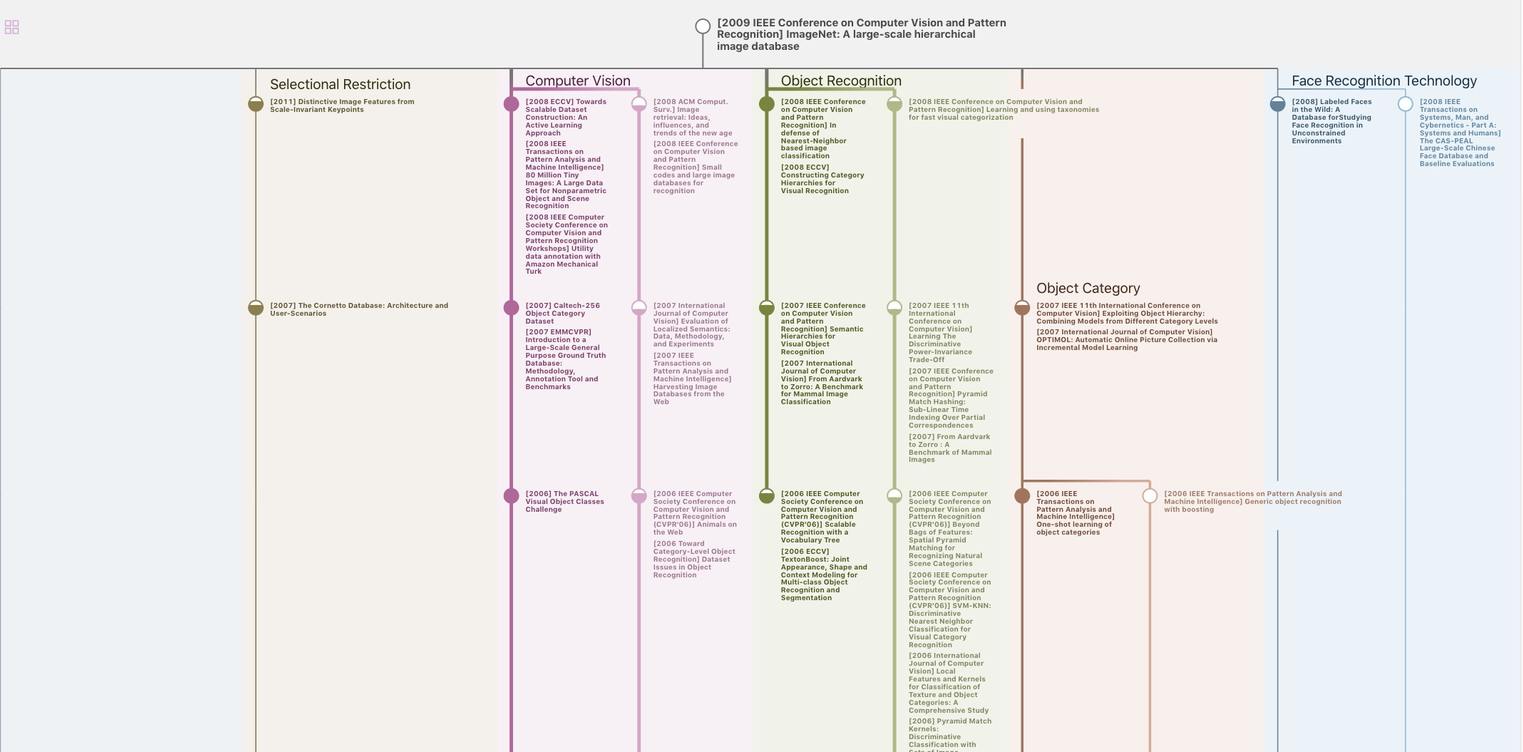
生成溯源树,研究论文发展脉络
Chat Paper
正在生成论文摘要